Machine Learning Reveals Lipidome Remodeling Dynamics in a Mouse Model of Ovarian Cancer
biorxiv(2023)
摘要
Ovarian cancer (OC) is one of the deadliest cancers affecting the female reproductive system. It may present little or no symptoms at the early stages, and typically unspecific symptoms at later stages. High-grade serous ovarian cancer (HGSC) is the subtype responsible for most ovarian cancer deaths. However, very little is known about the metabolic course of this disease, particularly in its early stages. In this longitudinal study, we examined the temporal course of serum lipidome changes using a robust HGSC mouse model and machine learning data analysis. Early progression of HGSC was marked by increased levels of phosphatidylcholines and phosphatidylethanolamines. In contrast, later stages featured more diverse lipids alterations, including fatty acids and their derivatives, triglycerides, ceramides, hexosylceramides, sphingomyelins, lysophosphatidylcholines, and phosphatidylinositols. These alterations underscored unique perturbations in cell membrane stability, proliferation, and survival during cancer development and progression, offering potential targets for early detection and prognosis of human ovarian cancer.
### Competing Interest Statement
The authors have declared no competing interest.
更多查看译文
关键词
lipidome remodeling dynamics,ovarian cancer,machine learning,mouse remodeling
AI 理解论文
溯源树
样例
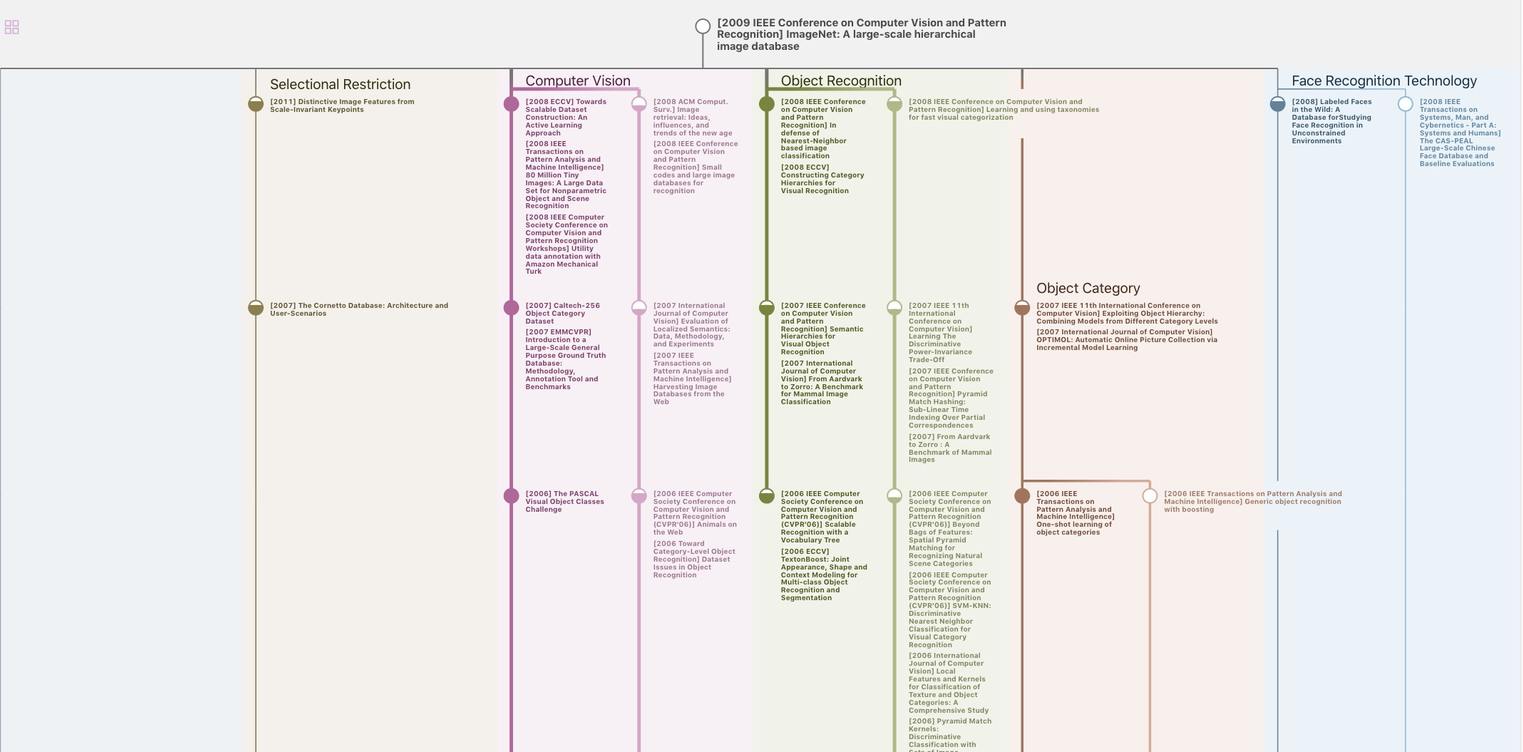
生成溯源树,研究论文发展脉络
Chat Paper
正在生成论文摘要