Semiparametric predictive inference for failure data using first-hitting-time threshold regression
Lifetime Data Analysis(2023)
摘要
The progression of disease for an individual can be described mathematically as a stochastic process. The individual experiences a failure event when the disease path first reaches or crosses a critical disease level. This happening defines a failure event and a first hitting time or time-to-event, both of which are important in medical contexts. When the context involves explanatory variables then there is usually an interest in incorporating regression structures into the analysis and the methodology known as threshold regression comes into play. To date, most applications of threshold regression have been based on parametric families of stochastic processes. This paper presents a semiparametric form of threshold regression that requires the stochastic process to have only one key property, namely, stationary independent increments. As this property is frequently encountered in real applications, this model has potential for use in many fields. The mathematical underpinnings of this semiparametric approach for estimation and prediction are described. The basic data element required by the model is a pair of readings representing the observed change in time and the observed change in disease level, arising from either a failure event or survival of the individual to the end of the data record. An extension is presented for applications where the underlying disease process is unobservable but component covariate processes are available to construct a surrogate disease process. Threshold regression, used in combination with a data technique called Markov decomposition, allows the methods to handle longitudinal time-to-event data by uncoupling a longitudinal record into a sequence of single records. Computational aspects of the methods are straightforward. An array of simulation experiments that verify computational feasibility and statistical inference are reported in an online supplement. Case applications based on longitudinal observational data from The Osteoarthritis Initiative (OAI) study are presented to demonstrate the methodology and its practical use.
更多查看译文
关键词
Bernoulli regression,Cumulant generating function,Disease progression,Failure process,Health process,Latent health,Lévy process,Longitudinal data,Markov decomposition,Osteoarthritis,Surrogate disease process,Stationary independent increments,Wiener process
AI 理解论文
溯源树
样例
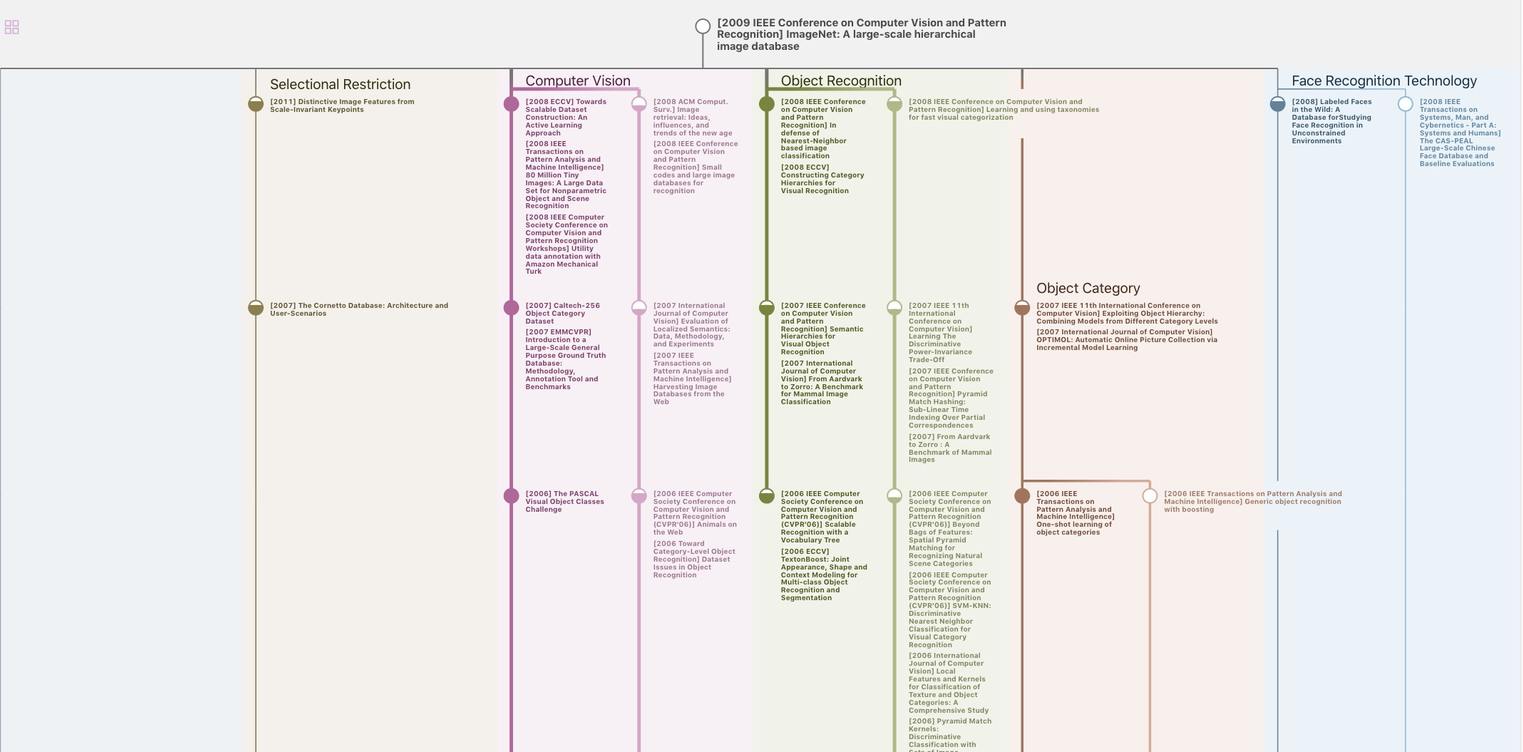
生成溯源树,研究论文发展脉络
Chat Paper
正在生成论文摘要