Diabetes Mellitus Risk Prediction Using Age Adaptation Models
Biomedical Signal Processing and Control(2023)
Abstract
Conventional disease prediction models frequently have imbalanced or insufficient observed samples. In a real-world situation, the learned model from an imbalanced dataset may have poor generalization ability. In this paper, we use machine learning methods to investigate age adaptation in Diabetes Mellitus (DM) risk prediction. Typical machine learning algorithms for DM prediction include Linear Regression (LR), Logistic Regression, Polynomial Regression (PR), Neural Network (NN), Support Vector Machines (SVM), Random Forest (RF), and XGboost (XGB). Based on feature compensation and soft decision threshold adjustment, we propose a novel age adaptation algorithm. The results were validated using the publicly available Pima Indian Diabetes Dataset. The experimental results show that the disease risk prediction model’s efficiency and accuracy are promising. The performance dropped for different age groups when using the original features. Using the compensated features, the accuracy rates for DM models were improved considerably. Age adaptation methods can be trained on one age group and adapted to another, solving the problem of data scarcity in a specific age range.
MoreTranslated text
Key words
Adaptive learning,Adaptive learning Disease prediction,Feature compensation,Diabetes mellitus
AI Read Science
Must-Reading Tree
Example
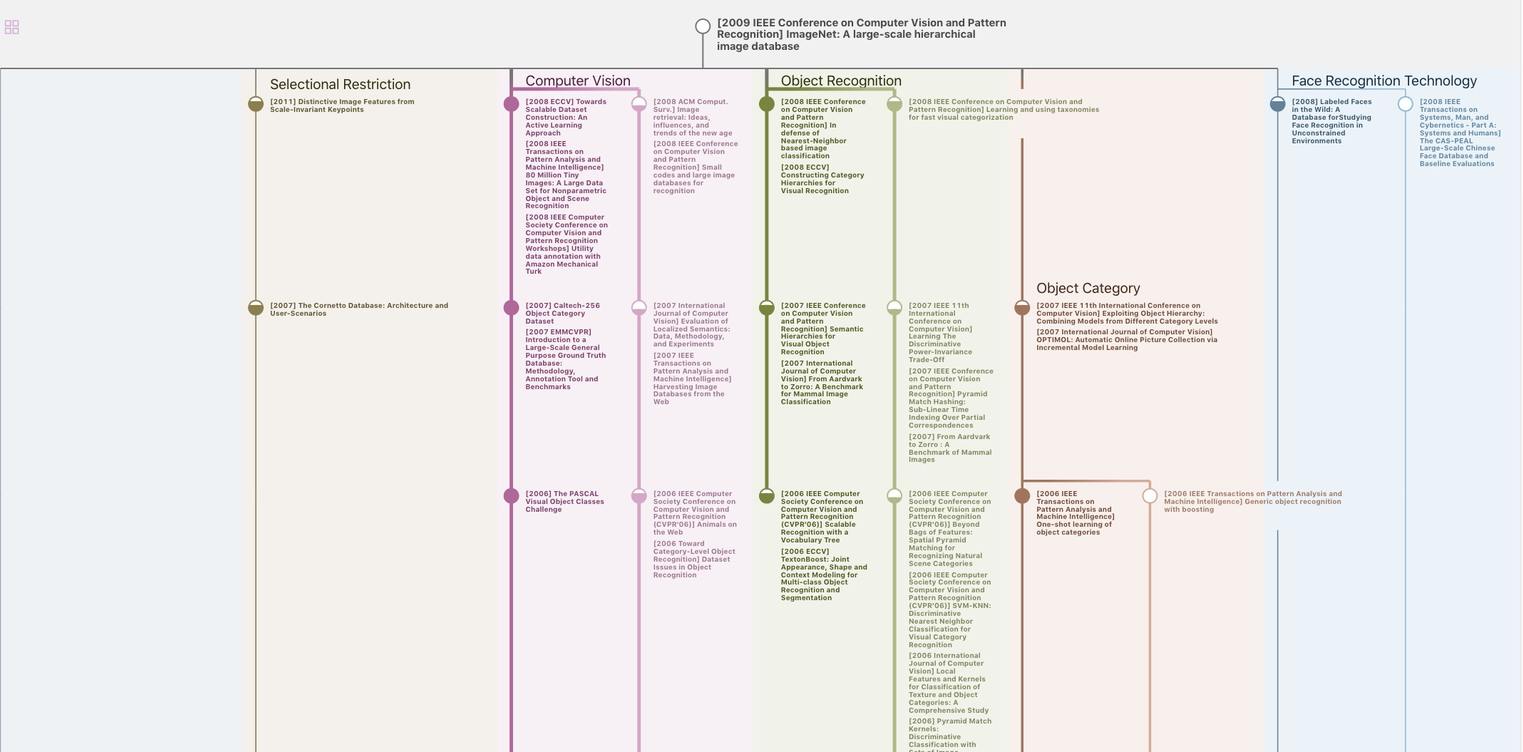
Generate MRT to find the research sequence of this paper
Chat Paper
Summary is being generated by the instructions you defined