Cluster Affiliation Model for Local Community Detection
2022 4th International Conference on Data Intelligence and Security (ICDIS)(2022)
摘要
Most community detection studies utilize the entire network to mine all communities. However, in practice, the whole network is often expensive to get or even unavailable. Besides, users may only concern the community containing the node they are interested in. To this end, local community detection emerges. Existing approaches based on Non-negative Matrix Factorization need to set parameters to extract subgraphs around the given node and detect overlapping communities rather than detecting one community for a given node. Therefore, in this paper, a novel parameter-free method, called LCAM (Cluster Affiliation Model for Local community detection), is proposed. It fits the edges in the network to obtain the affiliation of nodes and uses affiliation of nodes to gradually expand outward from the starting node to get the local community, instead of extracting a subgraph from networks before detecting local communities. Four real-world and five synthetic networks are adopted to test LCAM and experimental results demonstrate that LCAM yields better community structure than comparison algorithms.
更多查看译文
关键词
Community detection,Local community detection,Matrix factorization,Cluster affiliation model
AI 理解论文
溯源树
样例
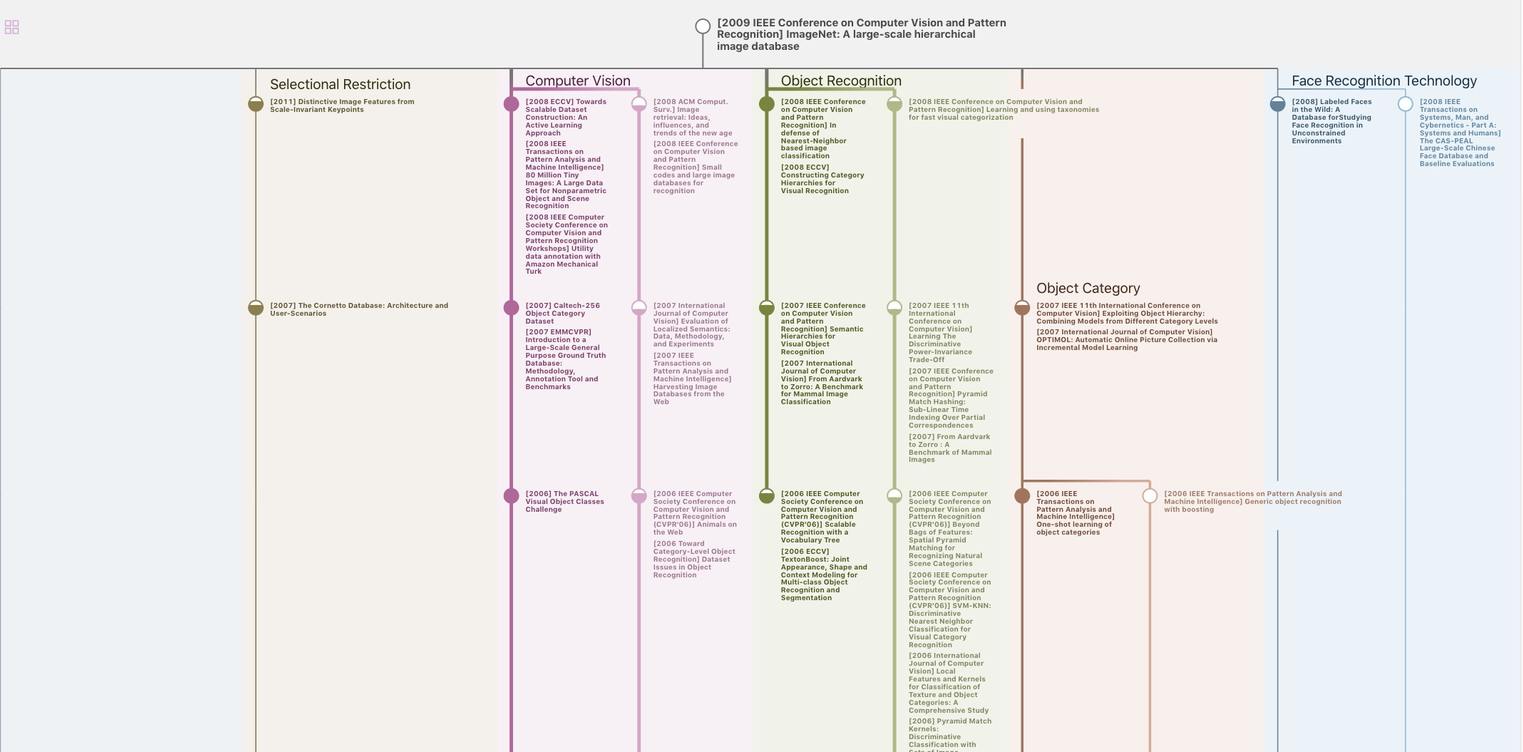
生成溯源树,研究论文发展脉络
Chat Paper
正在生成论文摘要