eXplainable Ensemble Strategy using distinct and restrict learning biases: A case study on the Brazilian Forest
Applied Soft Computing(2023)
摘要
Supervised learning algorithms consider different learning biases from the universe of all admissible functions to induce classifiers. When using ensembles, one takes advantage of different biases typically built from the same algorithm to combine complementary classifiers into a single model, such as Random Forest, that builds up several trees from different attributes and examples. This paper innovates ensemble strategies by explaining and exploring distinct, restrict, and complementary biases from different algorithms. Multi-bias classifiers are combined using Fuzzy rules to execute symbolic reasoning and explain how each learning bias contributes to the final classification results. The contributions of our work are twofold: first, the proposed approach looks for the most suitable learner by individually analyzing the attributes of each new instance, and second, the process used to perform such a search is based on inferences run on fuzzy rules, that uses IF–THEN structures, which are interpretable, thus allowing to explain the process used to select the best learner. Finally, it is worth emphasizing that our approach was applied to the Brazilian biodiversity dataset to corroborate that, even working on hundreds of examples, results are promising, thus stimulating studies on biodiversity and the design of sustainable economic solutions.
更多查看译文
关键词
Supervised learning,Ensemble method,Fuzzy Systems
AI 理解论文
溯源树
样例
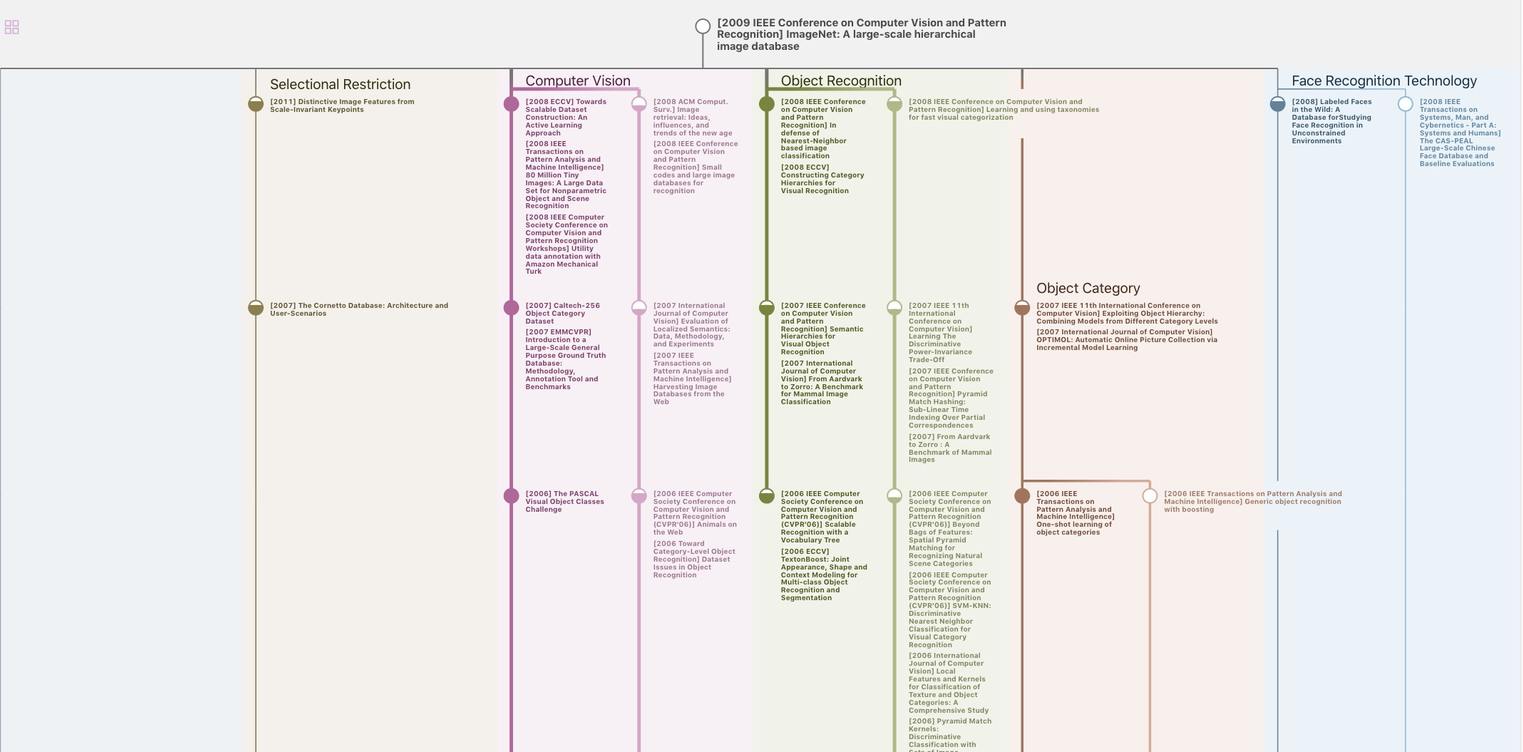
生成溯源树,研究论文发展脉络
Chat Paper
正在生成论文摘要