An interpretable convolutional neural network for nuclear power plant abnormal events
Applied Soft Computing(2023)
摘要
When an abnormal situation occurs in a nuclear power plant (NPP), operators must properly diagnose the event among hundreds of possible abnormal events. To do so, they monitor changes in plant parameters and confirm the correct abnormal operating procedure when the parameters match the entry conditions described in that procedure. In this process, operators are burdened with a lot of information. The purpose of this study is to optimize the number of main parameters to be monitored for abnormal state diagnosis in NPPs by clarifying the classification process with a deep learning model. To increase the transparency of the trained convolutional neural network model in the diagnosis of 10 different NPP states, we applied three explanation techniques: saliency mapping, guided gradient-weighted class activation mapping, and deep learning important features + Shapley values. These techniques can highlight the particular input parameters that are the most influential to the classification. Each transparency result confirmed that the parameters selected by these techniques can be a key rationale in NPP abnormal state diagnosis. By averaging the results of the two methods with the highest transparency performance, it was possible to intuitively classify all 10 NPP states with only 6 optimized monitoring parameters.
更多查看译文
关键词
Nuclear power plant,Abnormality diagnosis,Explanation method
AI 理解论文
溯源树
样例
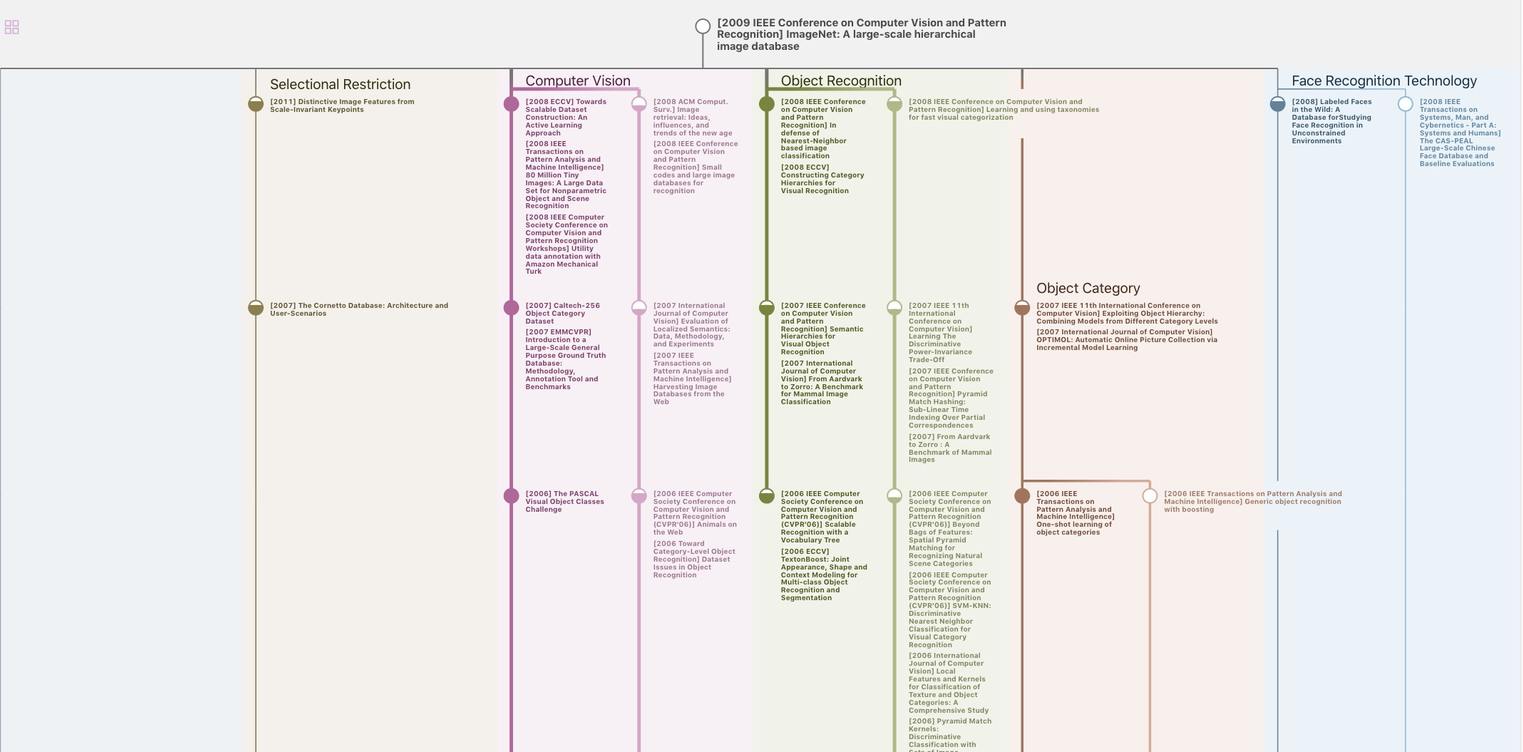
生成溯源树,研究论文发展脉络
Chat Paper
正在生成论文摘要