Soil erosion susceptibility mapping using ensemble machine learning models: A case study of upper Congo river sub-basin
CATENA(2023)
摘要
Despite its large size, the Congo Basin (CB), which spans ten countries, has remained an area of particular interest for scientific discovery due to gaps in Earth science, environmental and hydrological research. This includes mapping soil erosion, which is the dominant form of land degradation in the basin, particularly severe in its upper part. For these reasons, the use of predictive machine learning algorithms (ML-ALs) rather than conventional models is necessary. In particular, the introduction of the ensemble model which is becoming widely popular, but is still little used throughout Africa. In this study, ensemble ML-ALs were applied and aimed at evaluating the predictive power of combining different algorithms such as Support Vector Machine (SVM), Boosted Regression Trees (BRT), Logit Boost (LB) and K-Nearest Neighbor (KNN) with Random Forest (RF) as the base classifier for erosion susceptibility mapping (ESM) in the Elila catchment located in the Upper Congo sub basin. To achieve this goal, 500 erosion sites were identified by the RUSLE model and Google Earth historical maps and then a field survey was conducted to validate the identified erosion sites. The input data were randomly divided into training and test datasets. Fifteen important features were selected using Boruta's approach to produce the ESMs. The accuracy of the models was evaluated using the area under the receiver operating characteristic curve (AUROC) and four statistical measures (sensitivity, accuracy, specificity and kappa index). The overall accuracy in terms of AUROC values shows that RF-BRT (87.26%) was superior to all other algorithms, followed by RF-SVM, RF-KNN, and RF-LB with AUROC values ranging from 86.51%, and 85.37%, to 84.21%, respectively. In conclusion, the results of this work can be used to control and prevent erosion throughout the CB, and the methodology of this work can be useful in similar geo-environmental characteristic sites.
更多查看译文
关键词
Erosion susceptibility mapping,Boruta approach,Random forest,Boosted regression trees,Ensemble machine learning,Congo basin
AI 理解论文
溯源树
样例
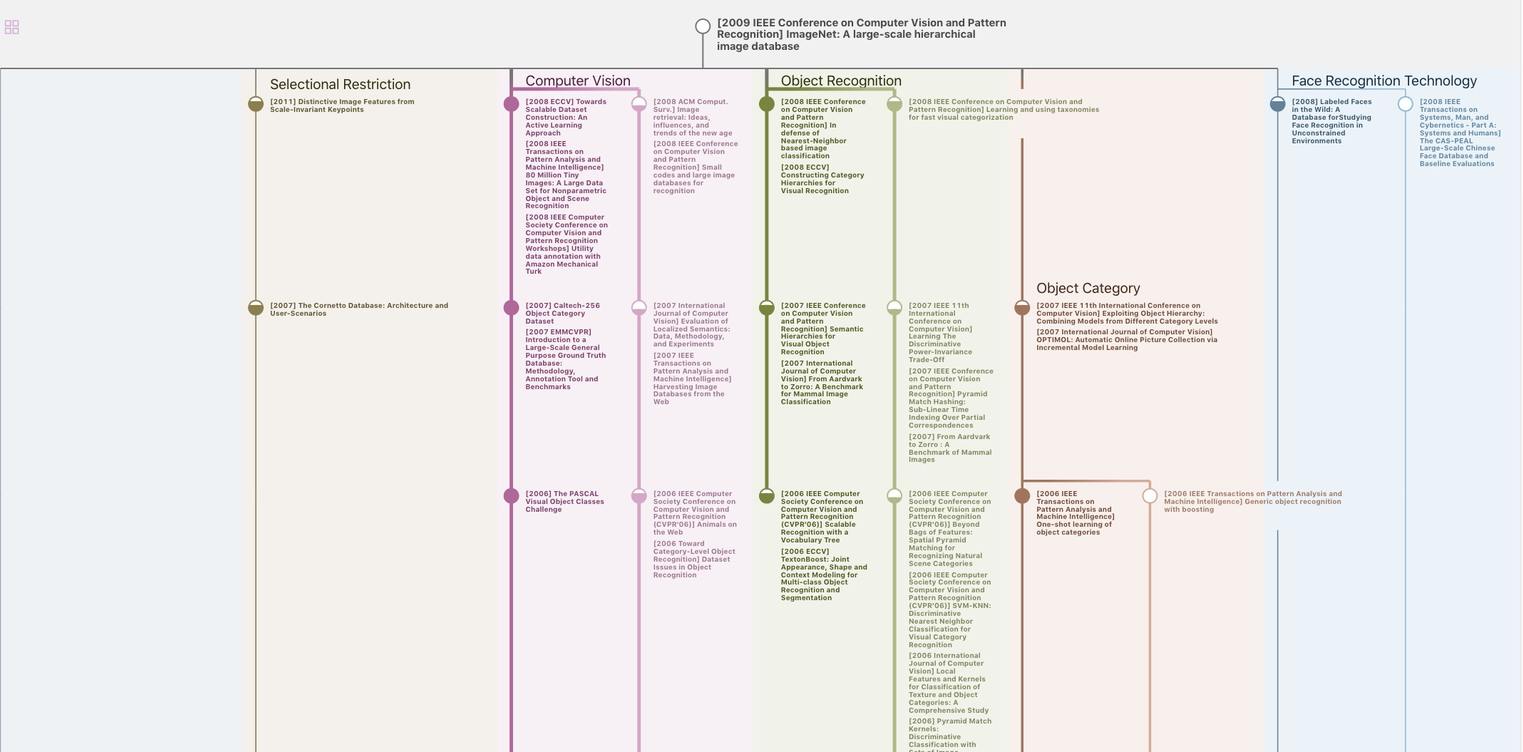
生成溯源树,研究论文发展脉络
Chat Paper
正在生成论文摘要