GMIX: Graph-based spatial–temporal multi-agent reinforcement learning for dynamic electric vehicle dispatching system
Transportation Research Part C: Emerging Technologies(2022)
摘要
The past decade has witnessed a significant growth of electric vehicles (EVs) deployment in public and private transportation sectors. Dynamic electric vehicle routing aims to plan the routes of EVs to serve dynamically generated customers’ requests while ensuring the battery level by visiting the recharge stations. This paper studies the dynamic electric vehicle routing problem with simultaneous pick-up and delivery and soft time windows (DEVRP-STW). We design a graph-based spatio-temporal multi-agent reinforcement learning (GMIX) framework consisting of a spatio-temporal graph attention network (ST-GAT) and a value decomposition-based multi-agent reinforcement learning algorithm (Graph-QMIX). Graph-QMIX derives a multi-agent soft policy gradient based on QMIX and Soft Actor–Critic and utilises a graph-based mixing network proposed to enhance the interaction of agents. Extensive experiments including a case study using real-world taxi data from New York City and a simulation study are conducted. The proposed GMIX outperforms the baseline algorithms on the request–response ratio (RRO), the average travelling distance per completed request (ATR), the out of energy ratio (OER) and the average waiting time per completed request (AWR).
更多查看译文
关键词
Transportation,Dynamic electric vehicle routing,Spatio-temporal graph attention network,Multi-agent soft policy gradient,Graph-based mixing network
AI 理解论文
溯源树
样例
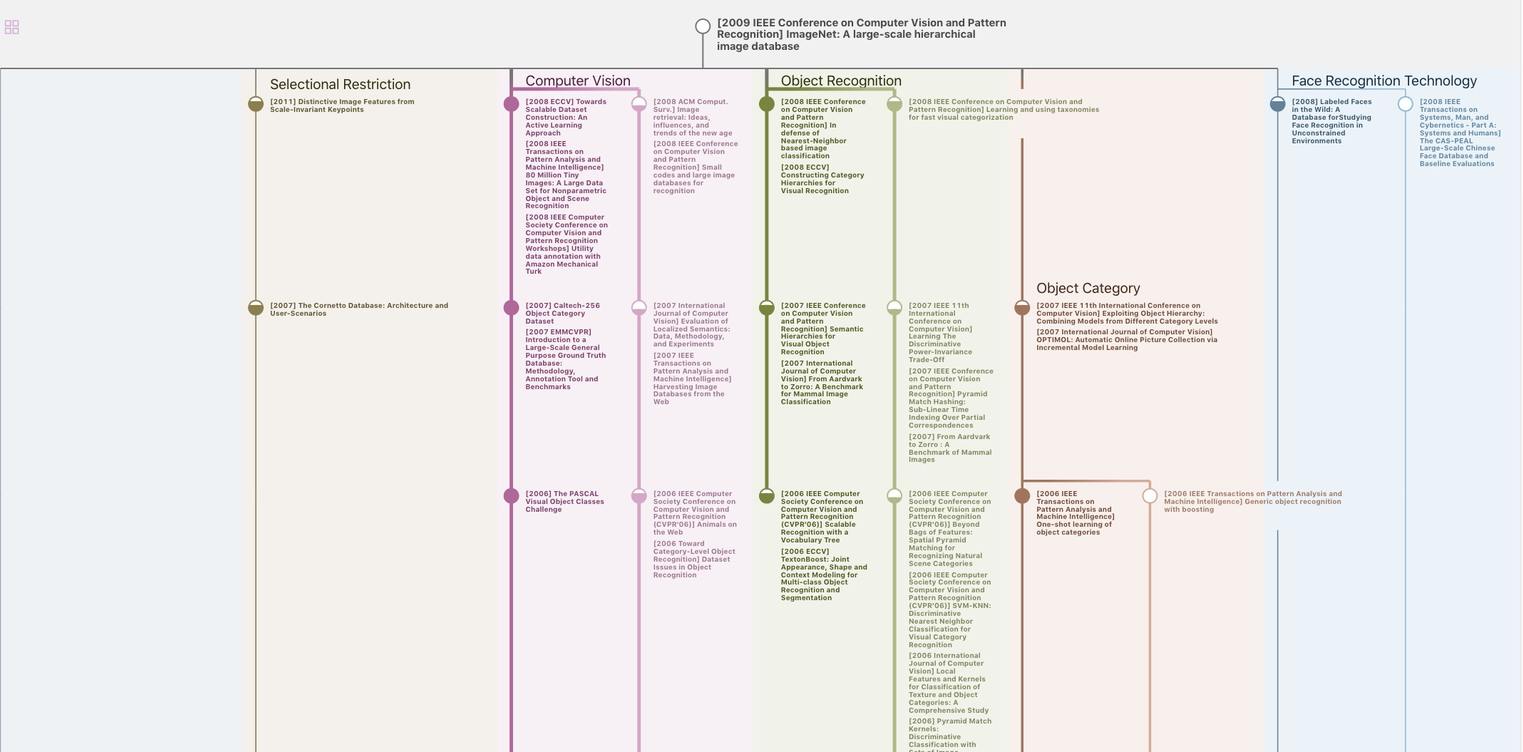
生成溯源树,研究论文发展脉络
Chat Paper
正在生成论文摘要