Domain-ensemble learning with cross-domain mixup for thoracic disease classification in unseen domains
Biomedical Signal Processing and Control(2023)
摘要
Chest radiography is the most common radiology examination for thoracic disease diagnosis, such as pneumonia. A tremendous number of chest X-rays prompt data-driven deep learning models in constructing computer-aided diagnosis (CAD) systems for thoracic disease diagnosis. However, in clinically realistic practice, a trained deep learning model often suffers from performance degradation when applied to unseen data, mainly due to domain shifts caused by various scanner vendors, imaging protocols, and patient demographics etc. To this end, we present a novel domain-ensemble learning with cross-domain mixup (DELCOM) method for thoracic disease diagnosis in unseen domains using chest X-rays. The core idea of our method is to learn multiple domain-specific models using data from both virtual and source domains and to generalize them to unseen domains. Specifically, we first introduce cross-domain mixup to construct virtual samples not belonging to any source domain by mixing up the pairs of samples from source domains. We then model the simulation process of domain shift through domain-ensemble learning. The results indicate that our method not only outperforms other state-of-the-art domain generalization (DG) methods on unseen datasets but also achieves comparable performance across all source domains.
更多查看译文
关键词
Thoracic diseases,Image classification,Deep learning,Domain generalization,Chest X-ray
AI 理解论文
溯源树
样例
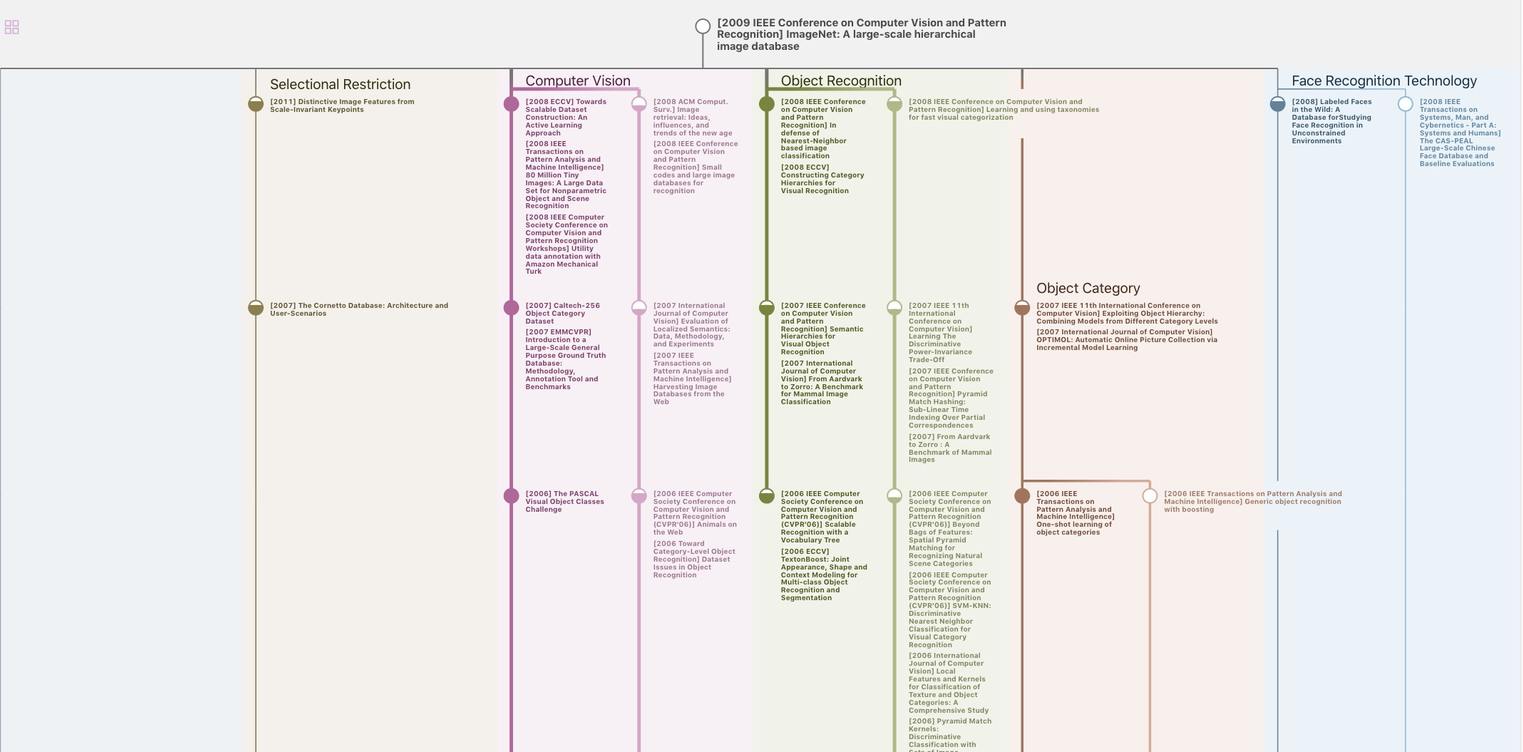
生成溯源树,研究论文发展脉络
Chat Paper
正在生成论文摘要