Prediction of energy consumption in campus buildings using long short-term memory
Alexandria Engineering Journal(2023)
摘要
In this paper, Long Short-Term Memory (LSTM) was proposed to predict the energy consumption of an institutional building. A novel energy usage prediction method was demon-strated for daily day-ahead energy consumption by using forecasted weather data. It used weather forecasting data from a local meteorological organization, the Malaysian Meteorological Depart-ment (MET). The predictive model was trained by considering the dependencies between energy usage and weather data. The performance of the model was compared with Support Vector Regres-sion (SVR) and Gaussian Process Regression (GPR). The experimental results with a dataset obtained from a building in Multimedia University, Malacca Campus from January 2018 to July 2021 outperformed the SVR and GPR. The proposed model achieved the best RMSE scores (561.692-592.319) when compared to SVR (3135.590-3472.765) and GPR (1243.307-1334.919). Through experimentation and research, the dropout method reduced overfitting significantly. Fur-thermore, feature analysis was done with SHapley Additive exPlanation to identify the most impor-tant weather variables. The results showed that temperature, wind speed, rainfall duration and the amount had a positive effect on the model. Thus, the proposed approach could aid in the implemen-tation of energy policies because accurate predictions of energy consumption could serve as system fault detection and diagnosis for buildings.(c) 2022 THE AUTHORS. Published by Elsevier BV on behalf of Faculty of Engineering, Alexandria University. This is an open access article under the CC BY license (http://creativecommons.org/licenses/by/ 4.0/).
更多查看译文
关键词
Energy consumption,Long short-term memory,Support vector regression,Gaussian process regression,Weather forecasting
AI 理解论文
溯源树
样例
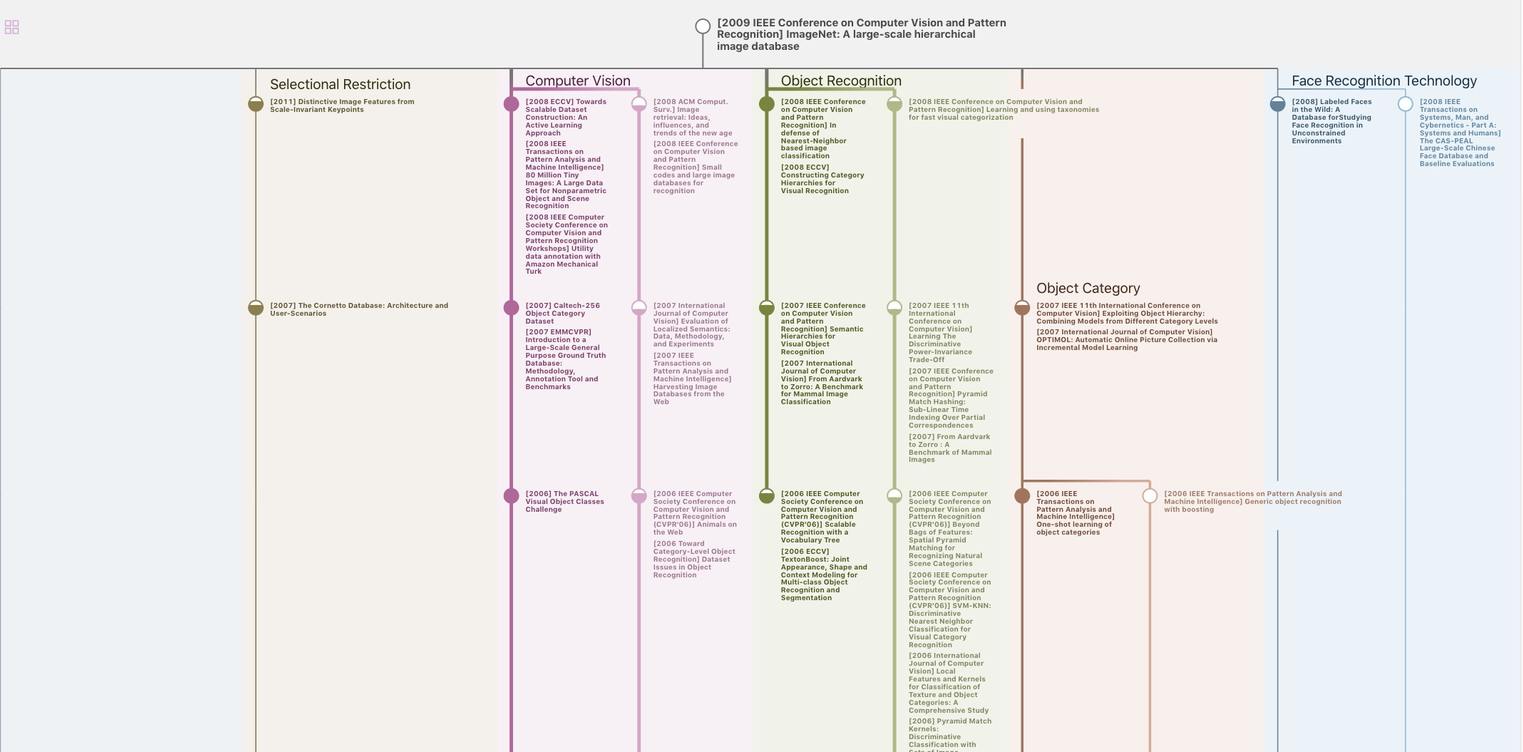
生成溯源树,研究论文发展脉络
Chat Paper
正在生成论文摘要