Derivation and validation of machine learning models for preoperative estimation of microvascular invasion risk in hepatocellular carcinoma.
Annals of Translational Medicine(2023)
摘要
Background:Hepatocellular carcinoma (HCC) represents a considerable burden to patients and health systems. Microvascular invasion (MVI) is a significant risk factor for HCC recurrence and survival after hepatectomy. We aimed to establish a preoperative MVI prediction model based on readily available clinical and radiographic characteristics using machine learning algorithms.
Methods:Two independent cohorts of patients with HCC who underwent hepatectomy were included in the analysis and divided into a derivation set (466 patients), an internal validation set (182 patients), and an external validation set (140 patients). Least absolute shrinkage and selection operator (LASSO) analysis was used to optimize variable selection. We constructed the MVI prediction model using several machine learning algorithms, including logistic regression, k-nearest neighbors, support vector machine, decision tree, random forest, extreme gradient boosting, and neural network. Performance of the model was assessed in terms of discrimination, calibration, and clinical usefulness.
Results:The three most significant variables associated with MVI-α-fetoprotein, protein induced by vitamin K absence or antagonist-II, and tumor size-were identified by the LASSO analysis. Among the machine learning algorithms, the logistic regression model achieved the largest area under the receiver operating characteristic curve and was presented in the form of a user-friendly, online calculator. The concordance (C)-statistic of the model was 0.745 [95% confidence interval (CI): 0.701-0.790] for the derivation set, 0.771 (95% CI: 0.703-0.839) for the internal validation set, and 0.812 (95% CI: 0.734-0.891) for the external validation set. The Hosmer-Lemeshow calibration test and calibration plot indicated a good fit for all 3 data sets. Decision curve analysis showed the model was clinically useful.
Conclusions:This study provided a convenient and explainable approach for MVI prediction before surgical intervention. Our model may assist clinicians in determining the optimal therapeutic modality and facilitate precision medicine for HCC.
更多查看译文
关键词
Hepatocellular carcinoma (HCC),machine learning,microvascular invasion (MVI),nomogram,prediction model
AI 理解论文
溯源树
样例
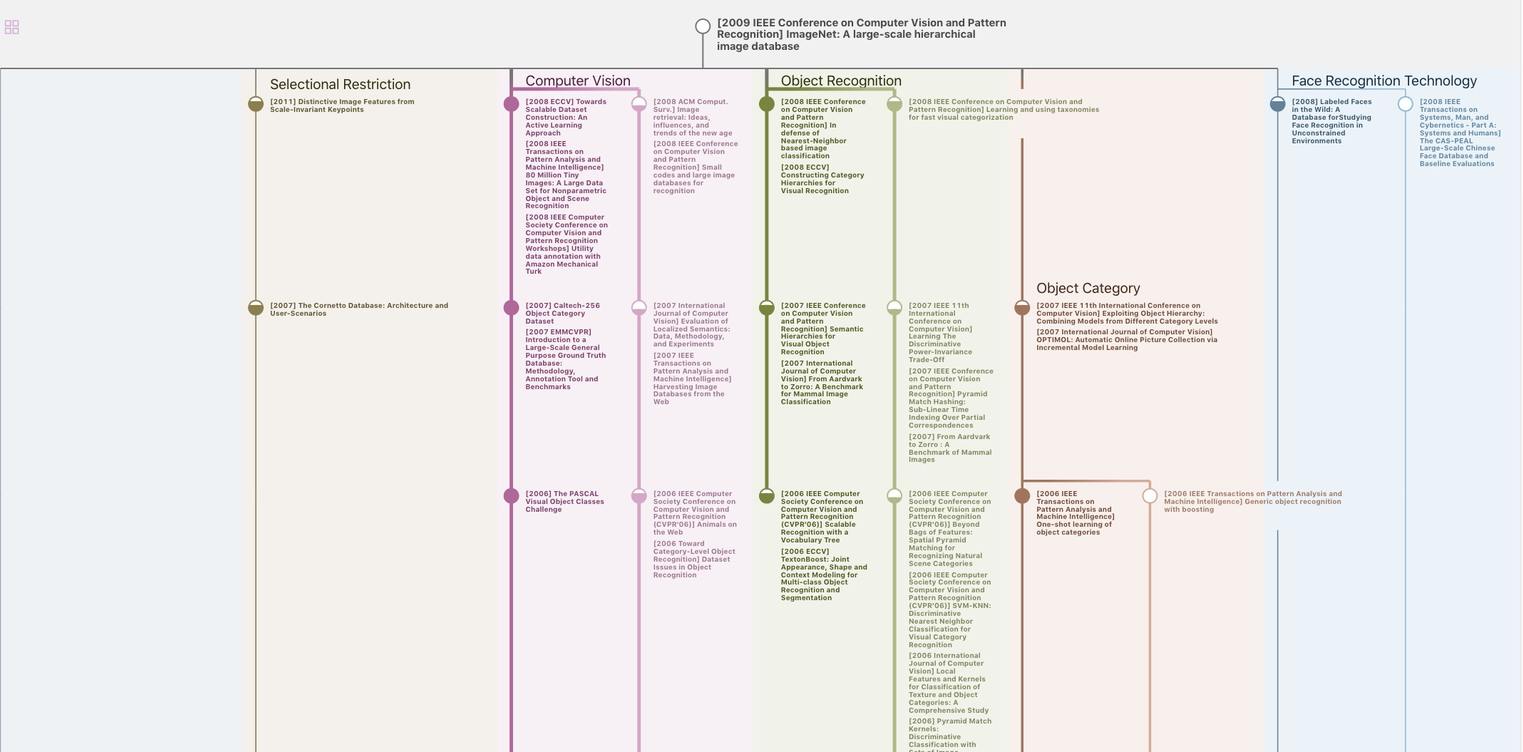
生成溯源树,研究论文发展脉络
Chat Paper
正在生成论文摘要