A Data and Knowledge-Driven Cutting Parameter Adaptive Optimization Method Considering Dynamic Tool Wear
ROBOTICS AND COMPUTER-INTEGRATED MANUFACTURING(2023)
摘要
Tool wear prediction is of significance to reduce energy consumption through cutting parameter optimization. However, the current studies ignore the effect of machine aging on the tool wear prediction model, and their cutting parameter optimization methods cannot cope with the dynamic change of tool wear in the machining process. Thus, a reinforcement learning-enabled integrated method of tool wear prediction and cutting parameter optimization is proposed for minimizing energy consumption and production time. Specifically, the multi-source heterogeneous data fusion-based (MHDF) tool wear prediction model considering machine aging is first proposed to obtain the tool wear of the cutting tool. Then, a Markov Decision Process is designed to model the cutting parameter optimization process, which can be utilized to determine the proper cutting parameters adapted to the dynamic change of tool wear. Finally, the proposed method is demonstrated by extensive comparative experiments, and the results show that: 1) The proposed tool wear prediction model eliminates the influence of machine aging on prediction accuracy and has better generalizability for the machining data under different machine aging conditions, and its testing accuracy reaches 96.09%. 2) The proposed optimization method can adapt to the dynamic change of tool wear and further reduce the energy consumption and production time by 6.72% and 8.60% compared to that of not considering tool wear. The computation time of the proposed method is reduced by an average of 71.80%.
更多查看译文
关键词
Cutting parameters,Energy consumption,Tool wear,Reinforcement learning
AI 理解论文
溯源树
样例
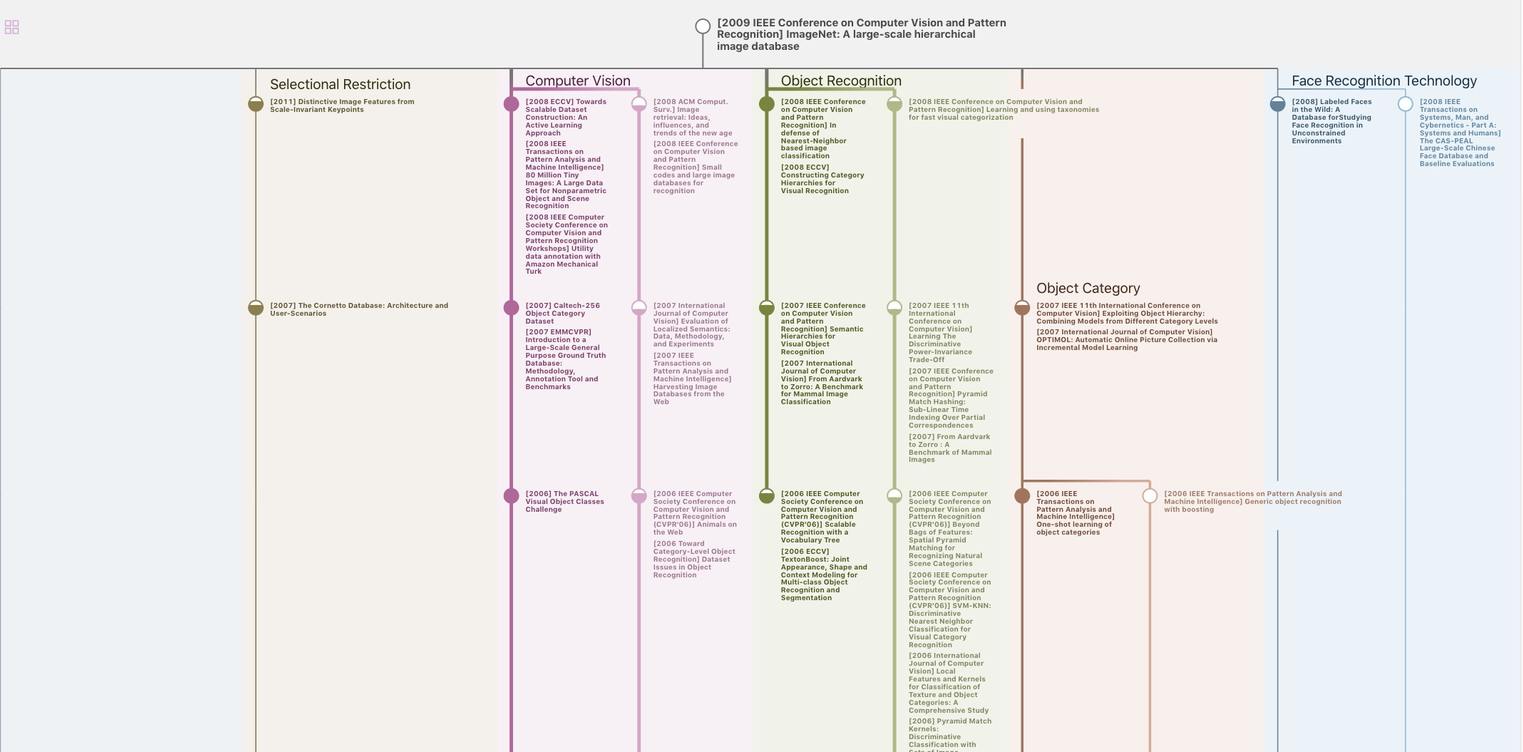
生成溯源树,研究论文发展脉络
Chat Paper
正在生成论文摘要