Raman Spectral Concentration Prediction of Naphthalene Based on Multi-CNN Model
2022 International Conference on Cyber-Physical Social Intelligence (ICCSI)(2022)
摘要
Polycyclic aromatic hydrocarbons (PAHs) may accumulate in edible oil from polluted environments or food, which are carcinogenic and cause serious harm to human health. In this study, thin-layer chromatography (TLC) and surface-enhanced Raman scattering (SERS) was used to detect the inset di-naphthalene Raman spectra in different concentrations from edible oil, and the Raman spectral data in the samples were recorded by Raman spectrometaer. Traditional machine learning algorithms of point to point the need for complex smoothing, baseline deduction of data pretreatment such as work, into will cause the data change after the manual intervention, and influence the results of the analysis accuracy, and machine learning can't deeply extract spectral characteristics, this study will Raman spectroscopy combined with deep learning, One-dimensional convolutional neural network (1D-CNN) model was used to predict the concentration of innervated di-naphthalene. Based on the test data of the four characteristic peaks of the original spectrum, spectral features were extracted by convolution at different scales, and the relationship between spectral features and concentration was learned by multi-layer network structure, to establish a more accurate model. Compared with logistic regression, support vector machine, decision tree, and other algorithms, it is verified that the one-dimensional convolutional neural network model has high concentration prediction accuracy and good generalization, with RMSEC only 0.43.
更多查看译文
关键词
Surface-enhanced Raman scattering,Thin layer chromatography,Binaphthalene spectrum,Machine learning,Convolutional neural network,Predict
AI 理解论文
溯源树
样例
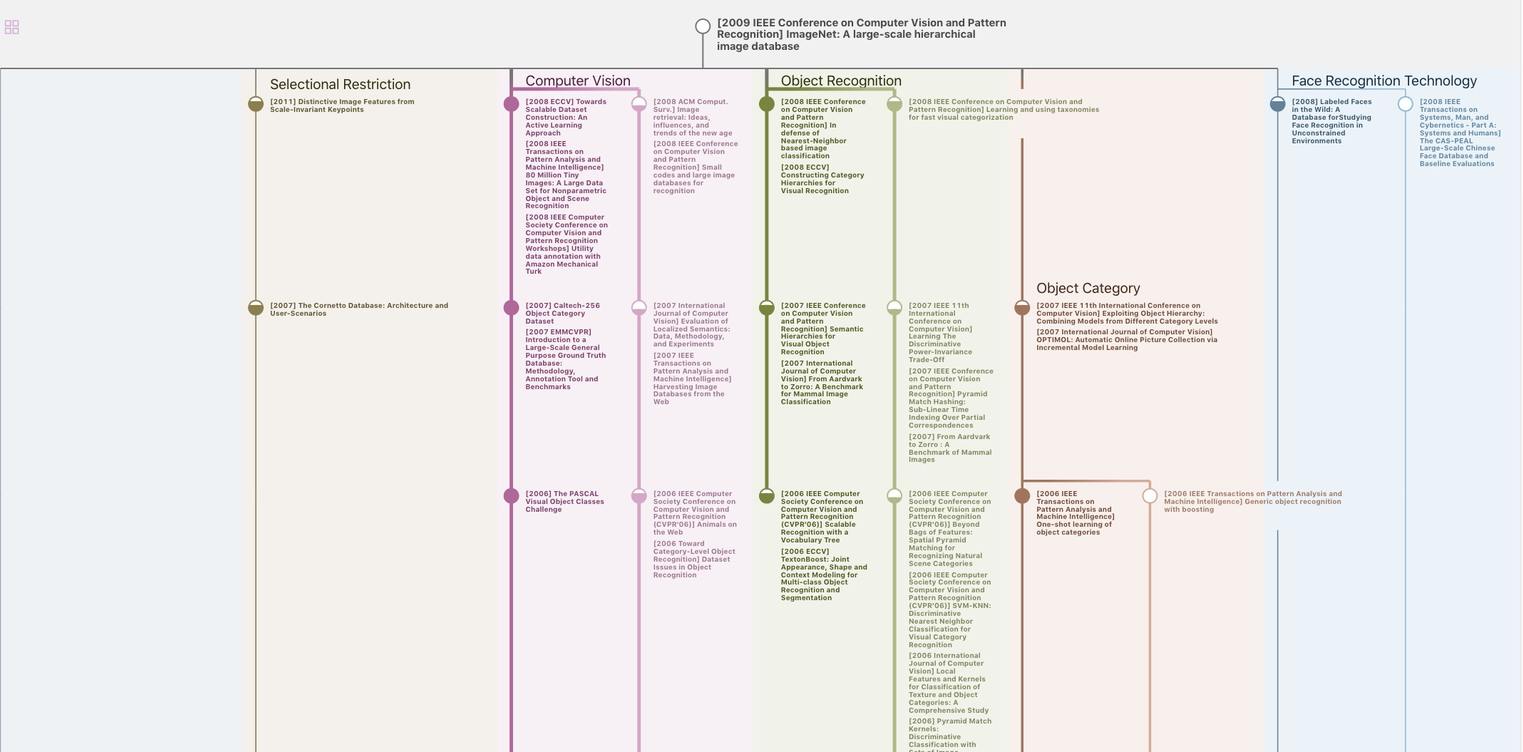
生成溯源树,研究论文发展脉络
Chat Paper
正在生成论文摘要