Robust tests for scatter separability beyond Gaussianity
Computational Statistics & Data Analysis(2023)
摘要
Separability (a Kronecker product) of a scatter matrix is one of favorable structures when multivariate heavy-tailed data are collected in a matrix form, due to its parsimonious representation. However, little attempt has been made to test separability beyond Gaussianity. In this paper, we present nonparametric separability tests that can be applied to a larger class of multivariate distributions not only including elliptical distributions but also generalized elliptical distributions and transelliptical distributions. The proposed test statistic exploits robustness of Tyler's M (or Kendall's tau) estimator and a likelihood function of a scaled variable. Since its distribution is hard to specify, we approximate the p-value using a permutation procedure, whose unbiasedness is obtained from the permutation invariance of multivariate paired data. Our simulation study demonstrates the efficacy of our method against other alternatives, and we apply it to rhesus monkey data and corpus callosum data.
更多查看译文
关键词
Scatter matrix,Kronecker product,Matrix-variate data,Generalized elliptical distribution,Transelliptical distribution
AI 理解论文
溯源树
样例
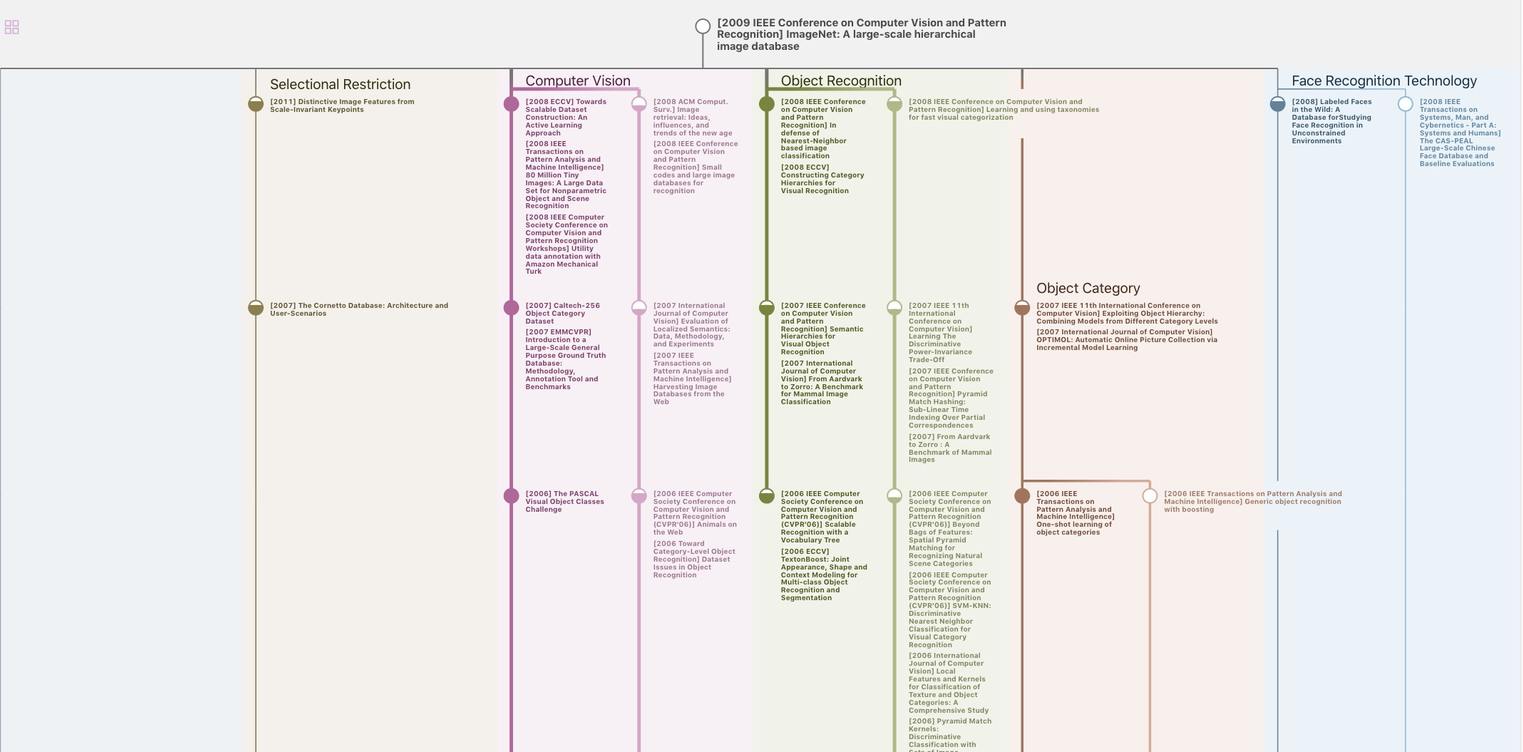
生成溯源树,研究论文发展脉络
Chat Paper
正在生成论文摘要