Modality-level Cross-Connection and Attentional Feature Fusion Based Deep Neural Network for Multi-Modal Brain Tumor Segmentation
Biomedical signal processing and control(2023)
摘要
Brain tumor segmentation from Magnetic Resonance Imaging is essential for early diagnosis and treatment planning for brain cancers in clinical practice. However, existing brain tumor segmentation methods cannot sufficiently learn high-quality feature information for segmentation. To address this issue, a modality-level cross-connection and attentional feature fusion based deep neural network is proposed for multi-modal brain tumor segmentation. The proposed method can not only locate the whole tumor region but also can accurately segment the sub-tumor regions. The proposed network architecture is a multi-encoder based 3D U-Net. Inspired by the characteristics of multi-modalities, a modality-level cross-connection (MCC) is first proposed to take advantage of the complementary information between the related modalities. Moreover, to enhance the feature learning capacity of the network, the attentional feature fusion module (AFFM) is proposed to fuse the multi-modalities as well as to extract the useful feature representation for segmentation. It consists of two components: multi-scale spatial feature fusion (MSFF) block and dual-path channel feature fusion (DCFF) block. They aim at learning multi-scale spatial contextual information and the channel-wise feature information to improve the segmentation accuracy. Also, the proposed fusion module can be easily integrated into other fusion models and deep neural network architectures. Comprehensive experiments evaluated on the BraTS 2018 dataset demonstrate that the proposed network architecture can effectively improve the brain tumor segmentation performance when compared with the baseline methods and the state-of-the-art methods.
更多查看译文
关键词
Brain tumor segmentation,Cross-connection,Feature fusion,Multi-modality,Deep learning
AI 理解论文
溯源树
样例
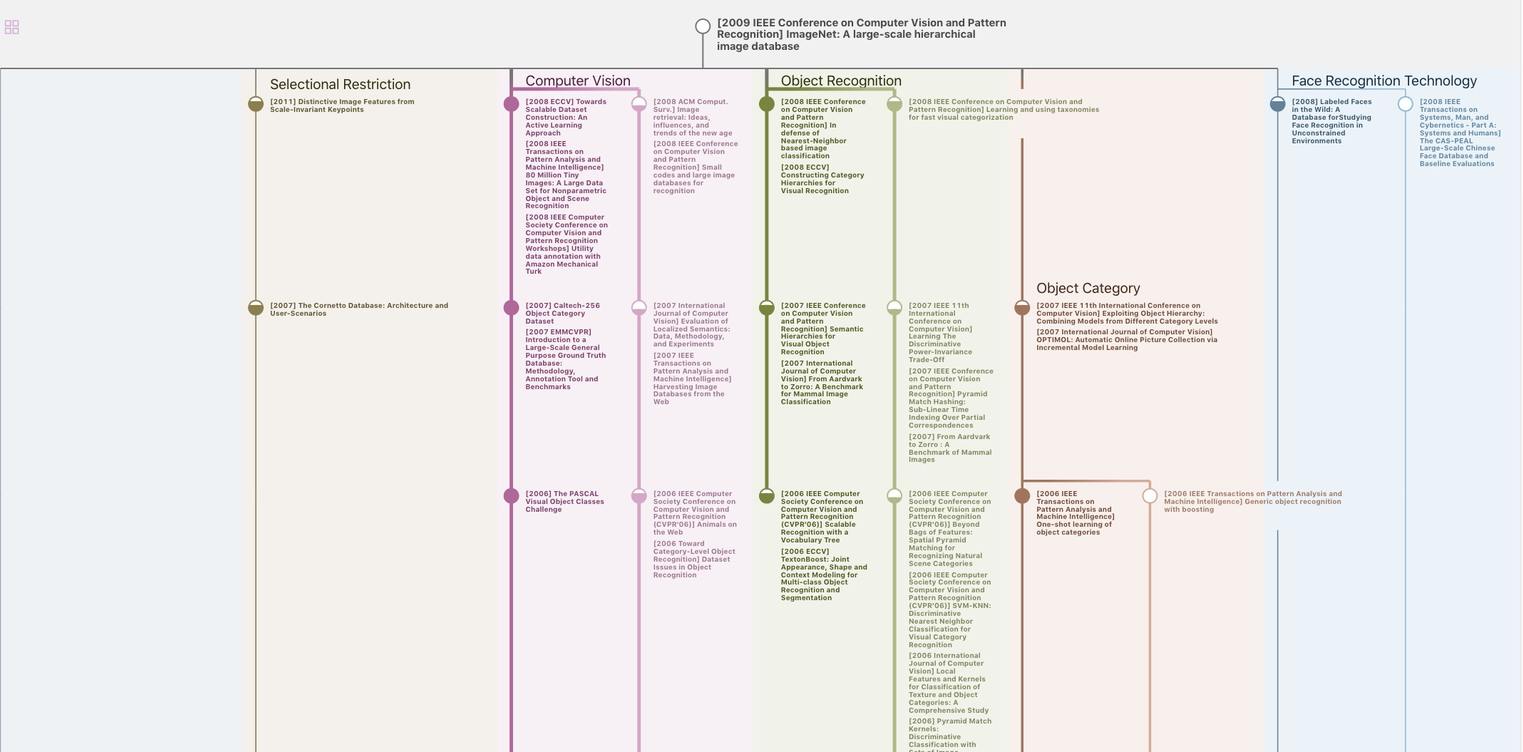
生成溯源树,研究论文发展脉络
Chat Paper
正在生成论文摘要