Siamese conditional generative adversarial network for multi-focus image fusion
Applied Intelligence(2023)
摘要
Multi-focus image fusion (MFIF) combines information by utilizing various image sequences of the same scenes at different of focus depths. The available MFIF method based on generative adversarial networks (GAN) lacks the feature complementarity of multi-focus images, resulting in the loss of details and noises in the generated decision maps. To resolve this problem, the learning framework of a joint distribution was developed via Siamese conditional generative adversarial network (SCGAN). This framework utilizes Siamese conditional generator that produces two-probabilistic feature maps from multi-focus images with complementary information. Additionally, the proposed framework also considers both the diversity of datasets and network convergence. The structural sparse objective function is designed to penalize the prediction of low confidence by sparse calculation of the rows and columns of the matrix. So, it endows a better Dice coefficient with higher values and improves the generalization capability of the GAN. Also, Wasserstein Divergence (DIV) is utilized to optimize the discrimination performance with stable training. In both quantitative and qualitative experiments, SCGAN has better scores on MFIF than other methods.
更多查看译文
关键词
Siamese conditional generative adversarial network,Structural sparse loss,Multi-focus image fusion,Deep learning
AI 理解论文
溯源树
样例
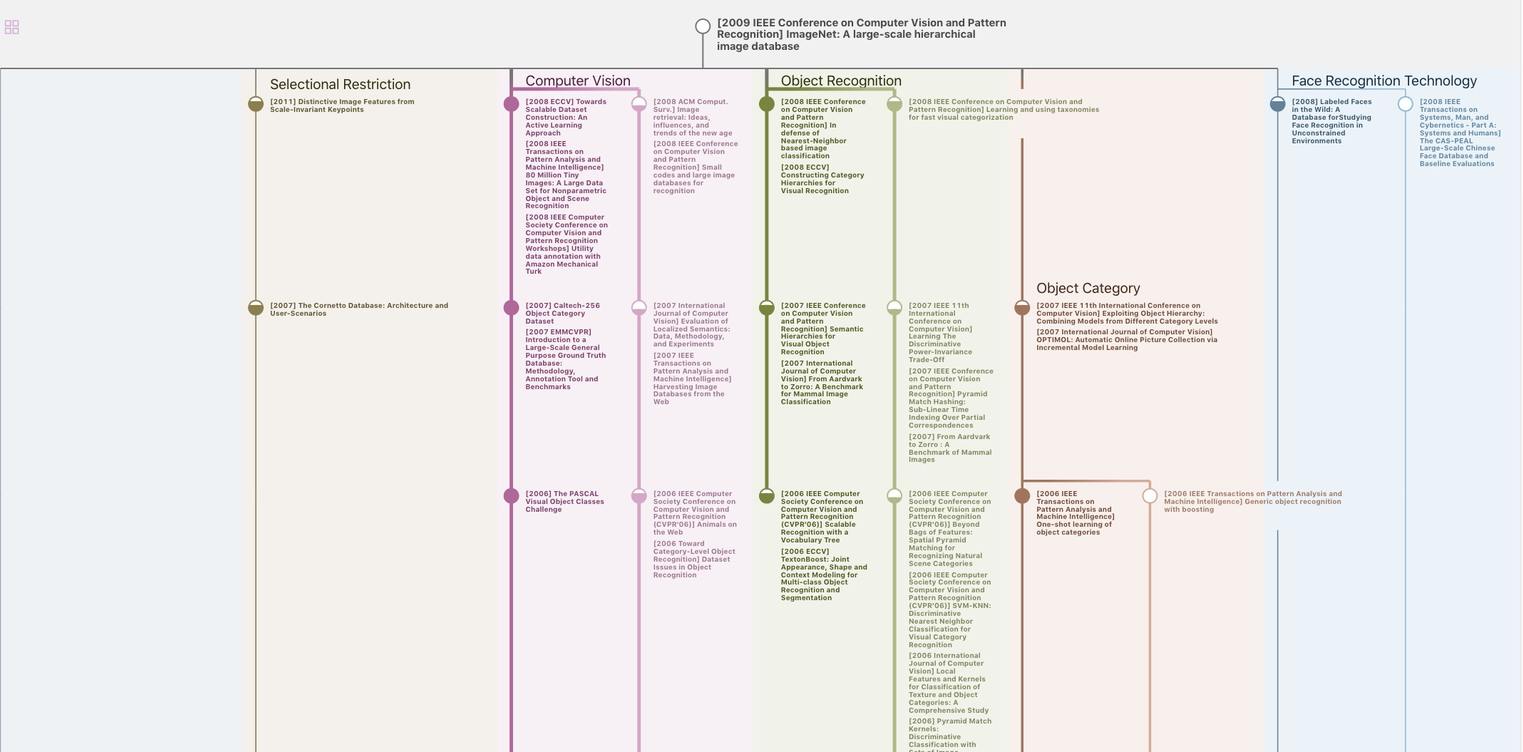
生成溯源树,研究论文发展脉络
Chat Paper
正在生成论文摘要