Comparison of molecular and structural features towards prediction of ionic liquid ionic conductivity for electrochemical applications
Journal of Molecular Liquids(2022)
摘要
Predicting relevant ionic liquid (IL) properties like ionic conductivity using machine learning techniques has attracted significant interest in literature due to the diverse IL design space. The accuracy of these predictions depends on the types of features used to train the machine learning-based models. Previous works using machine learning to predict IL ionic conductivity were limited in dataset size and diversity of ILs. Using graphical neural network and a multi-layer perceptron on a significantly more extensive and diverse dataset, we predict the ionic conductivity of ionic liquids. We establish a system-atic comparison by evaluating three feature sets: structural features learned through graph neural net-works, molecular features, and a combination of structural and molecular features. The better conductivity prediction performance observed herein using structural features only (compared to molec-ular features only) reinforces the importance of chemical structure in characterising the ionic conductiv-ity of ILs. The best prediction results were obtained with the model that combined structural and molecular features. Mean absolute error, root mean squared error, and coefficient of determination of 0.470, 0.677 and 0.937, respectively, were obtained by the model with the combined feature sets, using a dataset of 2,684 ILs. A well-informed choice of what molecular features to combine with structural fea-tures is critical for better property prediction accuracy.(c) 2022 Elsevier B.V. All rights reserved.
更多查看译文
关键词
Machine Learning,Ionic Liquids,Ionic Conductivity,Graph Neural Networks (GNN)
AI 理解论文
溯源树
样例
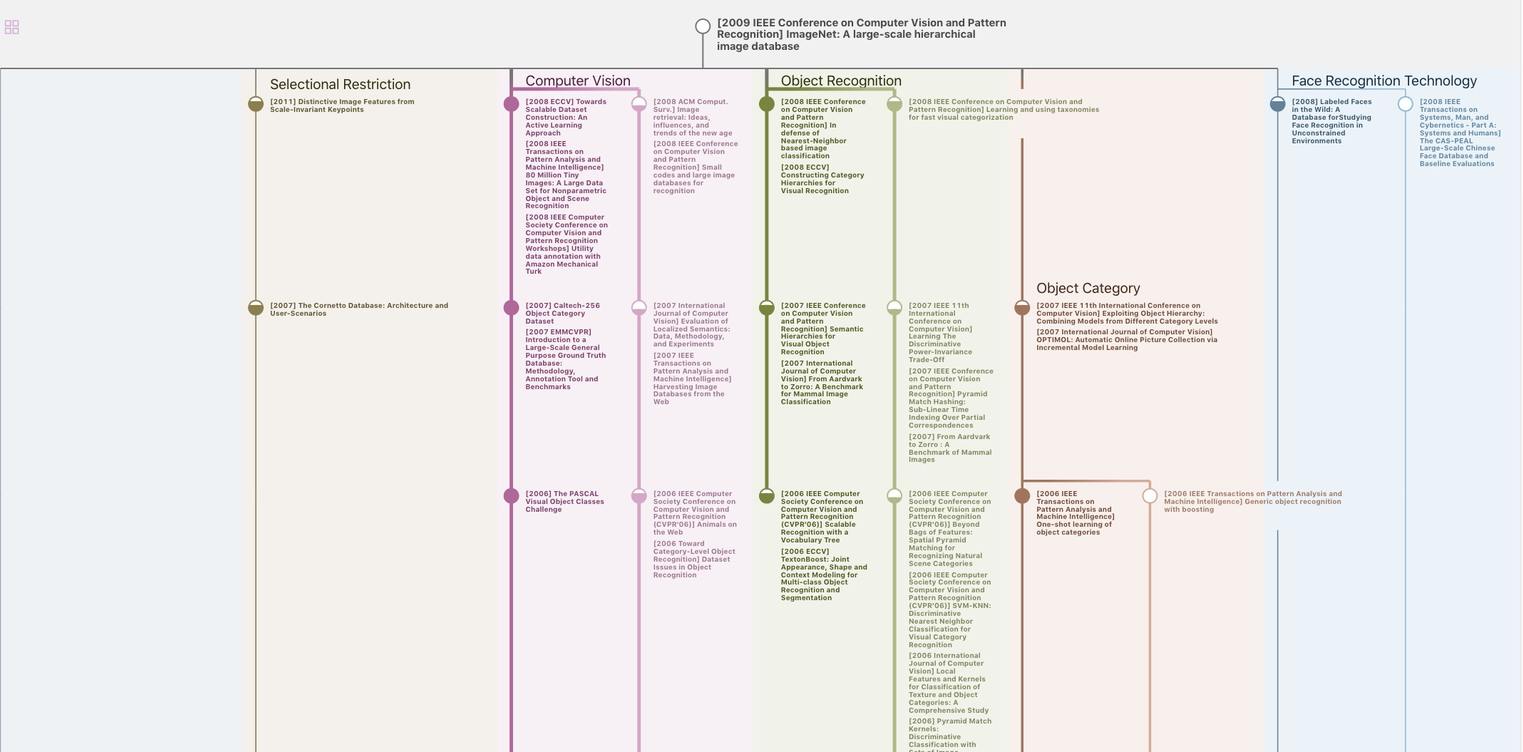
生成溯源树,研究论文发展脉络
Chat Paper
正在生成论文摘要