The topology of fMRI-based networks defines the performance of a graph neural network for the classification of patients with major depressive disorder
Chaos, Solitons & Fractals(2023)
摘要
Deep learning approaches are state-of-the-art computational tools employed at analyzing big data in fundamental and applied science. Recently, they gained popularity in neuroscience and medicine due to their ability to recognize hidden patterns and nonlinear relations in large amounts of nonstationary and ambiguous neuroimaging biomedical data. Analysis of functional connectivity matrices is a perfect example of such a computational task assigned to deep learning. Here, we trained a graph neural network (GNN) to classify the major depressive disorder (MDD) based on the topological features of the brain functional connectivity identified using fMRI technology. We show that the most important feature of the functional brain network is the shortest path, which defines the optimal number of GNN layers to ensure the most accurate classification in patients with MDD. The proposed GNN-based classifier reaches an accuracy of 93%, which is in line with the achievements of the best connectivity-based classifiers for MDD. The maximal F1-score is observed when we input the sparse graph consisting of 2.5% of the connections of the original one, which avoids feeding large amounts of data to the GNN and reduces overfitting.
更多查看译文
关键词
Graph neural network,Thresholding,fMRI,Topology analysis,GNN interpretation,Major depression disorder
AI 理解论文
溯源树
样例
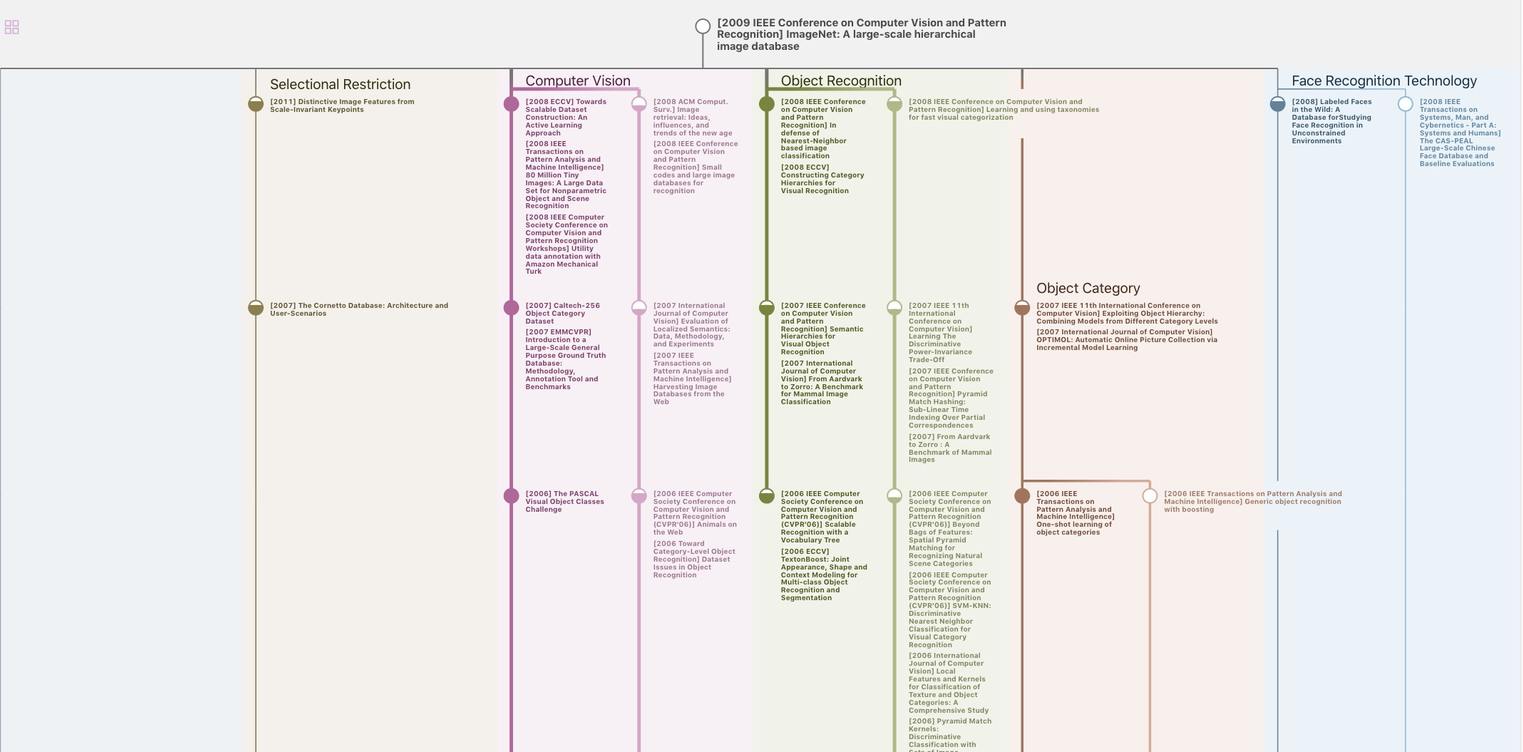
生成溯源树,研究论文发展脉络
Chat Paper
正在生成论文摘要