On-tree apple fruit size estimation using stereo vision with deep learning-based occlusion handling
Biosystems Engineering(2023)
摘要
Apple (Malus domestica) fruit size plays an integral role in orchard management decision -making, particularly during chemical thinning, fruit quality assessment, and yield pre-diction. A machine vision system was developed using stereo cameras synchronised to a custom-built LED strobe to perform on-tree sizing of fruit in images with high measure-ment accuracy. Two deep neural network models (Faster R-CNN and Mask R-CNN) were trained to detect fruit candidates for sizing followed by extrapolation of occluded fruit regions to improve size estimation. The segmented fruit shapes were converted to metric surface areas and diameters using spatial resolutions and depth information from the stereo cameras. Monthly field trials from June to October using the camera system were conducted, measuring fruit diameters ranging from 22 to 82 mm, and compared against ground truth diameters. Diameter estimates had a mean absolute error ranging from 1.1 to 4.2 mm for the five-month trial period, an average error of 4.8% compared to ground truth diameter measurements. Standard deviation errors ranged from 0.7 to 1.9 mm. Using neural network models for intelligent sampling of fruit in images followed by extrapolation of missing regions can be an alternative method of handling fruit occlusion in agricultural imaging and improving sizing accuracy.(c) 2022 The Author(s). Published by Elsevier Ltd on behalf of IAgrE. This is an open access article under the CC BY-NC-ND license (http://creativecommons.org/licenses/by-nc-nd/4.0/ ).
更多查看译文
关键词
Apple shape measurement,Machine vision,Fruit segmentation,Image inpainting,Deep learning,Precision agriculture
AI 理解论文
溯源树
样例
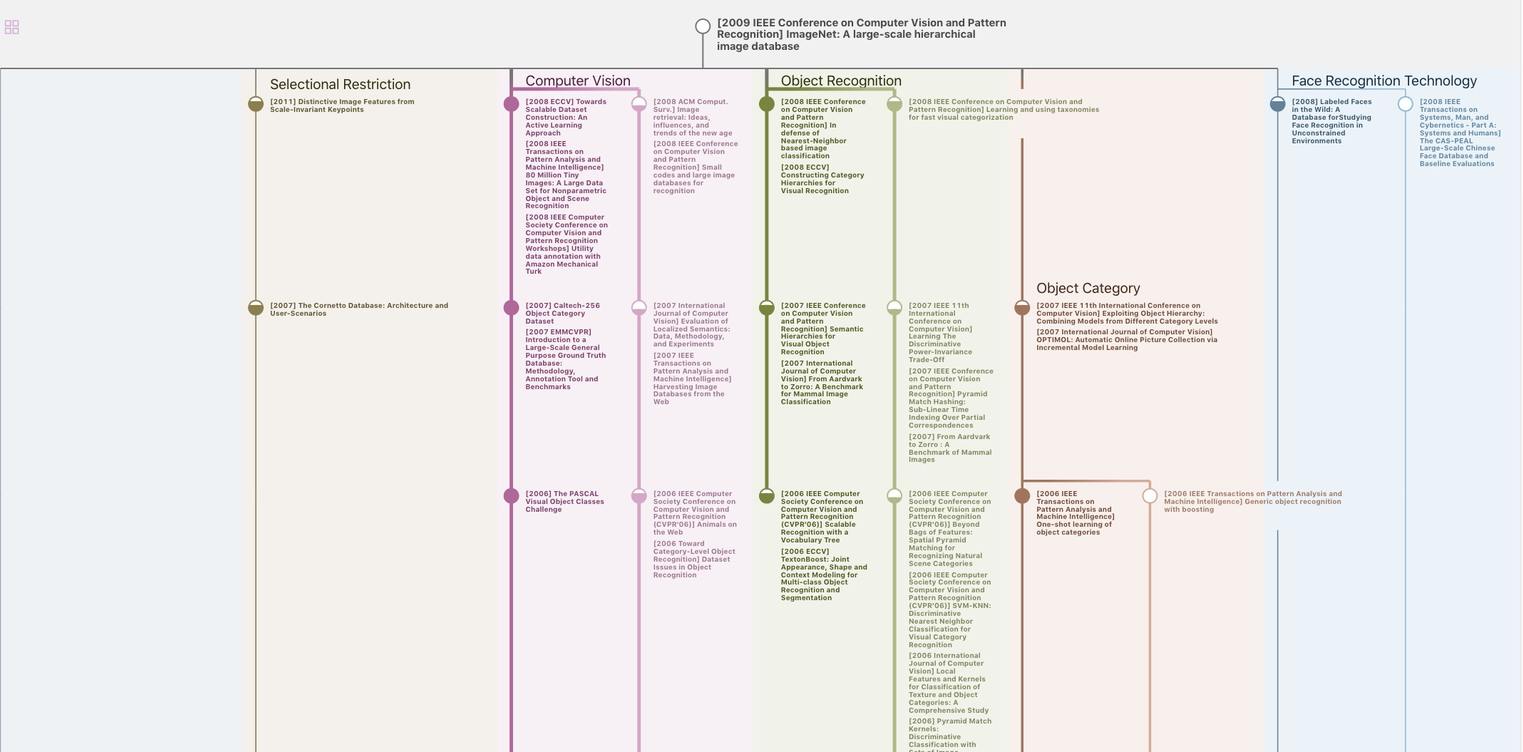
生成溯源树,研究论文发展脉络
Chat Paper
正在生成论文摘要