Instruction-aware Learning-based Timing Error Models through Significance-driven Approximations
2022 IEEE 40th International Conference on Computer Design (ICCD)(2022)
摘要
The adoption of aggressively down-scaled voltages along with worsening process variations, render nanometer devices prone to timing errors that threaten system functionality. The increased vulnerability of nanometer circuits to these errors attracted recent efforts in the development of timing error prediction models using machine learning (ML) methods. However, the majority of such models may be inaccurate, since they either neglect important microarchitecture properties and workload-dependent parameters, affecting timing error manifestation, or are constrained to limited operating areas. In this paper, we propose microarchitecture- and workload-aware ML models for timing error prediction that jointly consider various instruction types as well as all in-flight instructions in a pipeline. Our proposed models are able to predict the exact time and location (i.e., cycle, instruction and bit position) of timing errors with over 98% accuracy across multiple, critical operating regions. To circumvent the increased model complexity due to the considered features, we apply for the first time significance-driven approximations. Evaluation results for various workloads and voltage reduction levels show that our significance-driven precision scaling improves the models’ inference time up to 4.66×, with less than 4% accuracy loss. Finally, we use the proposed model to accurately and realistically inject timing errors during the evaluation of application resiliency. When compared to prior timing error evaluation frameworks that rely on workload-agnostic models, our framework improves the output quality estimation up to 82.6%.
更多查看译文
关键词
machine learning,timing error modelling,approximate computing
AI 理解论文
溯源树
样例
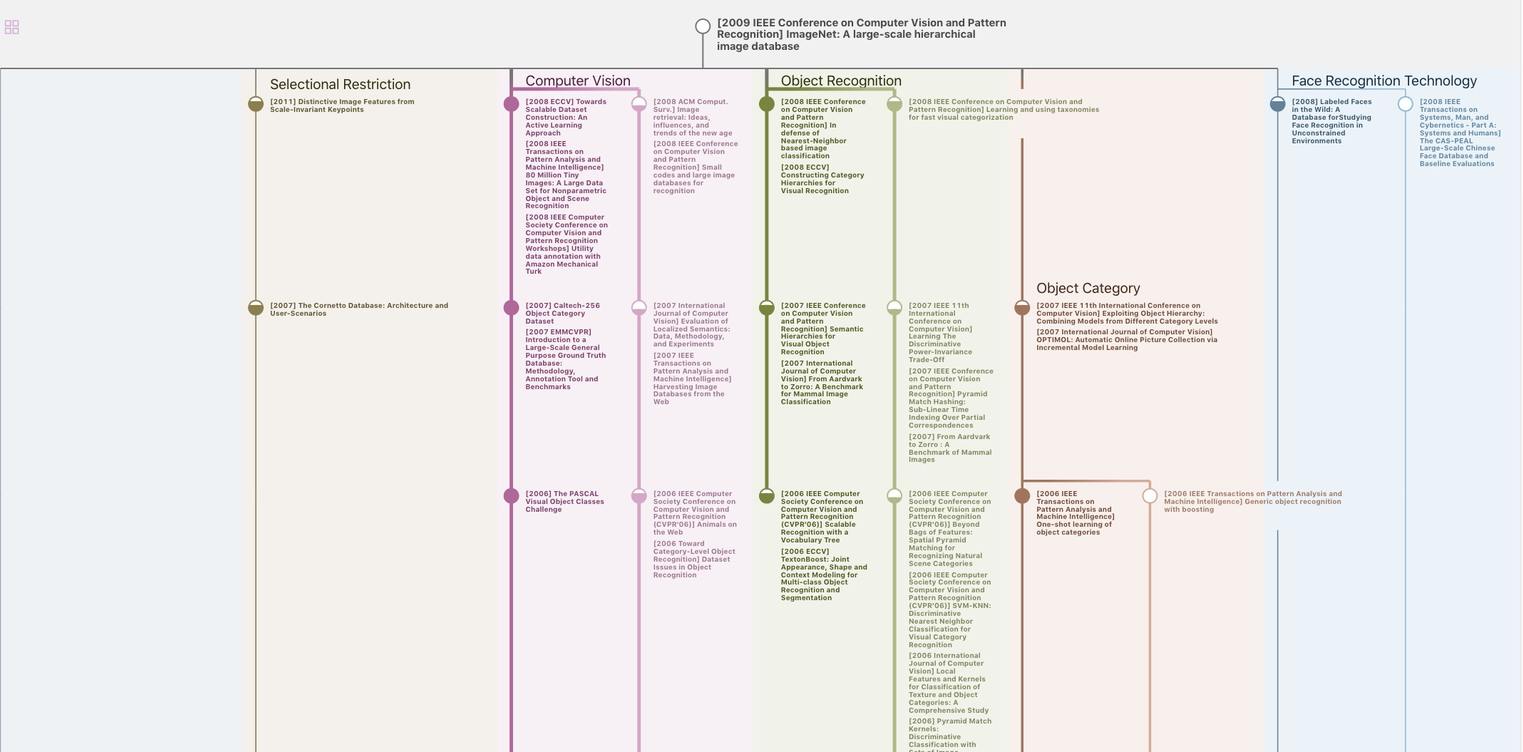
生成溯源树,研究论文发展脉络
Chat Paper
正在生成论文摘要