Neural Fault Analysis for SAT-based ATPG
2022 IEEE International Test Conference (ITC)(2022)
摘要
Continued advances in process technology have led to a relentless increase in the design complexity of integrated circuits (ICs). In order to meet the increasing demand of low defective-parts-per-million (DPPM) and high product quality of the complex circuit designs, Boolean Satisfactory (SAT) has worked as a robust alternative to conventional APTG techniques. In SAT-based ATPG, logic cones related to the target faults are transformed to Boolean formulas, and standard SAT solving procedures are then used for solving these formulas. Recently, artificial intelligence (AI) techniques have shown great potential in speeding-up SAT solvers. However, the high diversity of the structural characteristics within the logic cones of target faults limits the AI techniques being used for SAT-based ATPG. To meet this challenge, this paper proposes a neural fault analysis technology that is made up of a multi-stage learning model and the testability classifier to highly increase the SAT-based ATPG solving efficiency. The multi-stage learning model is composed of a generative model with a topology structure discriminator and a conflict structure discriminator. It is trained for high-quality data synthesis. Then the testability classifier is trained for adaptive heuristic selection and effective initialization in SAT-based ATPG. Experimental results on both open-source and industrial circuits demonstrate that the neural fault analysis can reduce the SAT solving time by 34.79% and reduce the runtime of SAT-based ATPG by 7.43% on average. It is also shown that the proposed neural fault analysis can cover 9.14% of the faults failed by the conventional SAT-based ATPG framework with a comparable runtime.
更多查看译文
关键词
SAT-based ATPG,Fault Analysis,Testability Prediction,Fault Category Prediction,Adaptive Algorithm Selection
AI 理解论文
溯源树
样例
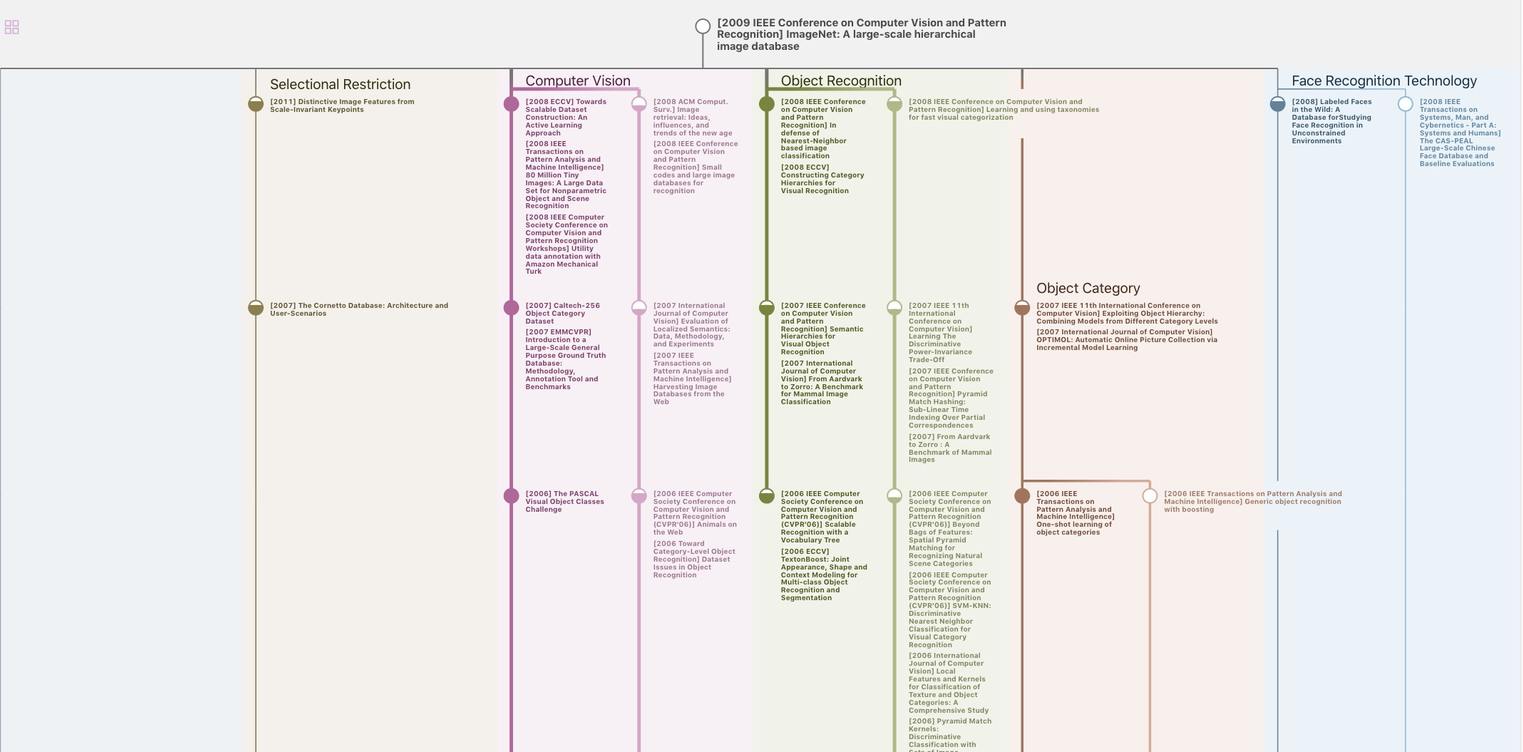
生成溯源树,研究论文发展脉络
Chat Paper
正在生成论文摘要