Continuous Authentication on Unconstrained Activities using Window and Cycle Based Segmentation
2022 Asia-Pacific Signal and Information Processing Association Annual Summit and Conference (APSIPA ASC)(2022)
摘要
Continuous authentication using an accelerometer approach is promising to improve security. Recently, various techniques have been proposed and gained significant performance improvement. However, the existing studies only focused on exploiting the constrained walking activity data, which tended to form a periodic pattern. Furthermore, previous studies did not represent the evaluation of unconstrained activities with “non-periodic” patterns. This paper proposes a continuous authentication method based on window-based and cycle-based segmentation to fit different activity patterns. We evaluated this method using a hybrid Convolution Neural Network (CNN) - Recurrent Neural Network (RNN) based architecture. We assessed this segmentation method using the BBMAS dataset, including walking, door entry, and stair entry activities. The experiments showed that the combination of windows and cycle-based segmentation data performs better for identification and verification than the previous methods. Best EER achieved 5.09% when the walking activity data was used to train and test the feature extraction model. For different activities, EERs achieved between 6 % to 14 %.
更多查看译文
关键词
cycle based segmentation,unconstrained activities
AI 理解论文
溯源树
样例
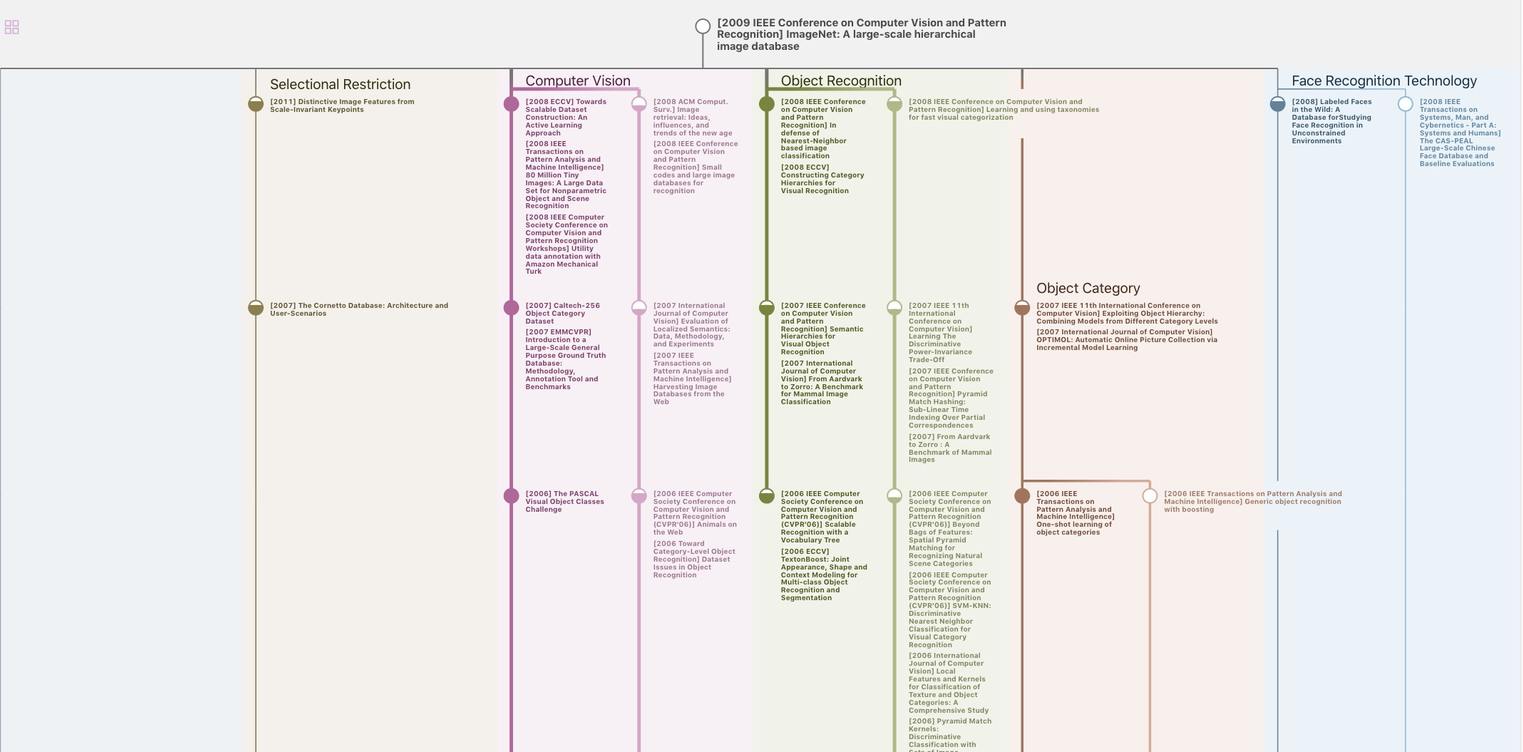
生成溯源树,研究论文发展脉络
Chat Paper
正在生成论文摘要