A Comparative Study of Learning Techniques for the Compressible Aerodynamics over a Transonic RAE2822 Airfoil
COMPUTERS & FLUIDS(2023)
摘要
In this study, the modeling of the compressible pressure field on the RAE 2822 airfoil using deep learning (DL) is investigated. The objective is to generate, at low cost, the complete Mach envelope from a given aerodynamic database. A dataset with more than 2.000 RANS simulations at various angles of attack and Mach numbers has been created, and validated, to train fast and accurate surrogate models model of the pressure field. The procedure is inspired by the well-known analytical Prandtl–Glauert transformation, which maps solutions from one Mach number to another, but however fails in the transonic regime because of the strong non-linearities due to shock waves. Thus, instead of a classical direct generation of the pressure fields, DL is used here to perform a geometric transformation from an incompressible pressure field towards a compressible solution. The key question addressed in this study is: how accurate and sample efficient are DL techniques compared with classical surrogate modeling tools? To answer this question, two types of DL are investigated (CNN and GCNN) and compared with Proper Orthogonal Decomposition (POD) coupled to a Gaussian Process Regression (GPR). These methods are trained on various input resolutions and database sizes. Results show that DL models are able to accurately predict the intensity and location of the shock over a wide range of angles of attack and Mach numbers up to the transonic regime. Predictions of the lift coefficient reveal a relative error lower than 2% with respect to the high-fidelity data. Because of their inherent nonlinear nature, CNN and GCNN provide more accurate results even for small training datasets, whereas POD encounters difficulties to reconstruct properly shock waves. Since CNN requires inputs and outputs in a pixel-like format, it suffers from interpolation errors that can only be mitigated with fine resolutions, making the training more difficult. By performing convolutions directly on unstructured data, GCNN eliminates this interpolation error and provides the best accuracy and sample efficiency. This study shows that advanced DL techniques, such as CNN and GCNN, are capable of predicting complex flows, and outperform classical tools on such tasks.
更多查看译文
关键词
Transonic aerodynamics,Deep learning,CFD,RAE2822
AI 理解论文
溯源树
样例
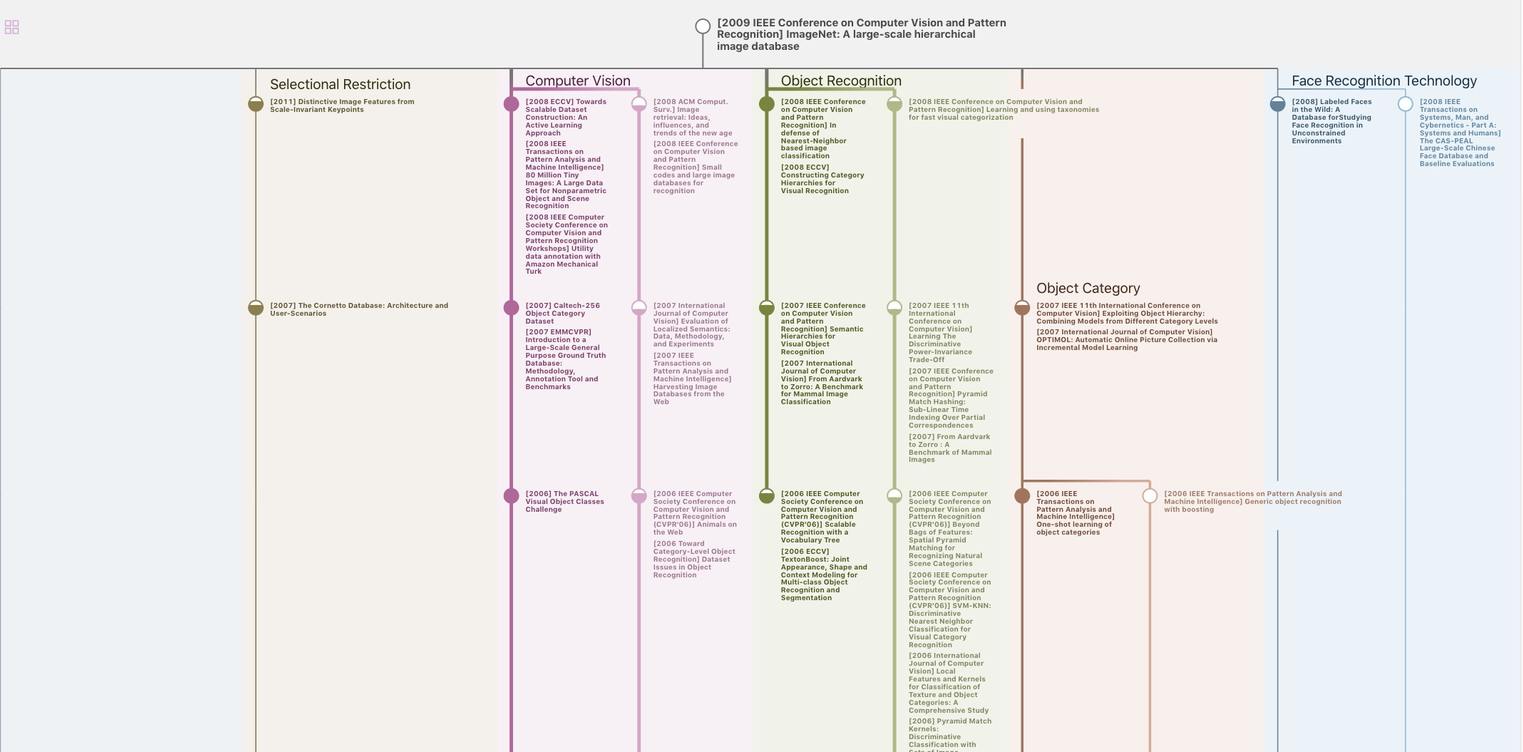
生成溯源树,研究论文发展脉络
Chat Paper
正在生成论文摘要