Smart detection of indoor occupant thermal state via infrared thermography, computer vision, and machine learning
Building and Environment(2023)
摘要
The ability to measure occupants’ thermal state in real time will enable major advances in the control of air conditioning systems. This study proposes predicting occupant thermal state by a combination of infrared thermography, computer vision, and machine learning. The approach (1) uses cheek, nose, and hand temperatures because they are least subject to blockage by hair, glasses, and clothing; (2) measures the distribution of skin temperatures within geometrically defined sub-areas of the face and hand; and (3) uses temperature differences within and between these areas to eliminate the effects of calibration drift that are unavoidable in thermal infrared (TIR) cameras. Two series of tests were conducted, respectively in an outdoor carport and an indoor environmental chamber, collecting a total of 48,422 sets of cheek, nose, and hand skin temperatures using a TIR camera and computer-vision technology, coupled with 715 subjective responses of thermal sensations. To predict occupant thermal state, Random Forest classification models were built using either absolute skin temperatures (the maximum and median temperatures of cheek and hand segments, and the temperature of the central spot on the nose), or intra- and inter-segment temperature differences of cheeks, hands, and nose. These measurements were found to accurately predict occupant thermal state. Using the maximum and median temperatures for cheek and nose, or for cheek and hand, predicts thermal state with an accuracy of 92–96%. Using only the intra- and inter-segment temperature differences from cheek and nose is 83% accurate; adding the hand temperature differences increases the accuracy to 96%.
更多查看译文
关键词
Infrared thermography,Computer vision,Machine learning,Thermal comfort,Prediction model
AI 理解论文
溯源树
样例
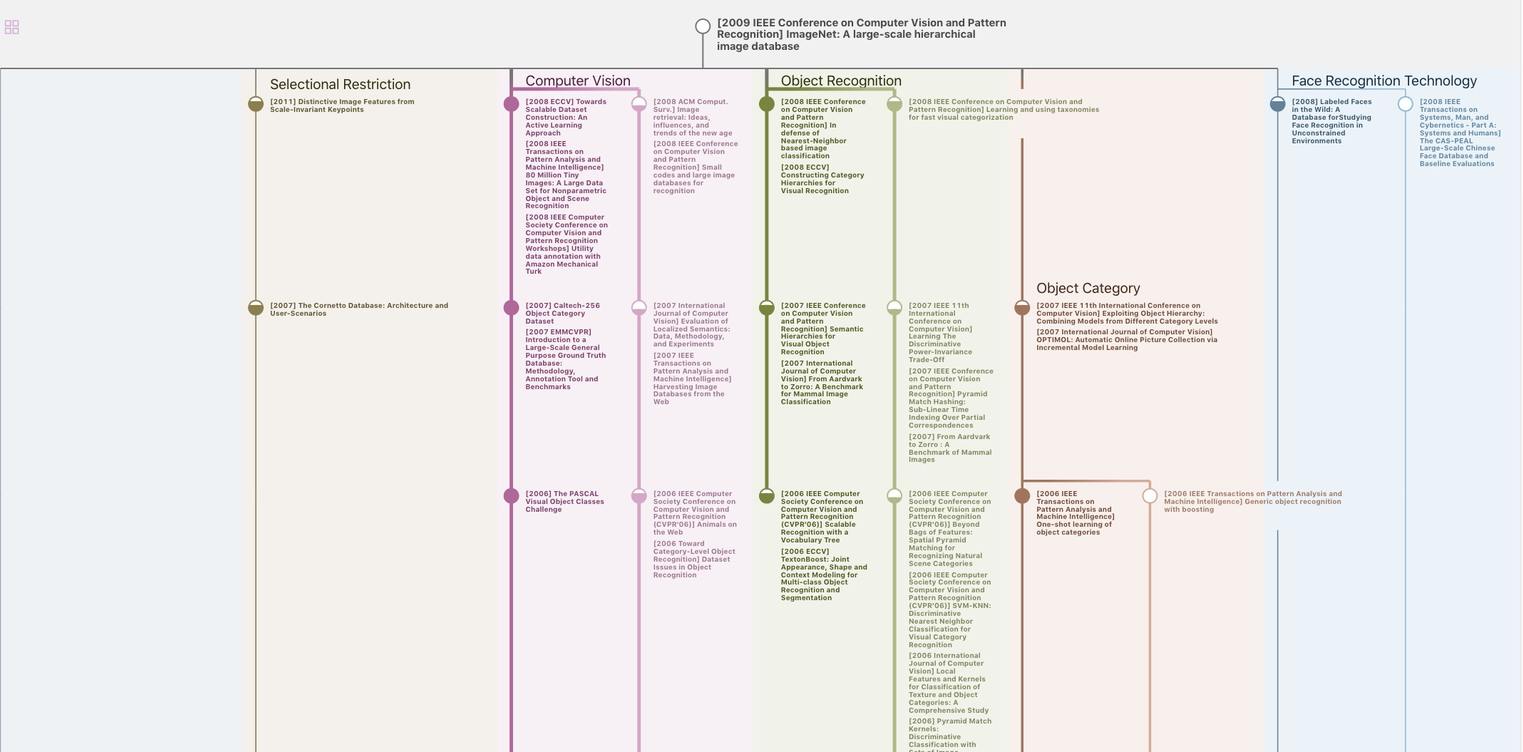
生成溯源树,研究论文发展脉络
Chat Paper
正在生成论文摘要