Deep Learning for Short-Latency Epileptic Seizure Detection with Probabilistic Classification
arxiv(2023)
摘要
In this manuscript, we propose a novel deep learning (DL)-based framework intended for obtaining short latency in real-time electroencephalogram-based epileptic seizure detection using multiscale 3D convolutional neural networks. We pioneer converting seizure detection task from traditional binary classification of samples from ictal and interictal periods to probabilistic classification of samples from interictal, ictal, and crossing periods. We introduce a crossing period from seizure-oriented EEG recording and propose a labelling rule using soft-label for samples from the crossing period to build a probabilistic classification task. A novel multiscale short-time Fourier transform feature extraction method and 3D convolution neural network architecture are proposed to accurately capture predictive probabilities of samples. Furthermore, we also propose rectified weighting strategy to enhance predictive probabilities, and accumulative decision-making rule to achieve short detection latency. We implement leave-one-seizure-out cross validation on two prevalent datasets -- CHB-MIT scalp EEG dataset and SWEC-ETHZ intracranial EEG dataset. Eventually, the proposed algorithm achieved 94 out of 99 seizures detected during the crossing period, averaged 14.84% rectified predictive ictal probability (RPIP) errors of crossing samples, 2.3 s detection latency, 0.32/h false detection rate on CHB-MIT dataset, meanwhile 84 out of 89 detected seizures, 16.17% RPIP errors, 4.7 s detection latency, and 0.75/h FDR are achieved on SWEC-ETHZ dataset. The obtained detection latencies are at least 50% faster than state-of-the-art results reported in previous studies.
更多查看译文
AI 理解论文
溯源树
样例
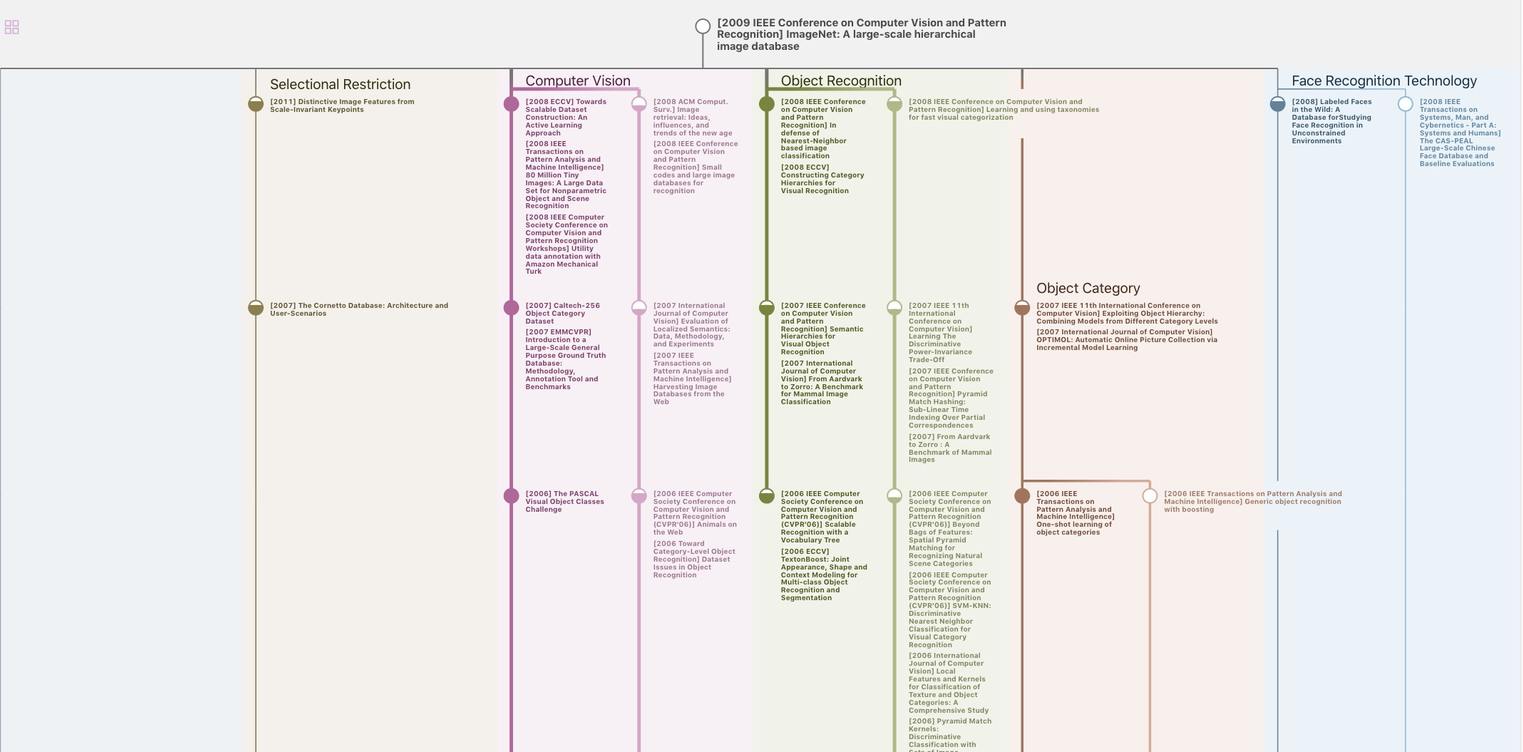
生成溯源树,研究论文发展脉络
Chat Paper
正在生成论文摘要