Divergence vs. Decision P-values: A Distinction Worth Making in Theory and Keeping in Practice
arxiv(2023)
摘要
There are two distinct definitions of 'P-value' for evaluating a proposed hypothesis or model for the process generating an observed dataset. The original definition starts with a measure of the divergence of the dataset from what was expected under the model, such as a sum of squares or a deviance statistic. A P-value is then the ordinal location of the measure in a reference distribution computed from the model and the data, and is treated as a unit-scaled index of compatibility between the data and the model. In the other definition, a P-value is a random variable on the unit interval whose realizations can be compared to a cutoff alpha to generate a decision rule with known error rates under the model and specific alternatives. It is commonly assumed that realizations of such decision P-values always correspond to divergence P-values. But this need not be so: Decision P-values can violate intuitive single-sample coherence criteria where divergence P-values do not. It is thus argued that divergence and decision P-values should be carefully distinguished in teaching, and that divergence P-values are the relevant choice when the analysis goal is to summarize evidence rather than implement a decision rule.
更多查看译文
关键词
distinction worth,decision,p-values
AI 理解论文
溯源树
样例
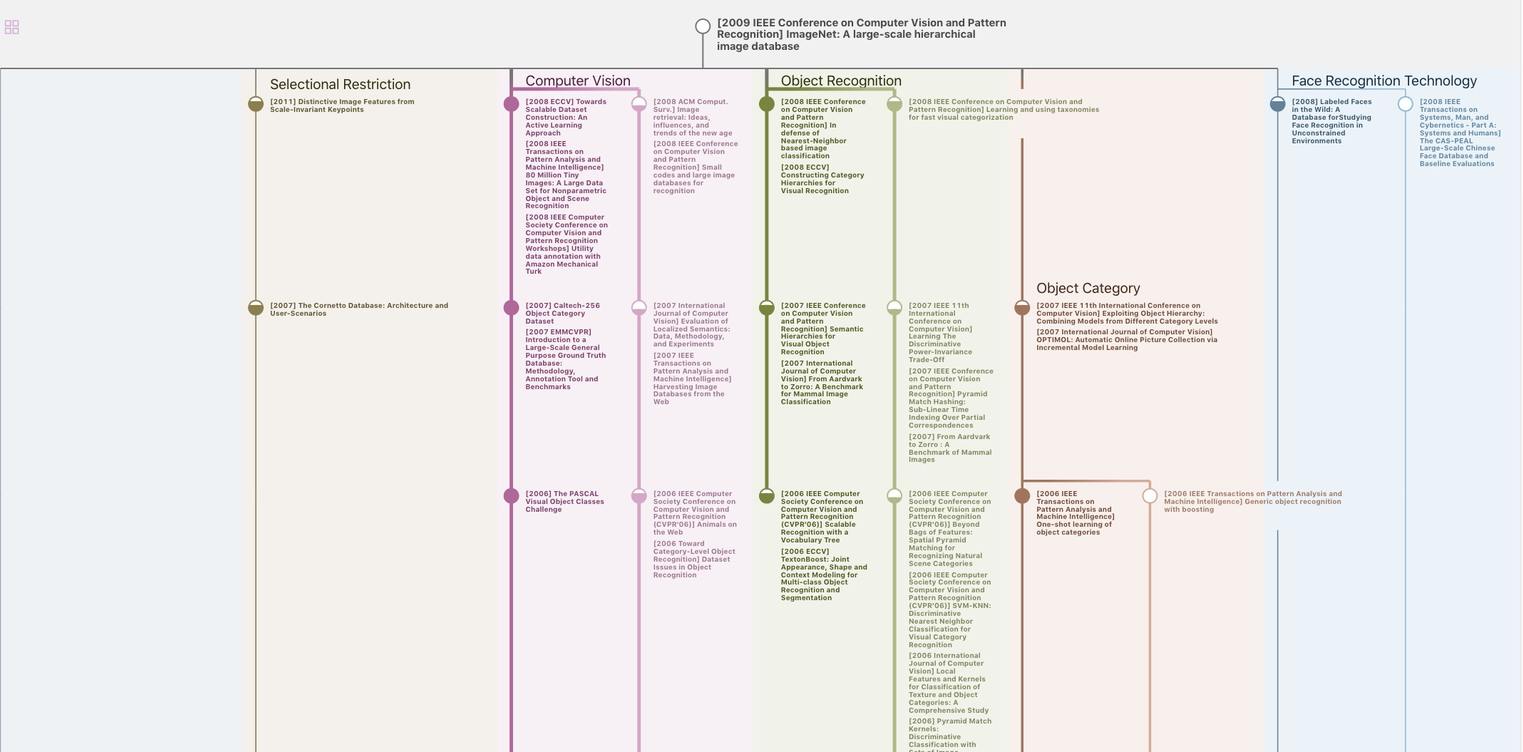
生成溯源树,研究论文发展脉络
Chat Paper
正在生成论文摘要