Super-resolution DOA estimation for correlated off-grid signals via deep estimator
Journal of Systems Engineering and Electronics(2022)
摘要
This paper develops a deep estimator framework of deep convolution networks (DCNs) for super-resolution direction of arrival (DOA) estimation. In addition to the scenario of correlated signals, the quantization errors of the DCN are the major challenge. In our deep estimator framework, one DCN is used for spectrum estimation with quantization errors, and the remaining two DCNs are used to estimate quantization errors. We propose training our estimator using the spatial sampled covariance matrix directly as our deep estimator's input without any feature extraction operation. Then, we reconstruct the original spatial spectrum from the spectrum estimate and quantization errors estimate. Also, the feasibility of the proposed deep estimator is analyzed in detail in this paper. Once the deep estimator is appropriately trained, it can recover the correlated signals' spatial spectrum fast and accurately. Simulation results show that our estimator performs well in both resolution and estimation error compared with the state-of-the-art algorithms.
更多查看译文
关键词
off-grid direction of arrival (DOA) estimation,deep convolution network (DCN),correlated signal,quantization error,super-resolution
AI 理解论文
溯源树
样例
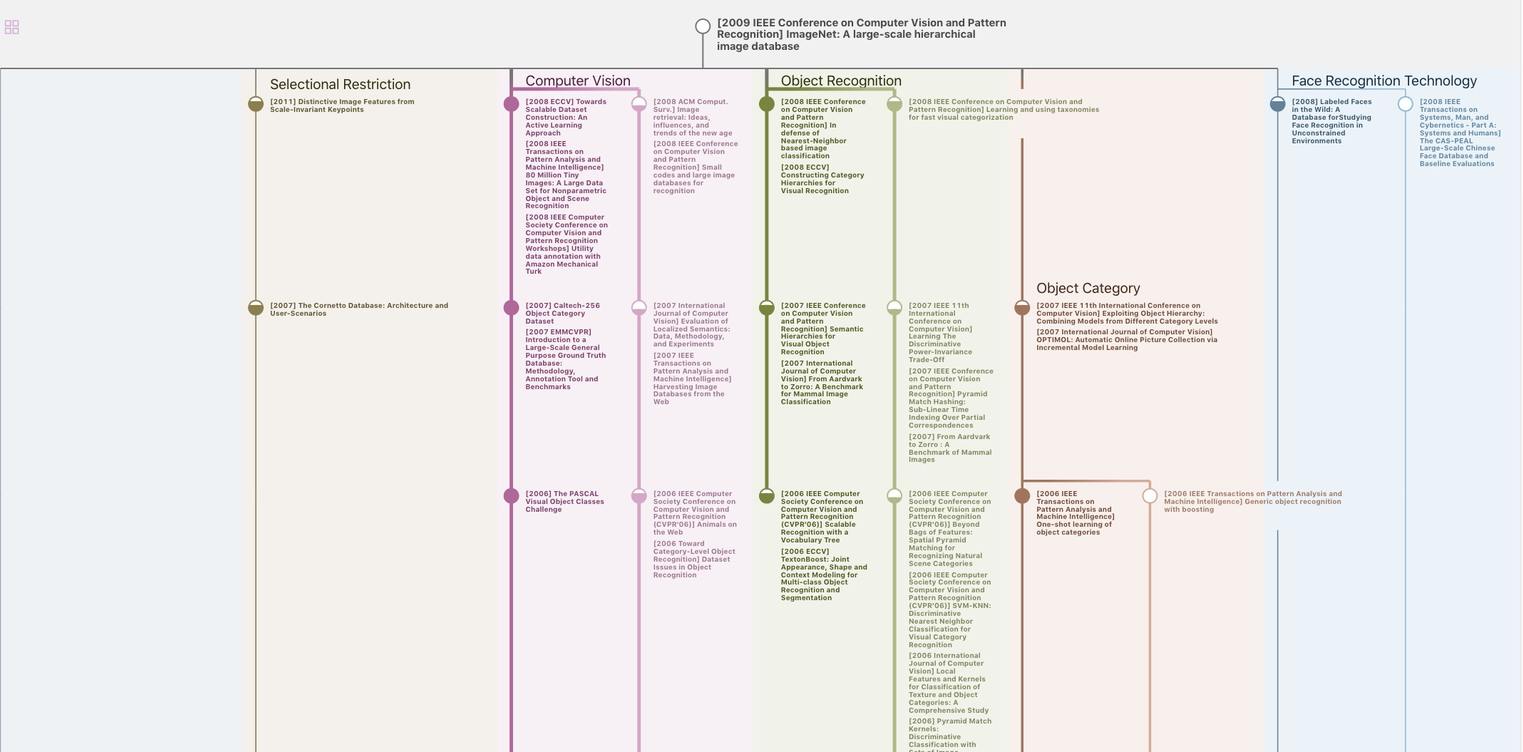
生成溯源树,研究论文发展脉络
Chat Paper
正在生成论文摘要