Learning Low-Complexity Robust Transceiver for Massive MIMO Downlink with Enhanced Mobility
2022 IEEE 33rd Annual International Symposium on Personal, Indoor and Mobile Radio Communications (PIMRC)(2022)
摘要
This paper studies low-complexity robust beamforming in mobile massive multiple-input multiple-output (MIMO) wireless communication systems, which introduces a robustness factor and deep learning (DL)-based framework to mitigate the impact of imperfect channel state information (CSI). By incorporating the estimation uncertainty, we aim to design transceivers to minimize outage probability subject to a total transmit power constraint. However, due to the probabilistic constraints, the optimization problem is difficult to solve. Therefore, we introduce a robustness factor to the quality of service (QoS) constraints by maximizing a zero-outage region, based on an extension of the offset maximization method. Then, we convert the problem into a convex problem and get a quasi-closed-form solution. Besides, iterating the fixed point equation of the Lagrange multipliers in the inequality optimization introduces enormous computational complexity. To this end, we present a novel DL-based algorithm to predict the multipliers directly from imperfect CSI, which includes a column convolutional layer elaborately designed for channel input. Comprehensive experimental comparisons demonstrate the proposed robust transceiver can improve the outage probability significantly over conventional baselines, and the DL-based algorithm can further reduce the running time.
更多查看译文
关键词
massive MIMO,transceiver beamforming,imperfect CSI,deep learning
AI 理解论文
溯源树
样例
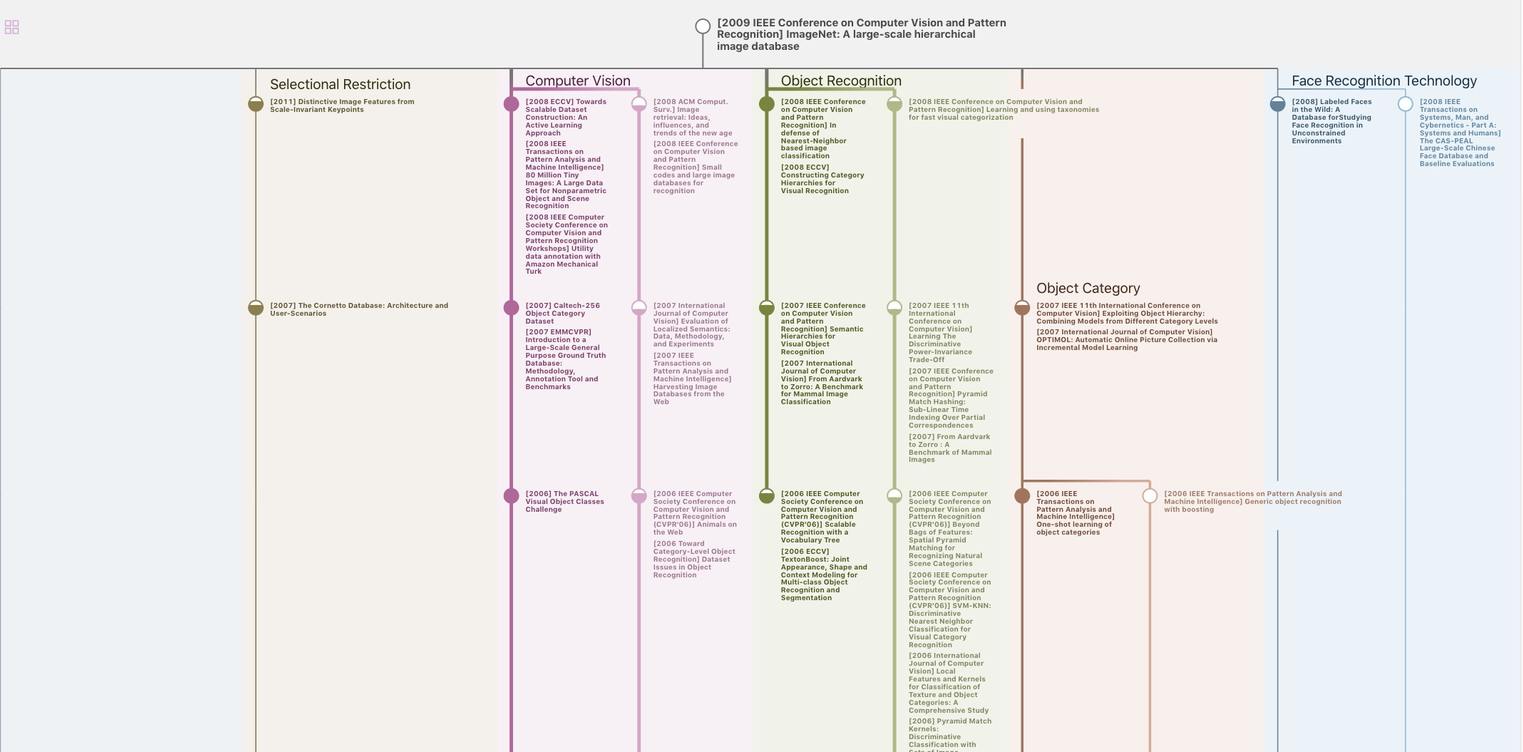
生成溯源树,研究论文发展脉络
Chat Paper
正在生成论文摘要