Graph Regularized Nonnegative Matrix Factorization for Community Detection in Attributed Networks
IEEE Transactions on Network Science and Engineering(2023)
摘要
Community detection has become an important research topic in machine learning due to the proliferation of network data. However, most existing methods have been developed based on only exploiting the topology structures of the network, which can result in missing the advantage of using the nodes' attribute information. As a result, it is expected that much valuable information that could be used to improve the quality of discovered communities will be ignored. To solve this limitation, we propose a novel Augment Graph Regularization Nonnegative Matrix Factorization for Attributed Networks (AGNMF-AN) method, which is simple yet effective. Firstly, Augment Attributed Graph (AAG) is applied to combine both the topological structure and attributed nodes of the network. Secondly, we introduced an effective framework to update the affinity matrix, in which the affinity matrix's weight in each iteration is modified adaptively instead of using a fixed affinity matrix in the classical graph regularization-based nonnegative matrix factorization methods. Thirdly, the
$l_{2,1}$
-norm is utilized to reduce the effect of random noise and outliers in the quality of structure community. Experimental results show that our method performs unexpectedly well in comparison to existing state-of-the-art methods in attributed networks.
更多查看译文
关键词
Complex network,community detection,nonnegative matrix factorization,attributed networks
AI 理解论文
溯源树
样例
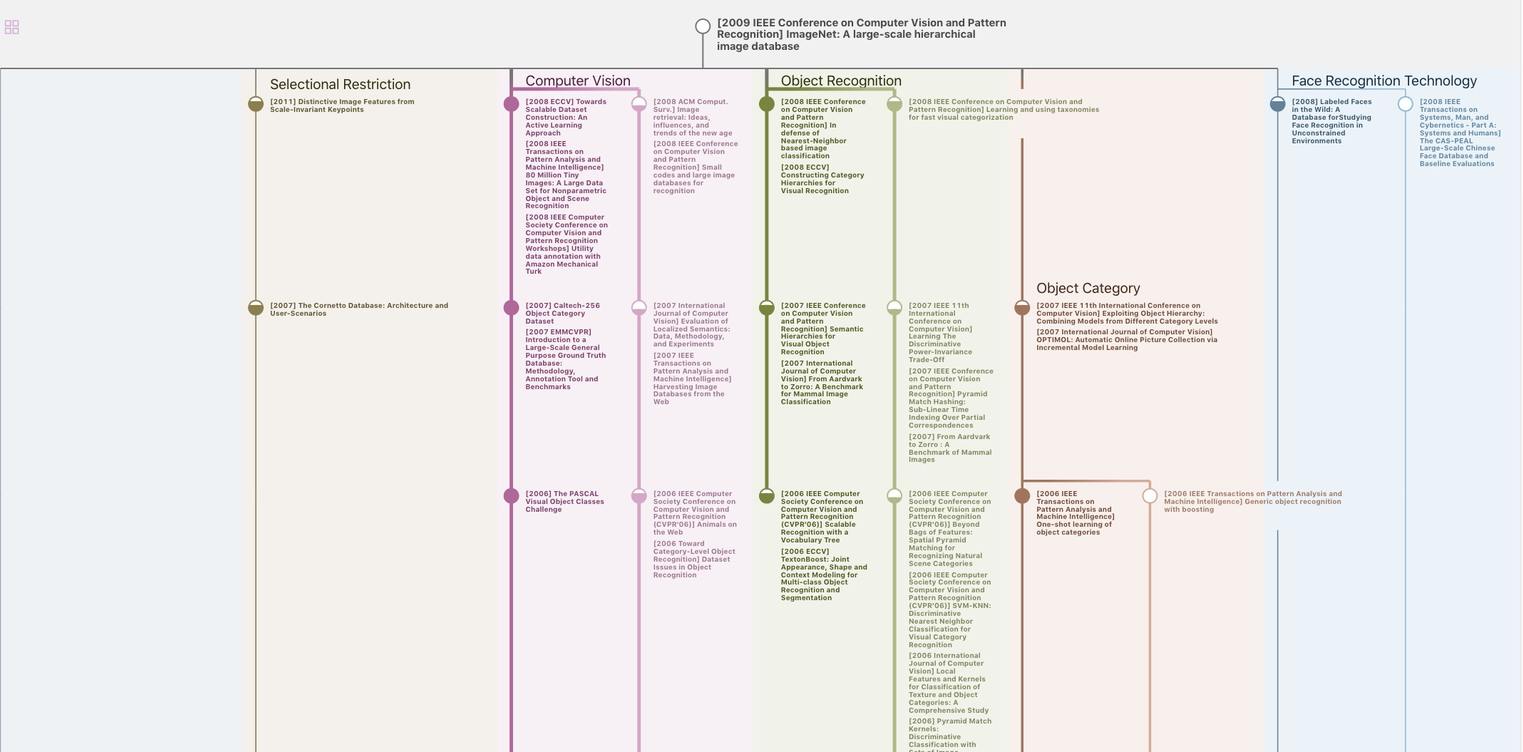
生成溯源树,研究论文发展脉络
Chat Paper
正在生成论文摘要