MULTI-modal radiomics to predict early treatment response from PSA (prostate specific antigen) decline in prostate cancer patients under stereotactic body radiotherapy in MR-Linac
JOURNAL OF RADIATION RESEARCH AND APPLIED SCIENCES(2024)
摘要
Introduction: In prostate cancer Stereotactic Body Radiotherapy (SBRT), there is a lack of prediction in early treatment response which is critical to assist treatment decision making. This retrospective study will predict 1-month post-treatment serum prostate specific antigen (PSA) level as a surrogate of early treatment response for prostate cancer patient treated with SBRT via computed tomography (CT) and magnetic resonance (MR) based radiomics model. Methods: One hundred and nine prostate cancer patients receiving five fractions of SBRT using Elekta Unity MR-Linac were retrospectively recruited into the study. Pre-treatment CT and T2-weighted MR images were processed using RAdiotherapy Data Analysis and Reporting (RADAR) software developed by Department of Health, Technology and Informatics (HTI) at Hong Kong Polytechnic University. A total of 1130 radiomics features were extracted from the prostate clinical target volume (CTV) in CT and MR images. The radiomics model was trained using CT and MR-based radiomic features to predict the serum PSA status one-month post-treatment. The maximum relevance and minimum redundancy (mRMR) feature selection algorithm was implemented to select the final features for model building. The performance of the radiomic model was validated using repeated 3-fold cross-validation. Area under the receiver operating characteristic curve (AUC) was used to evaluate the predictive power of the radiomic model. Results: Highest 8 features from each modality were selected for model building. Averaged AUC in training and testing were 0.83 (SD = 0.04) and 0.77 (SD = 0.08) for CT model. For MR model, the AUC for training and testing were 0.85 (SD = 0.04) and 0.78 (SD = 0.09) respectively. The statistical test showed there is no significant AUC difference in CT vs. MR-based radiomic model (p > 0.05). The combined model (CT + MR) with radiomics features from CT (n = 6) and MR (n = 6) showed AUC of 0.89 (SD = 0.02) and 0.82 (SD = 0.09) for training and testing respectively. The statistical test demonstrated a statistically significant improvement (p < 0.05) when compared to CT alone model but not with MR alone model in response prediction. Conclusion: The results indicate radiomics features from CT & MR have the potential to predict the change of one-month post-treatment serum PSA level for prostate cancer. The multi-modality CT and MR radiomics can successfully predict early PSA results after SBRT in prostate cancer patients. And the prediction power in multi-modalities model is more superior than single modality model. The potential application of the results in clinical environment could assist the clinician in practicing precision medicine based on radiomics model prediction.
更多查看译文
关键词
Radiomics,Prostate,Prediction
AI 理解论文
溯源树
样例
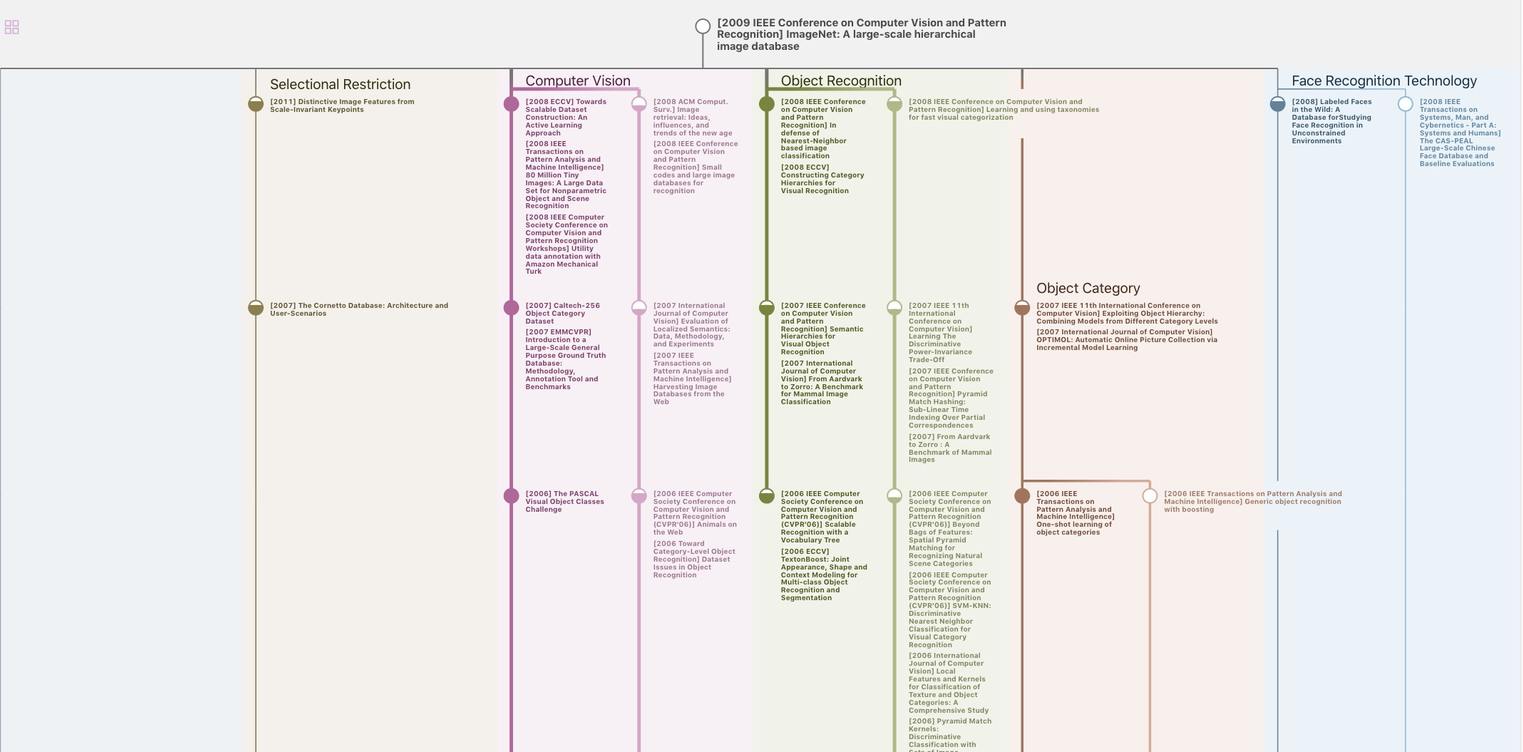
生成溯源树,研究论文发展脉络
Chat Paper
正在生成论文摘要