Bayesian nonparametric general regression with adaptive kernel bandwidth and its application to seismic attenuation
Advanced Engineering Informatics(2023)
摘要
General Regression Neural Network (GRNN) possesses distinct function approximation capability and predictive power without the requirement of a prescribed functional form. However, its prediction accuracy relies on uniformly distributed input training data. If the input training data are non-uniformly distributed, considerable bias will occur. It is especially pronounced when the data points are sparsely distributed. Moreover, GRNN presumes a set of input variables to be included in the regression model so it remains an issue to determine the proper set of input variables. To address these issues, we propose the Bayesian Nonparametric General Regression with Adaptive Kernel Bandwidth (BNGR-AKB). First, it determines the bandwidth of the kernels adaptively so as to accommodate non-uniformly distributed input training data. Furthermore, it utilizes Bayesian inference to determine the input variables to be included in the regression model. To demonstrate the variable selection and regression capacity of the proposed method for non-uniformly distributed input training data, we present three simulated examples and one real data example using the ground motion records of Wenchuan earthquake.
更多查看译文
关键词
Adaptive bandwidth,General regression,Model class selection,Variable selection,Sparse data,Seismic attenuation
AI 理解论文
溯源树
样例
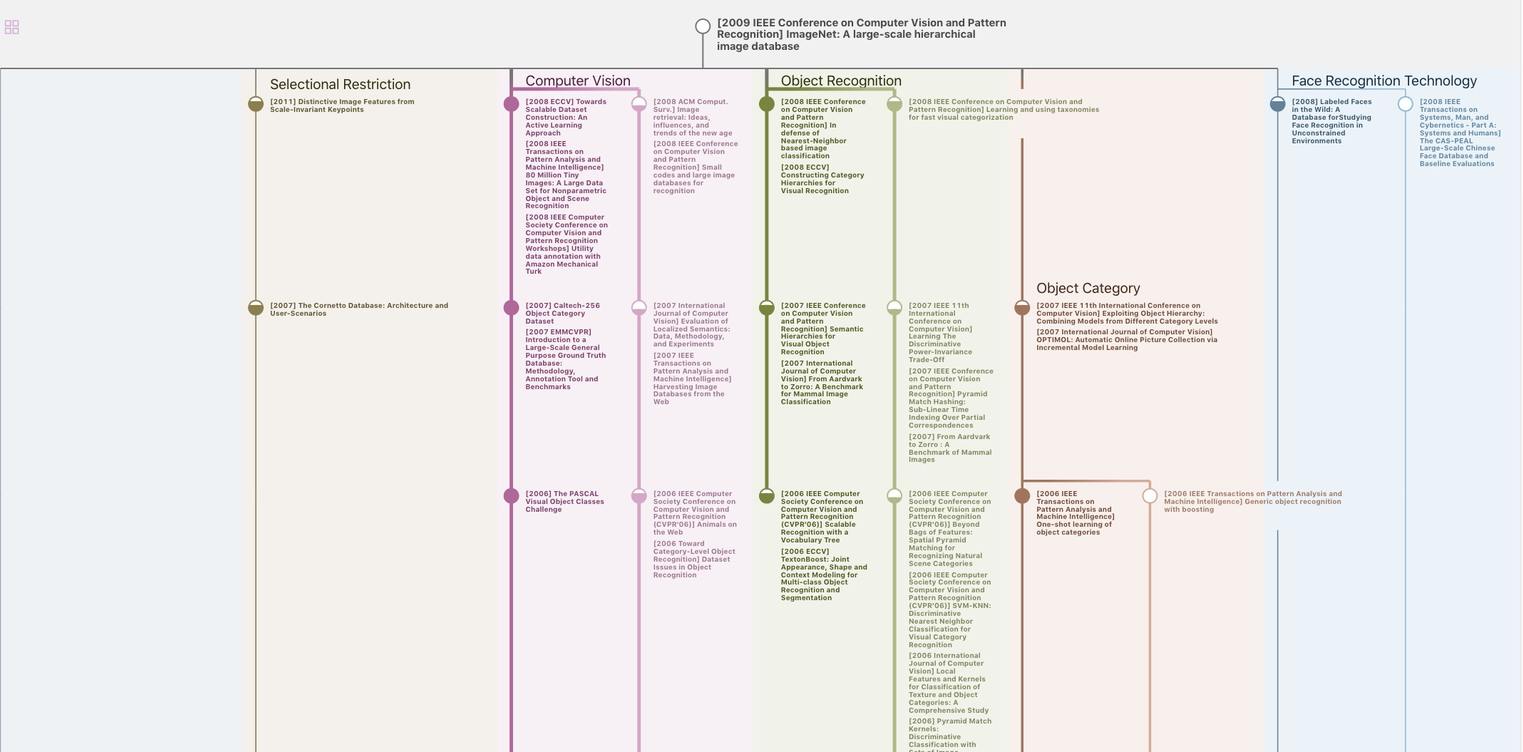
生成溯源树,研究论文发展脉络
Chat Paper
正在生成论文摘要