Rotating machinery fault detection and diagnosis based on deep domain adaptation: A survey
Chinese Journal of Aeronautics(2023)
摘要
In practical mechanical fault detection and diagnosis, it is difficult and expensive to collect enough large-scale supervised data to train deep networks. Transfer learning can reuse the knowledge obtained from the source task to improve the performance of the target task, which performs well on small data and reduces the demand for high computation power. However, the detection performance is significantly reduced by the direct transfer due to the domain difference. Domain adaptation (DA) can transfer the distribution information from the source domain to the target domain and solve a series of problems caused by the distribution difference of data. In this survey, we review various current DA strategies combined with deep learning (DL) and analyze the principles, advantages, and disadvantages of each method. We also summarize the application of DA combined with DL in the field of fault diagnosis. This paper provides a summary of the research results and proposes future work based on analysis of the key technologies.
更多查看译文
关键词
Deep learning,Domain adaptation,Fault detection and diagnosis,Transfer learning
AI 理解论文
溯源树
样例
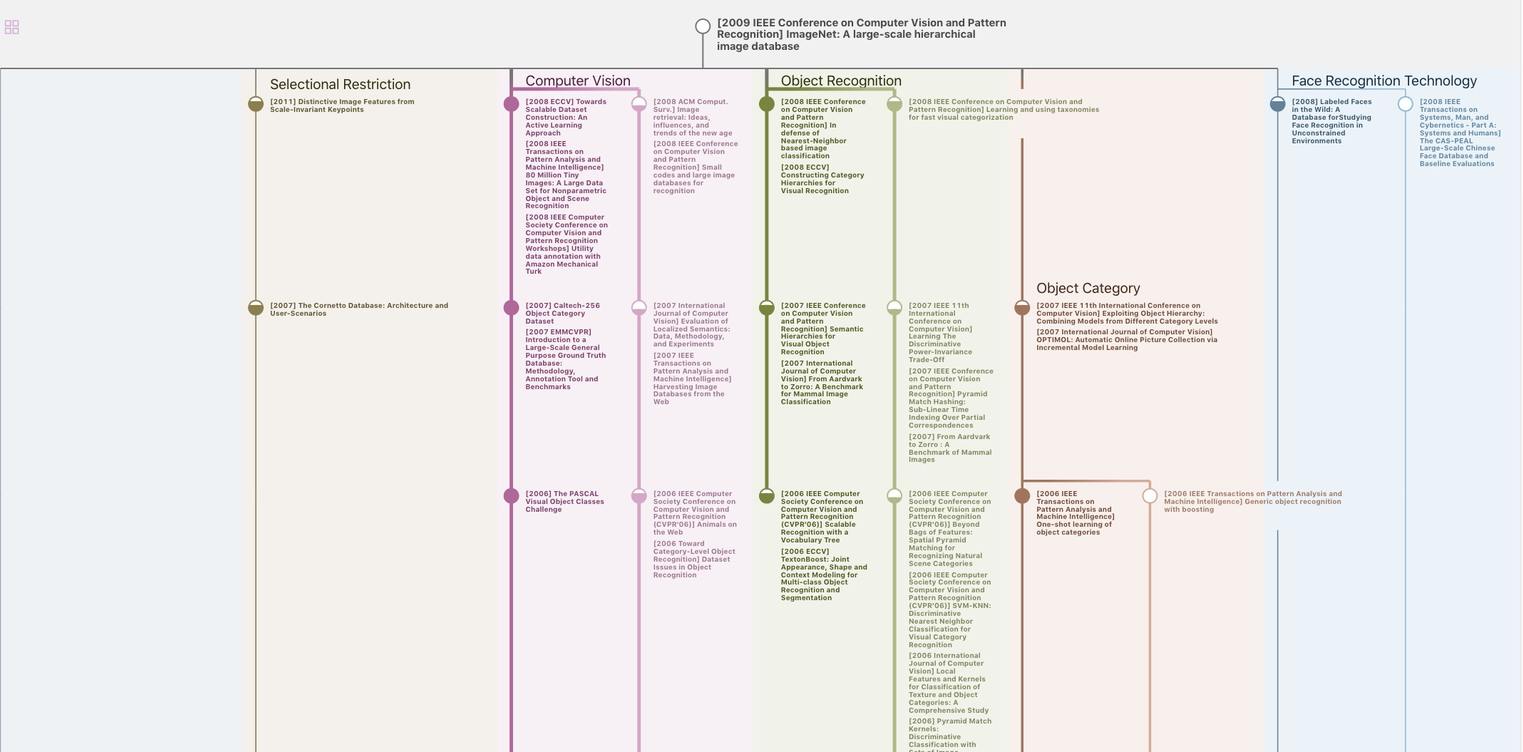
生成溯源树,研究论文发展脉络
Chat Paper
正在生成论文摘要