Color assignment optimization for categorical data visualization with adjacent blocks
Journal of Visualization(2023)
摘要
Categorical data charts composed of adjacent color blocks, such as pie charts and stack bar charts, are widely used in daily life and scientific research. Color is a significant channel in such charts. In general, colors are used to distinguish the categories of these data. In some scenes of low color accuracy, the indistinguishability of colors will make these charts challenging to read. Similar colors and poor viewing conditions make it difficult for people to read charts, and people spend more time distinguishing the boundaries of color blocks. There have been previous works by modifying the palette to achieve the distinction between colors. However, in many scenarios, people need to use a palette that meets a particular style and does not allow modification. There is also a method to optimize the color assignment with a given palette, but the method is only designed for scatter plots. In this paper, we propose an automatic coloring method for optimizing the distinguishability of blocks with a given palette based on graph theory and color science. We consider the adjacency of blocks in visual charts as a graph structure and take into account the color difference, block size, and color harmony. To demonstrate the method’s effectiveness, we compared our results with those of another color assignment method. We also use a class visibility measurement method and an aesthetic evaluation method based on deep learning to evaluate each method’s results. The results show that our method can guarantee the distinguishability of the color blocks and produce a sufficiently harmonious visualization. Graphical abstract
更多查看译文
关键词
Color assignment, Color difference, Color harmony, Data visualization, Graph matching
AI 理解论文
溯源树
样例
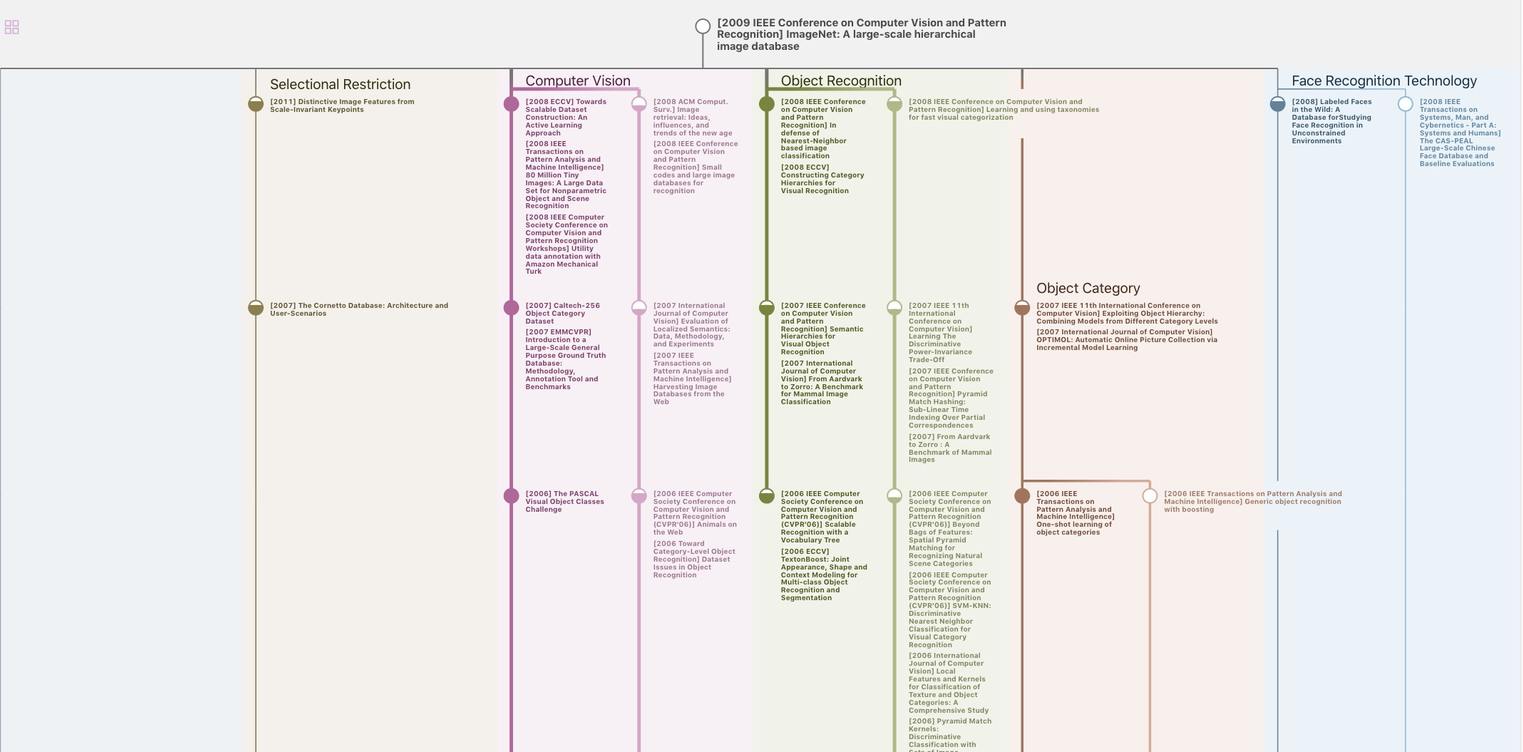
生成溯源树,研究论文发展脉络
Chat Paper
正在生成论文摘要