Dynamic Ensemble Deep Echo State Network for Significant Wave Height Forecasting
Applied Energy(2023)SCI 1区
Abstract
Forecasts of the wave heights can assist in the data-driven control of wave energy systems. However, the dynamic properties and extreme fluctuations of the historical observations pose challenges to the construction of forecasting models. This paper proposes a novel dynamic ensemble deep Echo state networks (ESN) to learn the dynamic characteristics of the significant wave height. The dynamic ensemble ESN creates a profound representation of the input and trains an independent readout module for each reservoir. To begin, numerous reservoir layers are built in a hierarchical order, adopting a reservoir pruning approach to filter out the poorer representations. Finally, a dynamic ensemble block is used to integrate the forecasts of all readout layers. The suggested model has been tested on twelve available datasets and statistically outperforms state-of-the-art approaches.
MoreTranslated text
Key words
Forecasting,Machine learning,Deep learning,Randomized neural networks,Echo state network
求助PDF
上传PDF
View via Publisher
AI Read Science
AI Summary
AI Summary is the key point extracted automatically understanding the full text of the paper, including the background, methods, results, conclusions, icons and other key content, so that you can get the outline of the paper at a glance.
Example
Background
Key content
Introduction
Methods
Results
Related work
Fund
Key content
- Pretraining has recently greatly promoted the development of natural language processing (NLP)
- We show that M6 outperforms the baselines in multimodal downstream tasks, and the large M6 with 10 parameters can reach a better performance
- We propose a method called M6 that is able to process information of multiple modalities and perform both single-modal and cross-modal understanding and generation
- The model is scaled to large model with 10 billion parameters with sophisticated deployment, and the 10 -parameter M6-large is the largest pretrained model in Chinese
- Experimental results show that our proposed M6 outperforms the baseline in a number of downstream tasks concerning both single modality and multiple modalities We will continue the pretraining of extremely large models by increasing data to explore the limit of its performance
Upload PDF to Generate Summary
Must-Reading Tree
Example
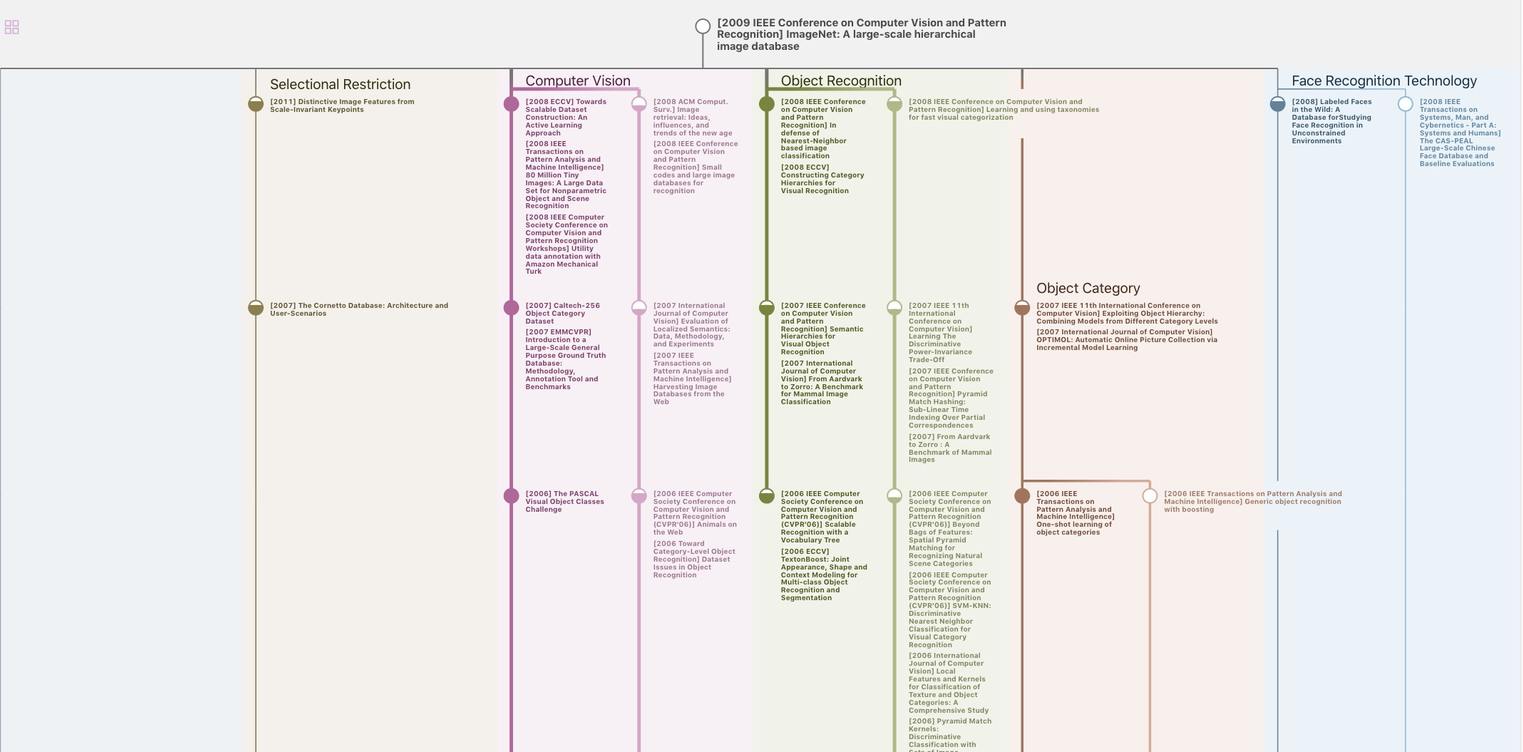
Generate MRT to find the research sequence of this paper
Related Papers
2008
被引用118 | 浏览
2012
被引用84 | 浏览
2016
被引用48 | 浏览
2020
被引用162 | 浏览
2020
被引用22 | 浏览
2020
被引用3 | 浏览
2020
被引用46 | 浏览
2021
被引用66 | 浏览
2021
被引用14 | 浏览
2021
被引用44 | 浏览
2021
被引用37 | 浏览
Data Disclaimer
The page data are from open Internet sources, cooperative publishers and automatic analysis results through AI technology. We do not make any commitments and guarantees for the validity, accuracy, correctness, reliability, completeness and timeliness of the page data. If you have any questions, please contact us by email: report@aminer.cn
Chat Paper