Automatic segmentation of ultrasound images using SegNet and local Nakagami distribution fitting model
Biomedical Signal Processing and Control(2023)
摘要
Automatic and accurate segmentation of ultrasound (US) images plays a vital role in computer-aided diagnosis of many diseases. However, multiple degradations within US images, such as speckle noise, intensity inhomogeneity, shadows, low contrast, and low signal-to-noise ratio, often cover up the details of imaging tissues and blur the edges of lesions, resulting in limited accuracy for existing segmentation algorithms. To tackle these issues, a coarse-to-fine segmentation method combining deep learning with an active contour model (ACM) is proposed in this paper. At the first stage, a SegNet is trained and employed to predict the segmentation mask because it not only has a superior boundary delineation capability, but also has high efficiency in terms of memory and computational time during inference. Due to the multiple degradations of US images, the unavailability of large-scale US image datasets, and the loss of spatial information during downsampling, the SegNet may only infer the coarse object boundaries, especially for the tissue with blurred edges. At the second stage, the segmentation mask of the SegNet is refined to locate the accurate object boundaries by a novel ACM termed the local Nakagami distribution fitting (LNDF) model, which takes advantage of discrepancies between Nakagami distributions of different tissues around the boundaries within US images. Experimental results on two US image datasets demonstrate that, compared with some state-of-the-art segmentation methods, our proposed method achieves the highest segmentation accuracy at the cost of acceptable running time, and thereby adapts to the automatic segmentation of US images in clinical applications.
更多查看译文
关键词
Image segmentation,SegNet,Active contour model,Nakagami distribution,Ultrasound image
AI 理解论文
溯源树
样例
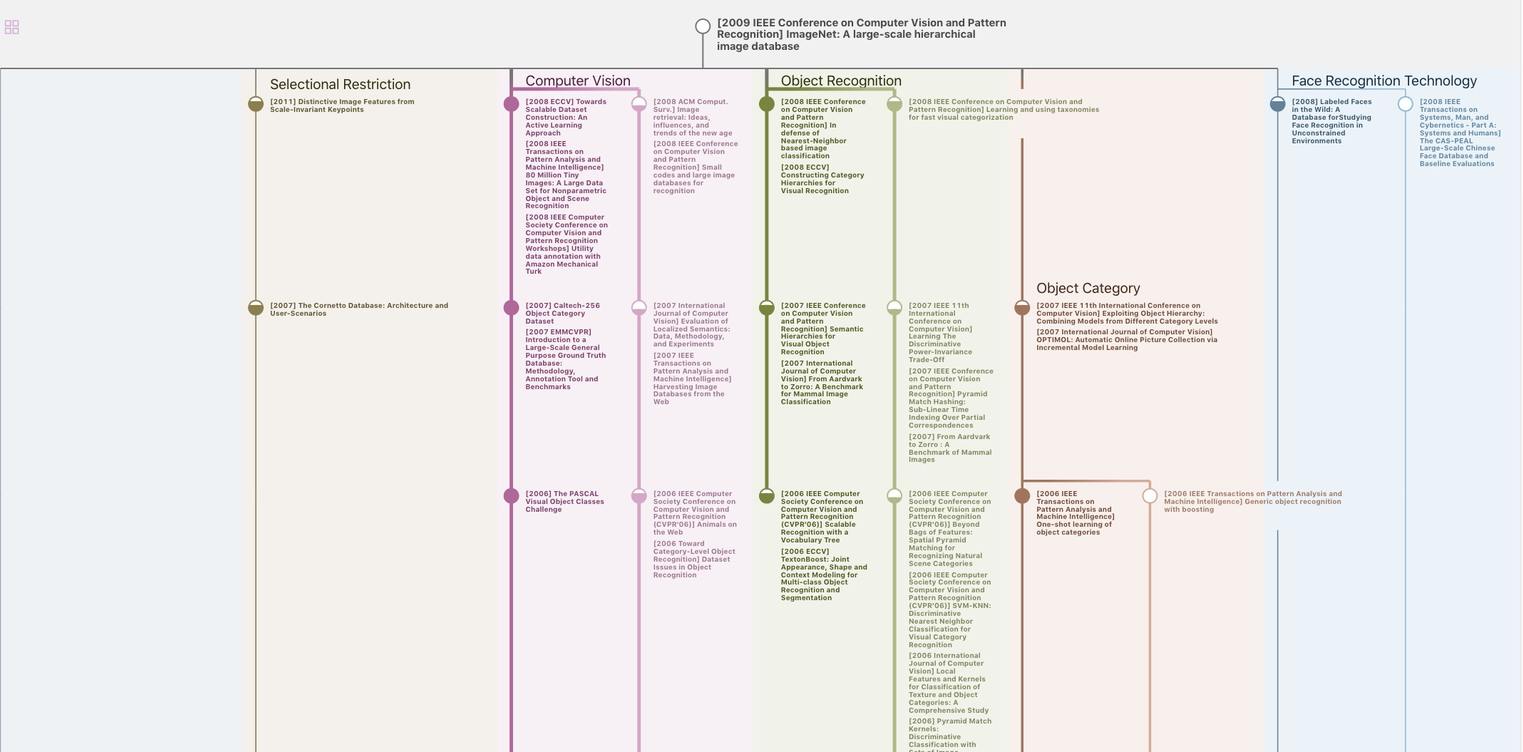
生成溯源树,研究论文发展脉络
Chat Paper
正在生成论文摘要