Graph neural network modeling for ethane recovery evaluation in RSV process
Geoenergy Science and Engineering(2023)
摘要
In the oil and gas industry, the Recycle Split Vapor (RSV) process is a widely-used technology for ethane recovery. It is of particular importance for industrial production to continually track ethane recovery affected by the running status of RSV. The commonly adopted method for this purpose is to aperiodically sample the output gas for laboratory test, which is time consuming and not in time. To address this problem, in this work we develop a graph neural network model for ethane recovery evaluation, which aims at diagnosing ethane recovery quality automatically and timely according to the temperature variations of the sensor array on the demethanizer. Specifically, we use a graph attentional neural network to establish a map between ethane recovery quality and the overall temperature dynamics of the sensors distributed on the demethanizer, where the sensors are abstracted by graph nodes and the spatial connections between sensors are denoted by the edges in the graph so that the temporal and spatial correlations between various temperature sensors on the demethanizer can be captured and the features of temperature vibration can be learned. By leveraging the learned features, the classifier makes “qualified/unqualified” prediction for ethane recovery quality. Moreover, the abnormal sensors are located based on the learned attention scores on the edges, which may guide the operators to coordinate the corresponding valves. The results on extensive experiments show that the method can effectively evaluate the quality of ethane recovery and infer the sensor points that may cause the disqualification.
更多查看译文
关键词
ethane recovery evaluation,graph neural network,neural network,rsv process
AI 理解论文
溯源树
样例
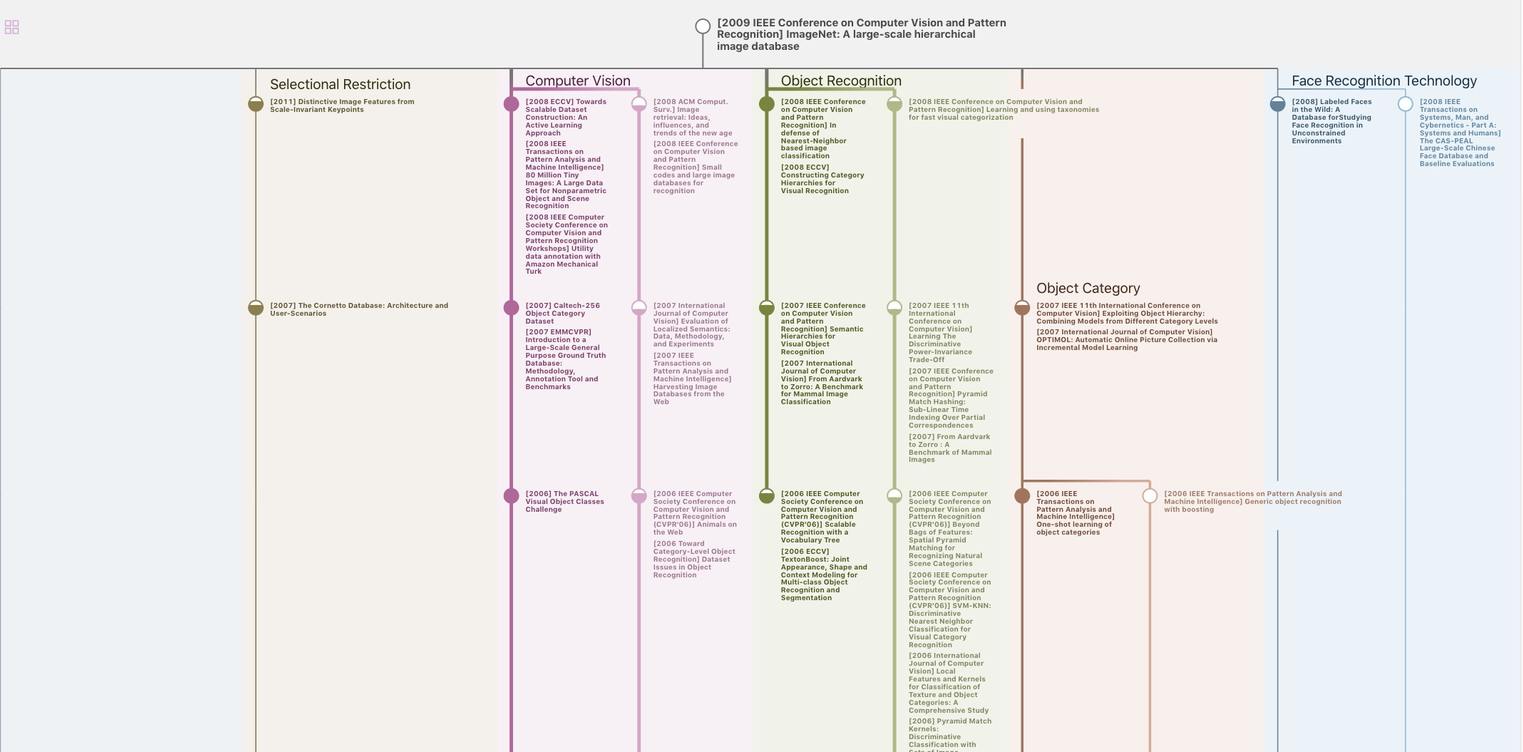
生成溯源树,研究论文发展脉络
Chat Paper
正在生成论文摘要