Enhancer-driven gene regulatory networks inference from single-cell RNA-seq and ATAC-seq data
biorxiv(2022)
摘要
We present an algorithm, STREAM, for enhancer-driven gene regulatory network (eGRN) inference from transcriptome and chromatin accessibility profiled in the same cells. The algorithm improves the prediction accuracy of relations among transcription factors (TFs), enhancers, and genes by two new ideas: (i) a Steiner forest problem model to identify the set of highly confident enhancer-gene relations underlying a context-specific functional gene module; and (ii) a hybrid biclustering pipeline integrated with submodular optimization for inferring eGRNs by identifying the optimal set of hybrid biclusters, each of which represents co-regulated genes by the same TF and co-accessible enhancers bound by the same TF over a cell subpopulation. These two ideas are embedded in an iterative framework by finding patterns in a pair of transcriptome and chromatin accessibility matrices. Benchmarking analysis shows that the performance, assessed by f -scores, precision, or recall, was significantly improved by our program compared to four state-of-the-art tools on nine datasets. Besides, by implementing STREAM on an Alzheimer’s disease dataset, we identified TF-enhancer-gene relations associated with pseudotime and investigated the changing of enhancer-gene relations alongside cell lineages. Additionally, by implementing STREAM on a diffuse small lymphocytic lymphoma dataset, we excavated key TF-enhancer-gene relations and TF cooperation underlying tumor cells.
### Competing Interest Statement
The authors have declared no competing interest.
更多查看译文
AI 理解论文
溯源树
样例
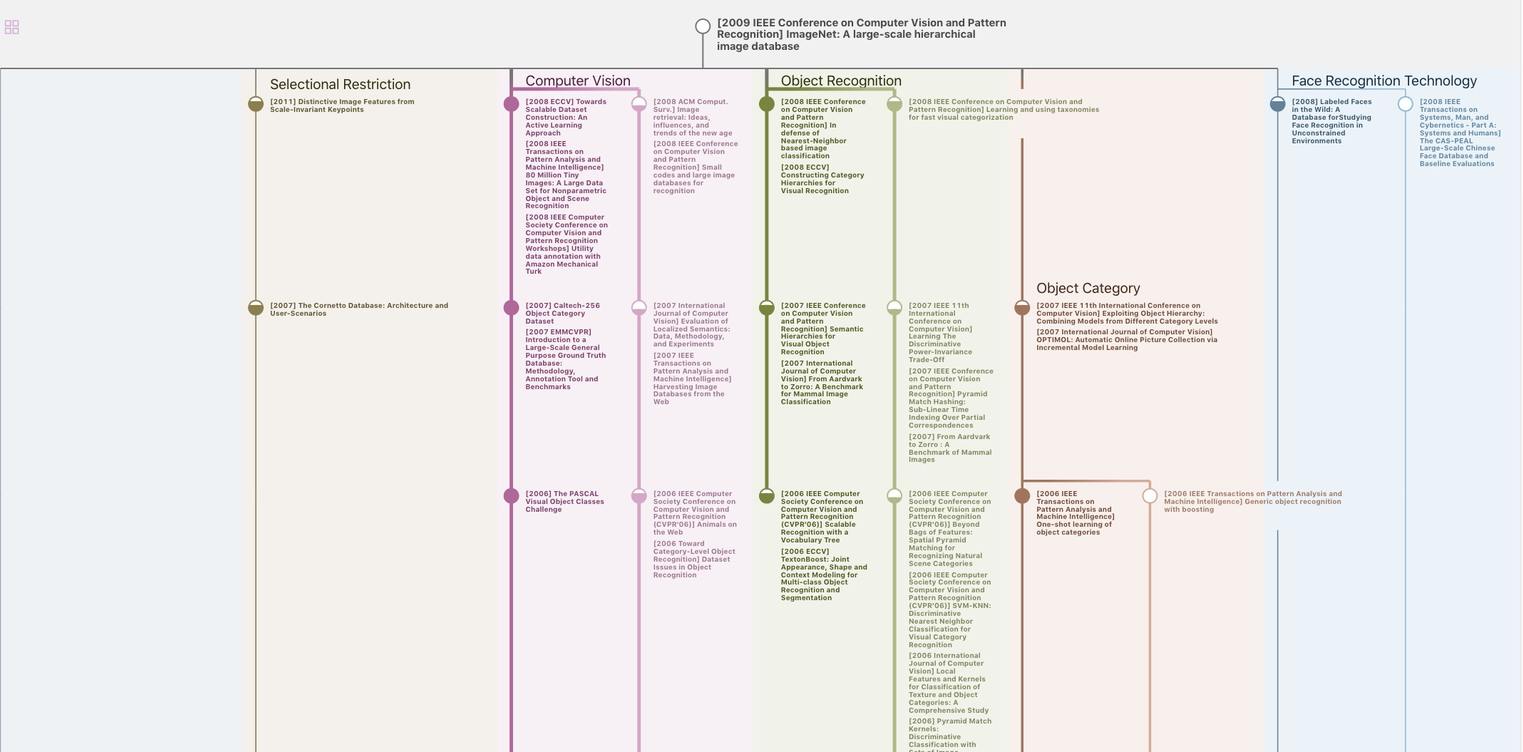
生成溯源树,研究论文发展脉络
Chat Paper
正在生成论文摘要