Self-optimizing multi-domain auxiliary fusion deep complex convolution recurrent network for speech enhancement
Digital Signal Processing(2023)
摘要
A self-optimizing multi-domain auxiliary fusion deep complex convolution recurrent network for speech enhancement (AMDCCRN) was developed to further improve the feature completeness, correlation expression, and global optimization efficiency of the deep speech enhancement model for speech signals. By constructing a multi-domain auxiliary fusion deep complex convolution recurrent network (MDCCRN), the correlation representation of speech features and features in different spatial domains was enriched. The sparrow search algorithm (PGSSA), which is based on a game theory parallel and global optimization strategy, was introduced to improve parallel search capability and to further augment the efficiency and performance of the model's hyperparameter adaptive search. At the same time, PGSSA was used for adaptive optimization of six key model parameters required for AMDCCRN construction. Finally, a speech enhancement model suitable for multi-domain auxiliary fusion with self-learning ability was constructed. Test results on two common speech corpus data sets, THCHS-30 and WSJ0, showed that the proposed method achieved a better speech enhancement effect than other existing methods and that the validity and generalization of the proposed method had been verified. (c) 2022 Elsevier Inc. All rights reserved.
更多查看译文
关键词
Speech enhancement,Multi-spatial domain feature representation,Model efficient search strategy,Model self-optimization
AI 理解论文
溯源树
样例
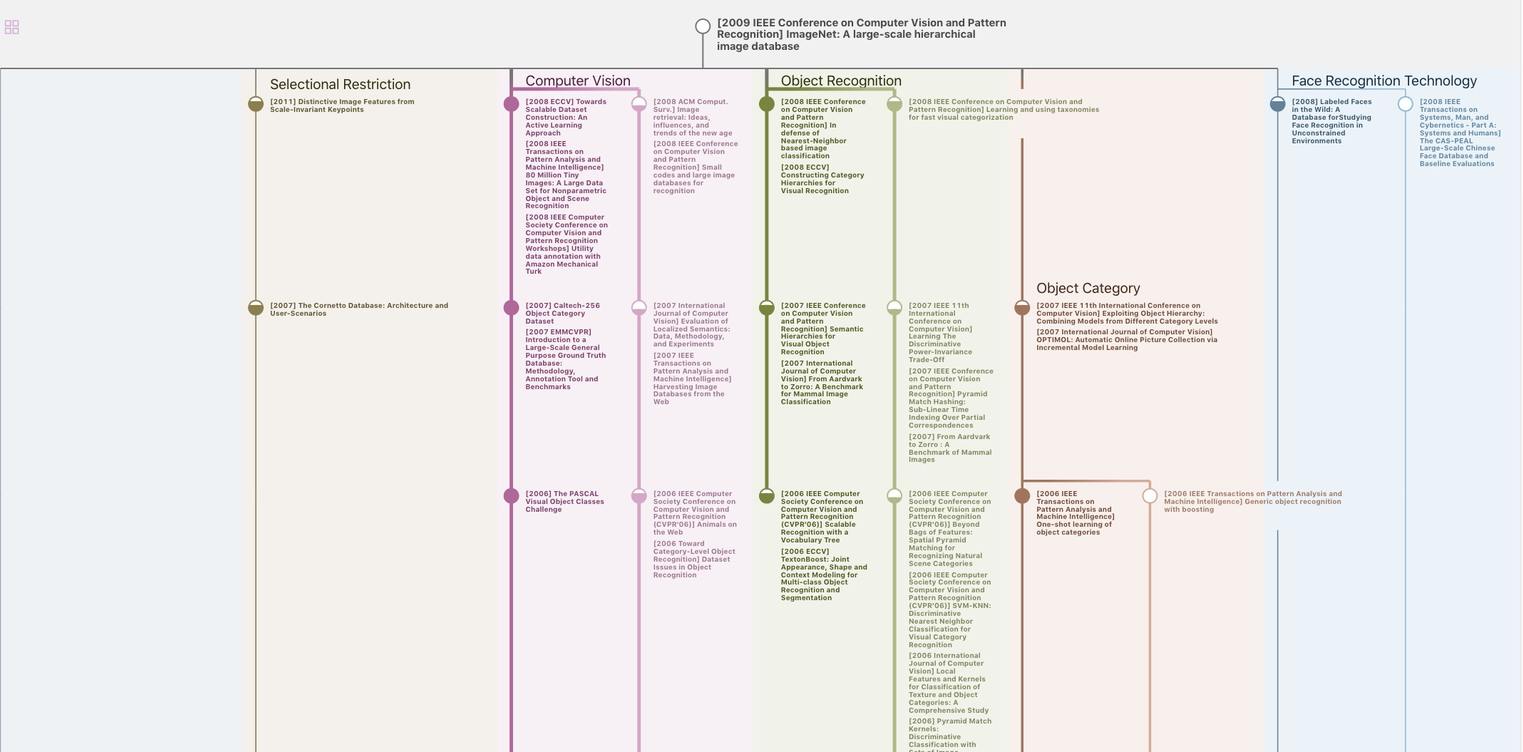
生成溯源树,研究论文发展脉络
Chat Paper
正在生成论文摘要