Spatial heterogeneity automatic detection and estimation
Computational Statistics & Data Analysis(2023)
摘要
Spatial regression is widely used for modeling the relationship between a dependent variable and explanatory covariates. Oftentimes, the linear relationships vary across space, such that some covariates have location-specific effects on the response. One fundamental question is how to detect the systematic variation in the model and identify which locations share common regression coefficients and which do not. Only a correct model structure can assure unbiased estimation of coefficients and valid inferences. A new procedure is proposed, called Spatial Heterogeneity Automatic Detection and Estimation (SHADE), for automatically and simultaneously subgrouping and estimating covariate effects for spatial regression models. The SHADE employs a class of spatially-weighted fusion type penalty on all pairs of observations, with location-specific weight constructed using spatial information, to cluster coefficients into subgroups. Under certain regularity conditions, the SHADE is shown to be able to identify the true model structure with probability approaching one and estimate regression coefficients consistently. An alternating direction method of multiplier algorithm (ADMM) is developed to compute the SHADE. In numerical studies, the empirical performance of the SHADE is demonstrated by using different choices of weights and comparing their accuracy. The results suggest that spatial information can enhance subgroup structure analysis in challenging situations when the spatial variation among regression coefficients is small or the number of repeated measures is small. Finally, the SHADE is applied to find the relationship between a natural resource survey and a land cover data layer to identify spatially interpretable groups. (c) 2022 The Author(s). Published by Elsevier B.V. This is an open access article under the CC BY-NC-ND license (http://creativecommons.org/licenses/by-nc-nd/4.0/).
更多查看译文
关键词
Areal data,Structure selection,Penalization,Repeated measures,Spatial heterogeneity,Subgroup analysis
AI 理解论文
溯源树
样例
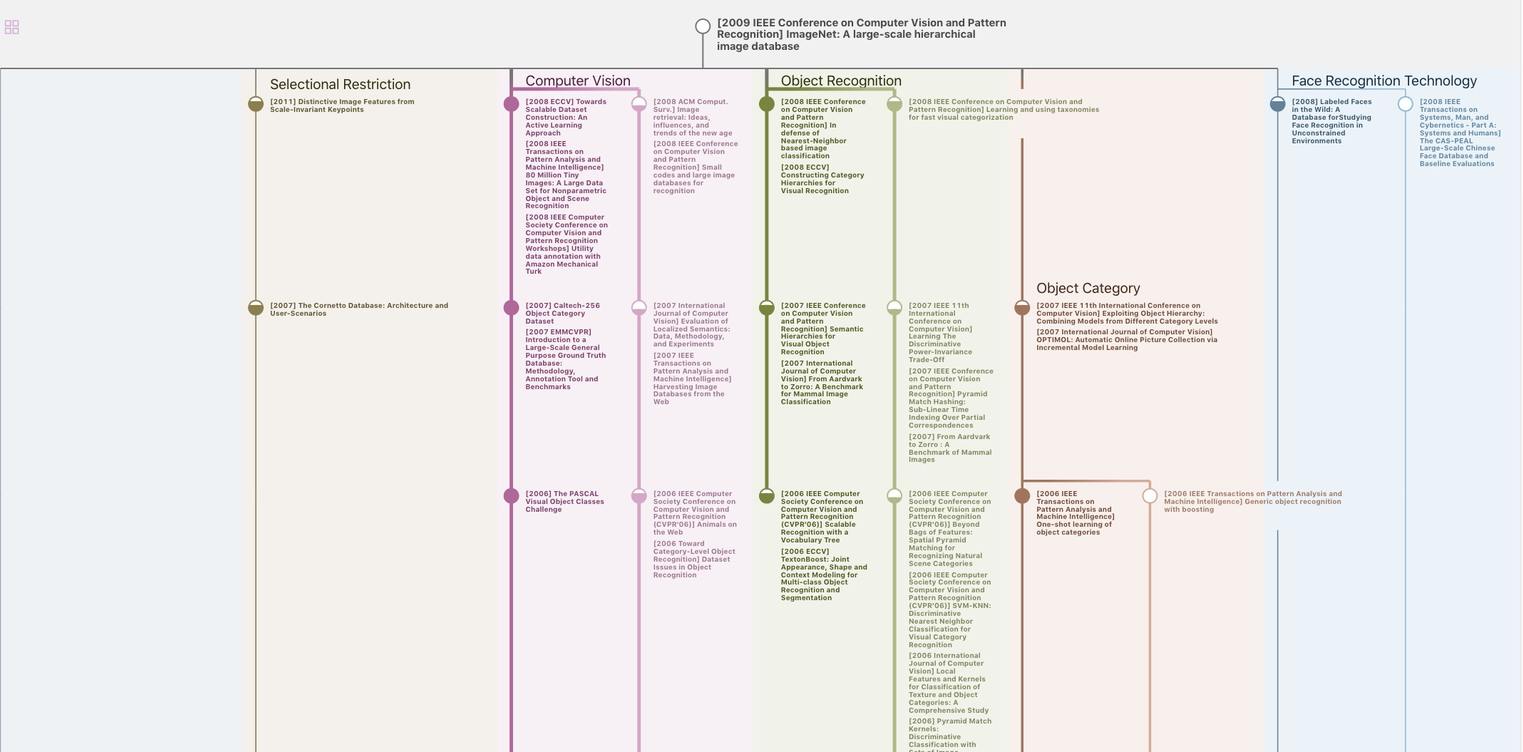
生成溯源树,研究论文发展脉络
Chat Paper
正在生成论文摘要