SALT: A multifeature ensemble learning framework for mapping urban functional zones from VGI data and VHR images
Computers, Environment and Urban Systems(2023)
摘要
Urban functional zone mapping is essential for providing deeper insights into urban morphology and improving urban planning. The emergence of Volunteered Geographic Information (VGI), which provides abundant semantic data, offers a great opportunity to enrich land use information extracted from remote sensing (RS) images. Taking advantage of very-high-resolution (VHR) images and VGI data, this work proposed a SATL multifeature ensemble learning framework for mapping urban functional zones that integrated 65 features from the shapes of building objects, attributes of points of interest (POIs) tags, locations of cellphone users and textures of VHR images. The dimensionality of SALT features was reduced by the autoencoder, and the compressed features were applied to train the ensemble learning model composed of multiple classifiers for optimizing the urban functional zone classification. The effectiveness of the proposed framework was tested in an urbanized region of Nanchang City. The results indicated that the SALT features considering population dynamics and building shapes are comprehensive and feasible for urban functional zone mapping. The autoencoder has been proven efficient for dimension reduction of the original SALT features as it significantly improves the classification of urban functional zones. Moreover, the ensemble learning outperforms other machine learning models in terms of the accuracy and robustness when dealing with multi-classification tasks.
更多查看译文
关键词
Urban functional zone mapping,SALT features,Ensemble learning,Volunteered geographic information
AI 理解论文
溯源树
样例
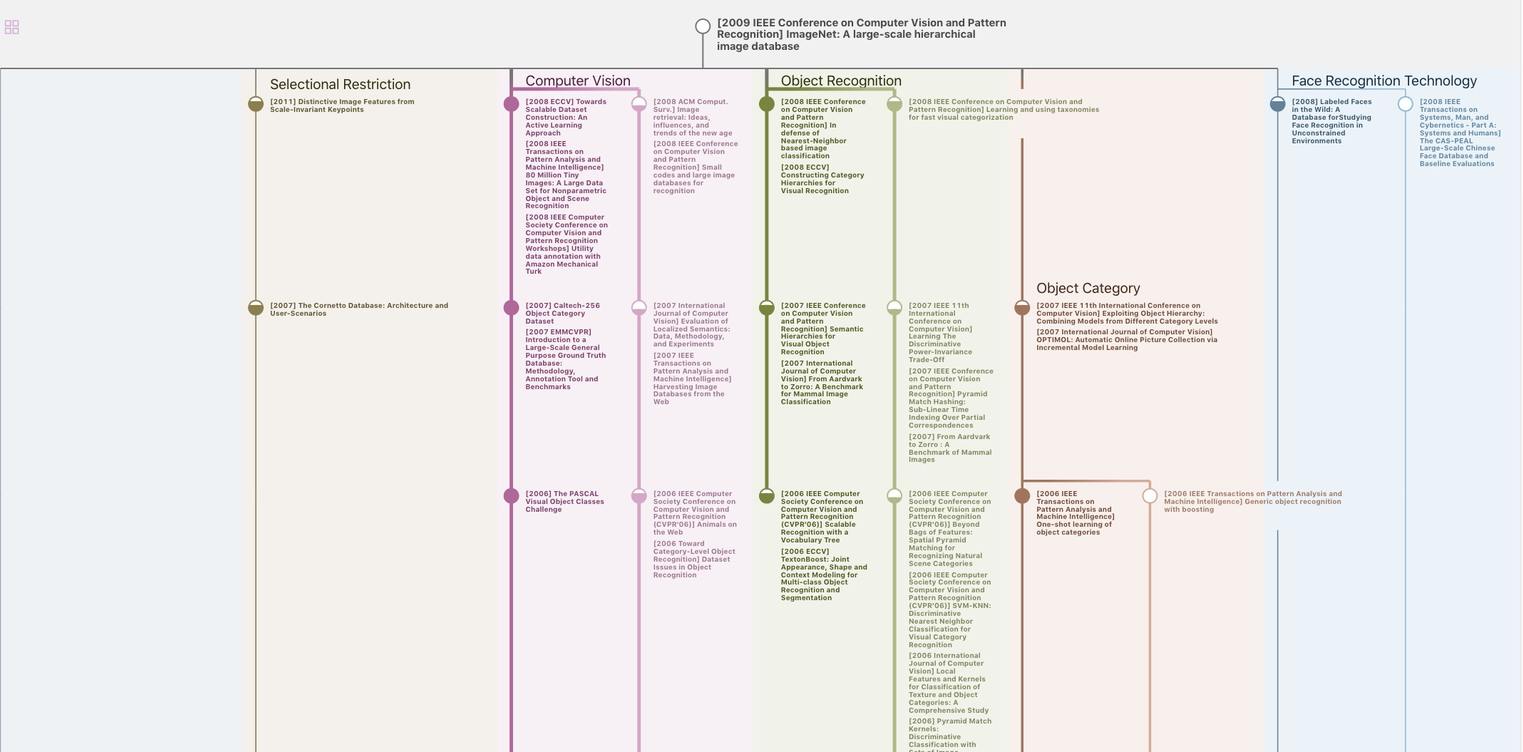
生成溯源树,研究论文发展脉络
Chat Paper
正在生成论文摘要