Self-attention-based long temporal sequence modeling method for temporal action detection.
Neurocomputing(2023)
摘要
Temporal Action Detection (TAD) is a basic and complex task in video understanding. It aims at detecting both the localization and category of actions in a video. The anchor-free TAD methods directly predict the action classes at each location and regress the distances to the boundaries. However, current anchor-free models based on convolutional neural network encode spatiotemporal sequences by 3D convolution networks. Due to the limited receptive field and the basic prior of the translation invariance, effective long temporal sequence modeling cannot be achieved. As a result, these methods cannot effectively detect temporal boundaries. To solve this problem, we design a novel end-to-end self-attention temporal enhancement TAD model, which introduces the Temporal Enhancement module to enhance the temporal feature encoding of the videos and expand the receptive field. Extensive experiments demonstrate that the self-attention Temporal Enhancement model yields an effective improvement on previous work, which improves the performance on THUMOS14 by 1.2%, reaching 53.2% on average mAP. Meanwhile, a competitive result of 34.7% average mAP is achieved on ActivityNet-1.3.
更多查看译文
关键词
Temporal Action Detection,Self-attention,Long temporal sequence modeling,Vision transformer
AI 理解论文
溯源树
样例
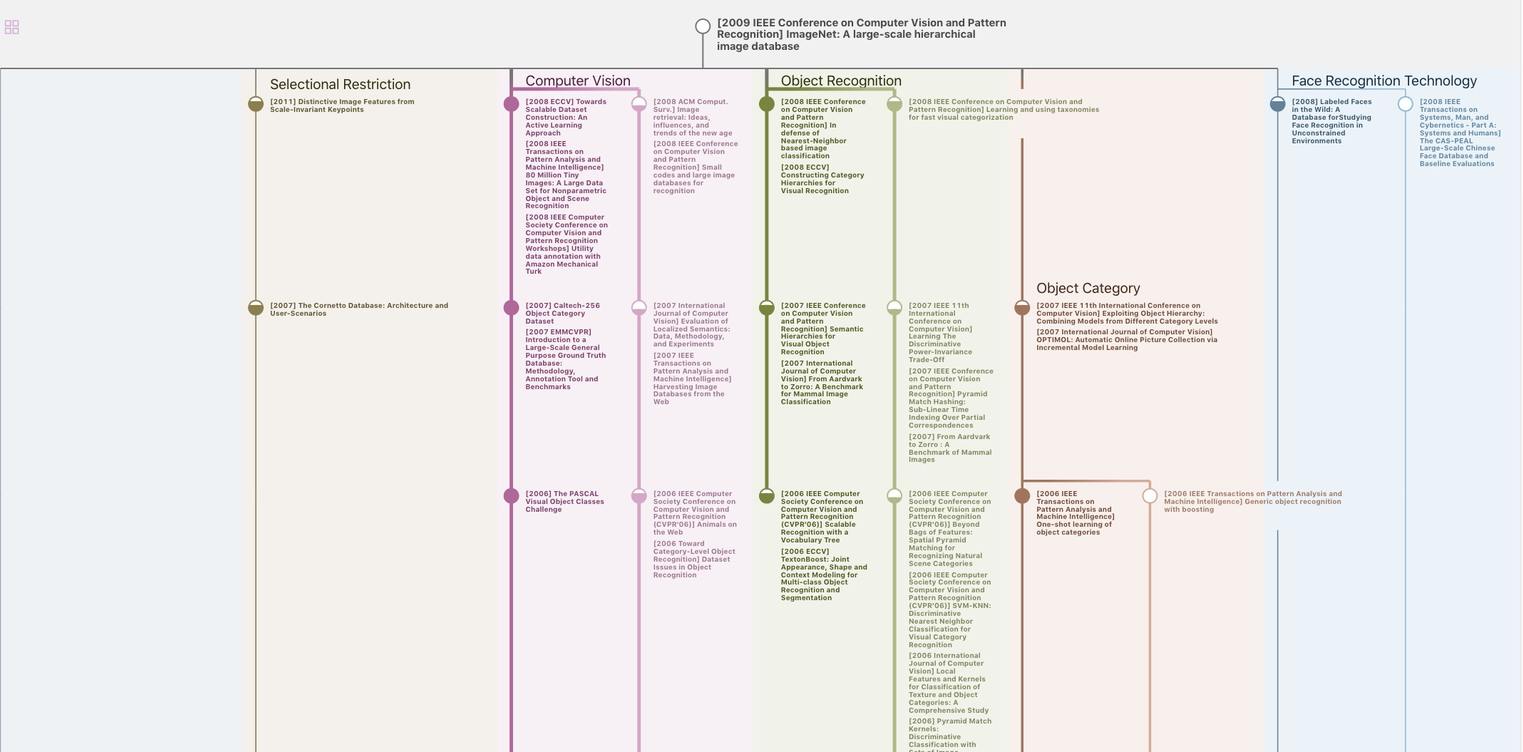
生成溯源树,研究论文发展脉络
Chat Paper
正在生成论文摘要