Multiclass Semantic Segmentation to Identify Anatomical Sub-Regions of Brain and Measure Neuronal Health in Parkinson's Disease
CoRR(2023)
摘要
Automated segmentation of anatomical sub-regions with high precision has become a necessity to enable the quantification and characterization of cells/ tissues in histology images. Currently, a machine learning model to analyze sub-anatomical regions of the brain to analyze 2D histological images is not available. The scientists rely on manually segmenting anatomical sub-regions of the brain which is extremely time-consuming and prone to labeler-dependent bias. One of the major challenges in accomplishing such a task is the lack of high-quality annotated images that can be used to train a generic artificial intelligence model. In this study, we employed a UNet-based architecture, compared model performance with various combinations of encoders, image sizes, and sample selection techniques. Additionally, to increase the sample set we resorted to data augmentation which provided data diversity and robust learning. In this study, we trained our best fit model on approximately one thousand annotated 2D brain images stained with Nissl/ Haematoxylin and Tyrosine Hydroxylase enzyme (TH, indicator of dopaminergic neuron viability). The dataset comprises of different animal studies enabling the model to be trained on different datasets. The model effectively is able to detect two sub-regions compacta (SNCD) and reticulata (SNr) in all the images. In spite of limited training data, our best model achieves a mean intersection over union (IOU) of 79% and a mean dice coefficient of 87%. In conclusion, the UNet-based model with EffiecientNet as an encoder outperforms all other encoders, resulting in a first of its kind robust model for multiclass segmentation of sub-brain regions in 2D images.
更多查看译文
关键词
parkinson,measure neuronal health,segmentation,brain,sub-regions
AI 理解论文
溯源树
样例
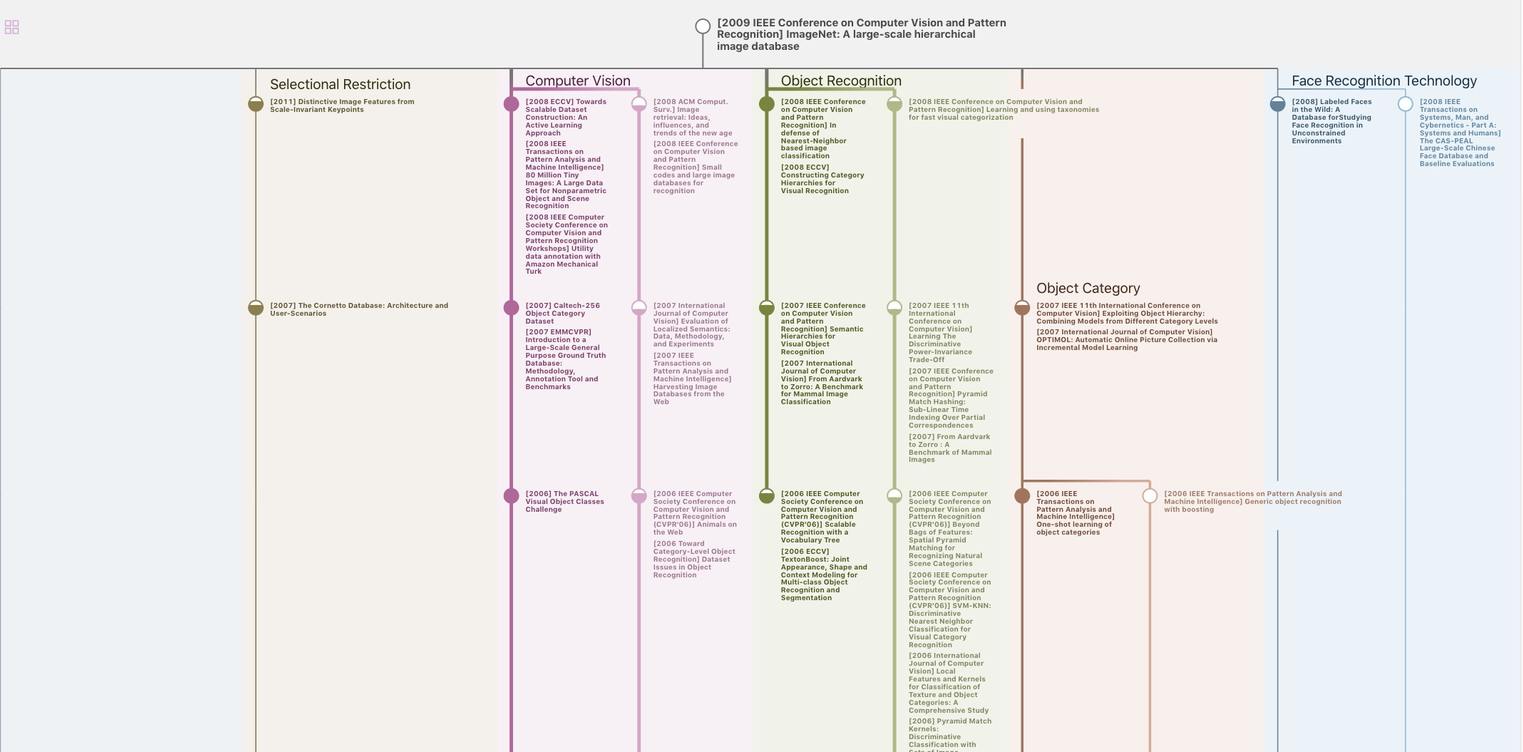
生成溯源树,研究论文发展脉络
Chat Paper
正在生成论文摘要