Joint few-shot registration and segmentation self-training of 3D medical images
Biomedical Signal Processing and Control(2023)
摘要
Medical image segmentation and registration are very important related steps in clinical medical diagnosis. In the past few years, deep learning techniques for joint segmentation and registration have achieved good results in both segmentation and registration tasks through one-way assisted learning or mutual utilization. However, they often rely on large labeled datasets for supervised training or directly use pseudo-labels without quality estimation. We propose a joint registration and segmentation self-training framework (JRSS), which aims to use segmentation pseudo-labels to promote shared learning between segmentation and registration in scenarios with few manually labeled samples while improving the performance of dual tasks. JRSS combines weakly supervised registration and semi-supervised segmentation learning in a self-training framework. Segmentation self-training generates high-quality pseudo-labels for unlabeled data by injecting noise, pseudo-labels screening, and uncertainty correction. Registration utilizes pseudo-labels to facilitate weakly supervised learning, and as input noise as well as data augmentation to facilitate segmentation self-training. Experiments on two public 3D medical image datasets, abdominal CT and brain MRI, demonstrate that our proposed method achieves simultaneous improvements in segmentation and registration accuracy under few-shot scenarios. Outperforms the single-task fully-supervised training state-of-the-art model in the metrics of Dice similarity coefficient and standard deviation of the Jacobian determinant.
更多查看译文
关键词
Image registration,Image segmentation,Few-shot,Self-training,Pseudo-labels
AI 理解论文
溯源树
样例
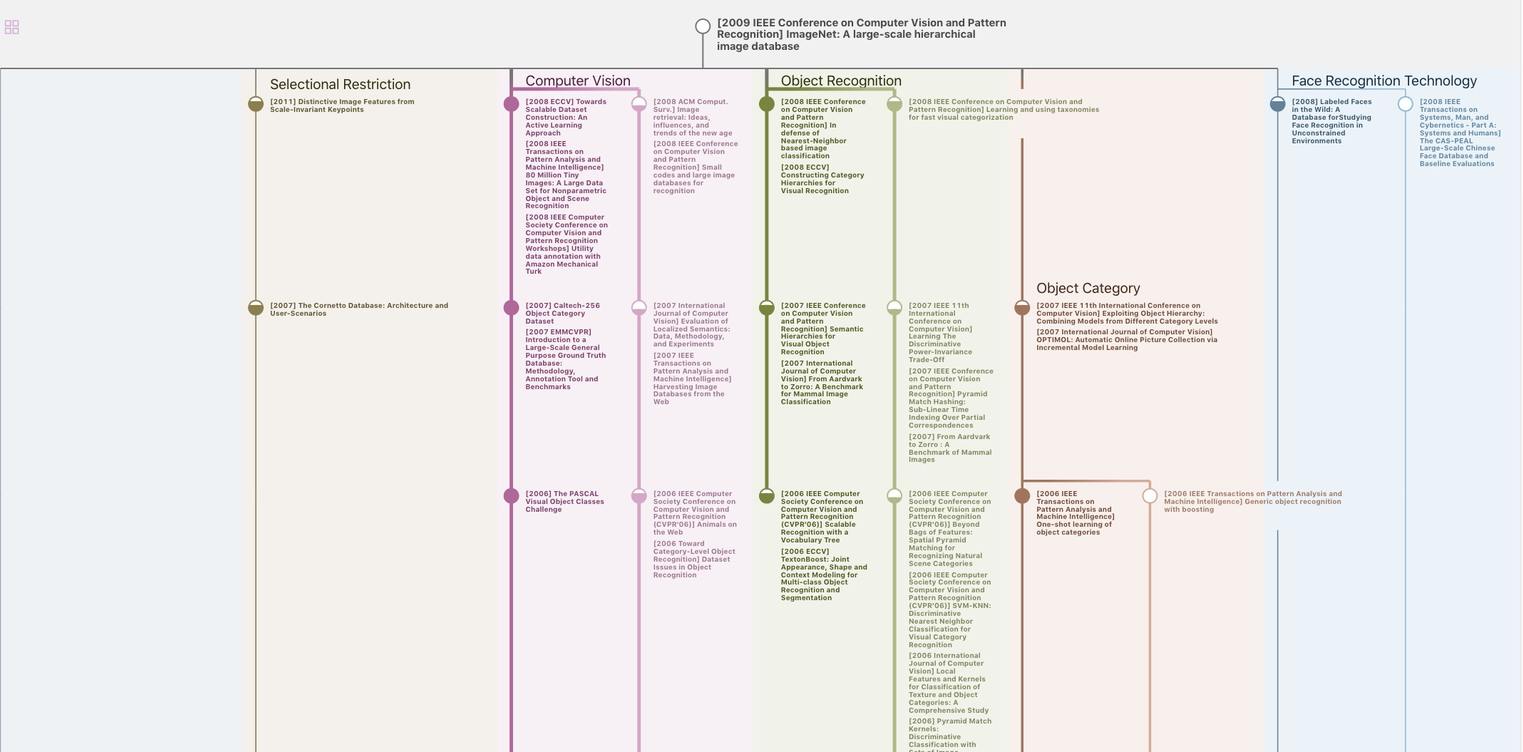
生成溯源树,研究论文发展脉络
Chat Paper
正在生成论文摘要