Optimizing Execution Time and Costs of Cross-Silo Federated Learning Applications with Datasets on different Cloud Providers
2022 IEEE 34th International Symposium on Computer Architecture and High Performance Computing (SBAC-PAD)(2022)
摘要
Under the coordination of a central server, Federate Learning (FL) enables a set of clients to collaboratively train a global machine learning model without exchanging their local data. When such clients have powerful machines, it is called cross-silo FL, and they store their data in private repositories denoted silos. We are interested in this paper in cross-silo FL where silos are geographically located in different regions of multi-cloud providers. Thus, aiming at minimizing financial costs and execution times of a cross-silo FL application, we propose a model based on a scheduling problem mathematical formulation, which receives as input both the application parameters and the cloud providers' resource features where clients' data are stored and renders the best assignment of clients and server to virtual machines. This formulation is part of a framework proposal to execute FL applications in different cloud providers. Taking as a use case a Tumor-Infiltrating Lymphocytes Classification problem, an FL application whose clients' datasets spread over different cloud providers' data repositories, evaluation results show that our model is scalable and improves the execution time and financial costs of the FL application by up to 53.70% and 48.34% in a scenario with 50 clients, executing in around 200 seconds, when compared to results where VMs are randomly selected. Experimental results with client silos in different Google (GCP) and Amazon (AWS) cloud regions also confirmed the effectiveness of our proposed model in a real multi-cloud environment.
更多查看译文
关键词
Scheduling Problem,Federated Learning,MultiCloud
AI 理解论文
溯源树
样例
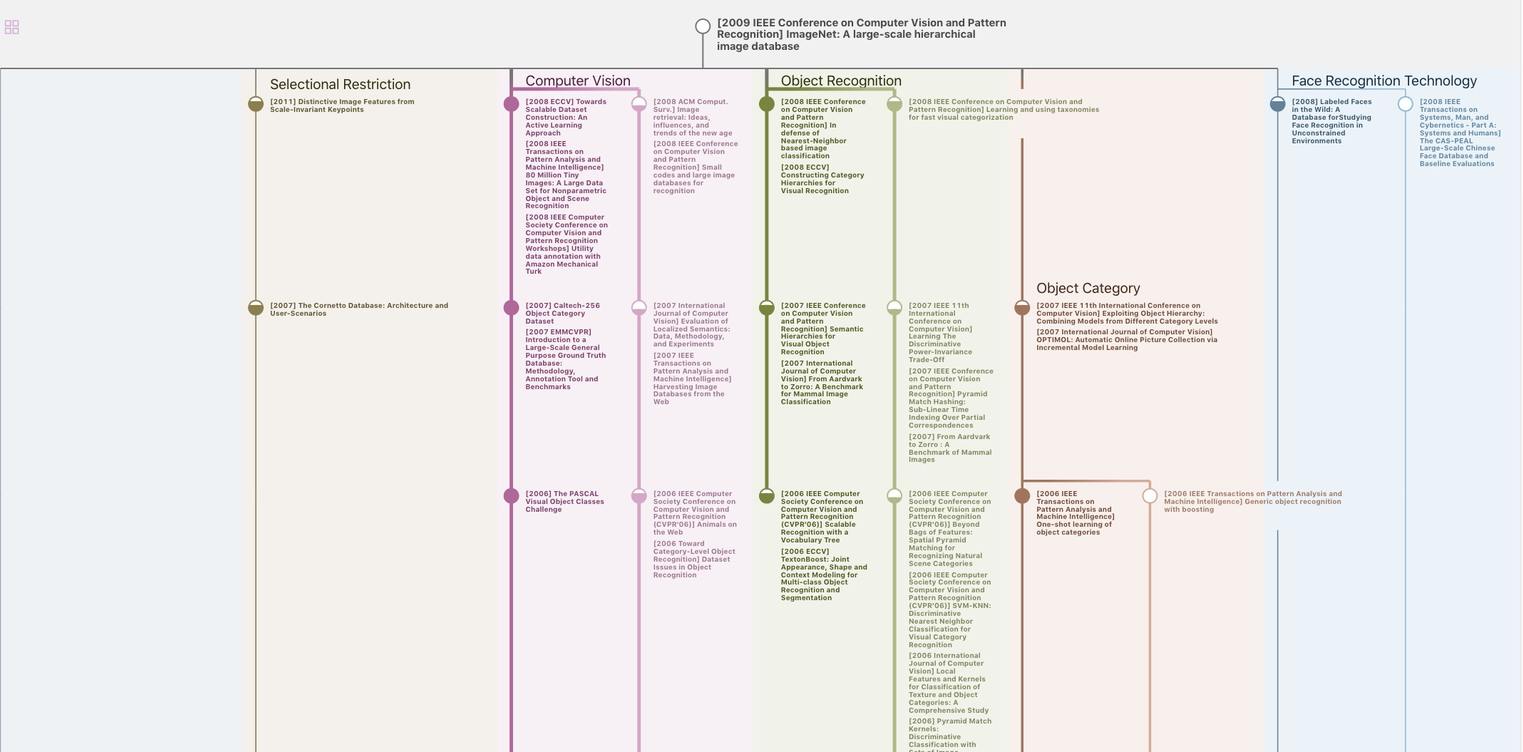
生成溯源树,研究论文发展脉络
Chat Paper
正在生成论文摘要