Fast Detection of Moving Traffic Participants in LiDAR Point Clouds by using Particles augmented with Free Space Information
2022 IEEE/RSJ International Conference on Intelligent Robots and Systems (IROS)(2022)
摘要
To navigate safely, it is essential for a robot to detect all kinds of moving objects that could possibly interfere with the own trajectory. For common object classes, like cars, regular pedestrians, and trucks, there are large scale datasets as well as corresponding machine learning techniques, which provide remarkable results in commonly available detection benchmarks. A big challenge that remains, are less frequent classes, which are not part of a dataset in a sufficient number and variation. Dynamic occupancy grids are a promising approach for detection of moving objects in point clouds since they impose only a few assumptions about the objects' appearance and shape. Typically, they use particle filters to detect motion of occupancy in the grid. Existing approaches, however, often generate false positives at long obstacles because particles move along them. Therefore we propose a highly efficient approach, which performs the classification in a more structured and conservative way by making extensive use of available free space information. As a result, much less false positives are generated while the number of false negatives remains low. Our approach can be used to complement CNN-based object detections in order to detect both, frequent and uncommon object classes reliably. By using polar data structures that match the polar measurement principle, we are able to process even large point clouds of modern LiDARs with 128 lasers efficiently.
更多查看译文
AI 理解论文
溯源树
样例
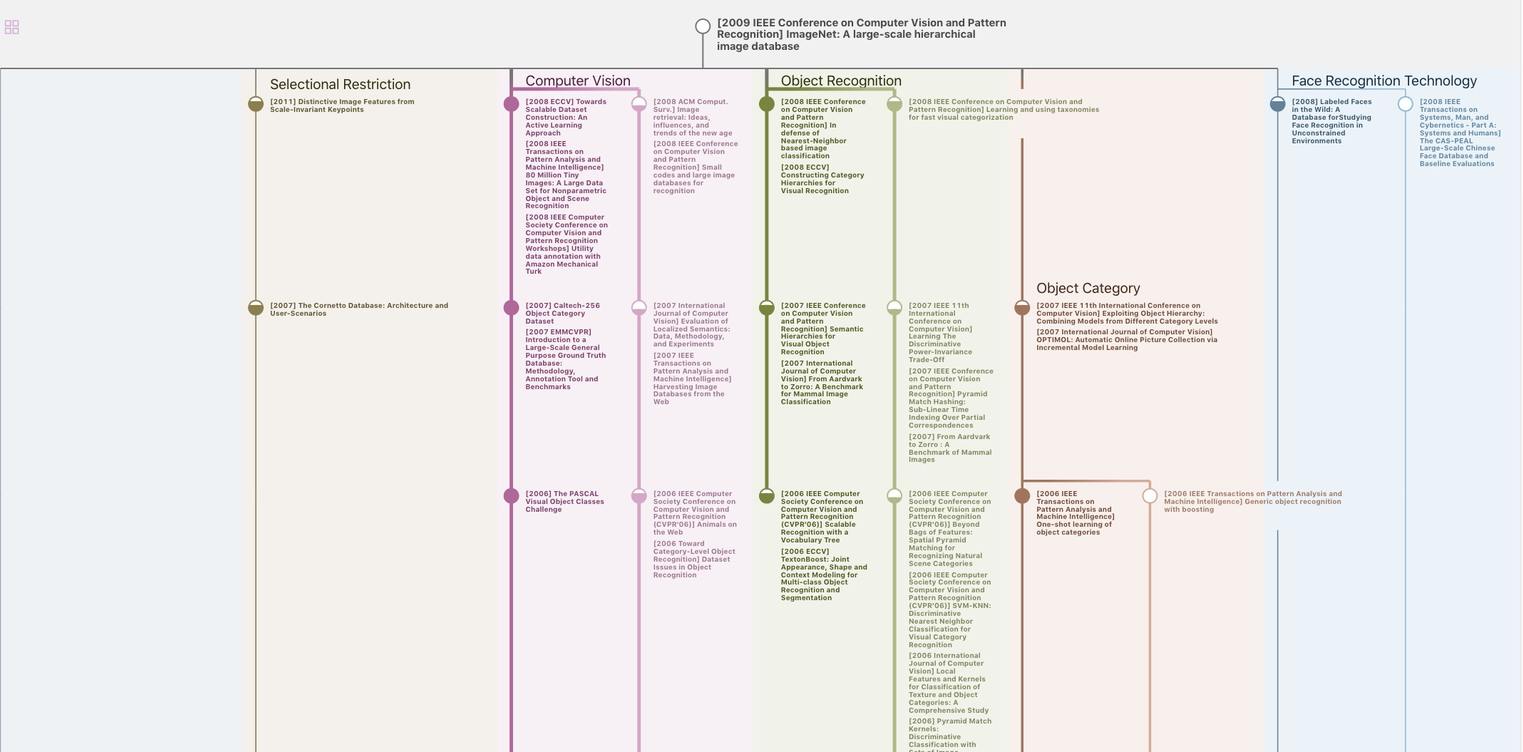
生成溯源树,研究论文发展脉络
Chat Paper
正在生成论文摘要