Class-Aware Pseudo Labeling for Non-random Missing Labels in Semi-supervised Learning.
2022 IEEE Eighth International Conference on Multimedia Big Data (BigMM)(2022)
摘要
Semi-supervised learning (SSL) is a classic missing label problem. Existing SSL algorithms always rely on the basic assumption, label missing completely at random (MCAR), where both labeled and unlabeled data share the same class distribution. Compared to MCAR, the label missing not at random (MNAR) problem is more realistic. In MNAR, the labeled and unlabeled data have different class distributions resulting in biased label imputation, which leads to the performance degradation of SSL models. Existing SSL algorithms can hardly perform well on tail classes (the classes with few training examples) in MNAR setting, since the pseudo-labels learned from unlabeled data tend to be biased toward head classes (the classes with a large number of training examples). To alleviate this issue, we propose a class-aware pseudo-labeling (CAPL) for non-random missing labels in SSL, which utilizes the unlabeled data by dynamically adjusting the threshold for selecting pseudo-labels. Under various MNAR settings, our method achieves up to 15.0% overall accuracy gain upon FixMatch in CIFAR-10 compared with existing baselines.
更多查看译文
关键词
Semi-Supervised Learning,Missing Label Not At Random
AI 理解论文
溯源树
样例
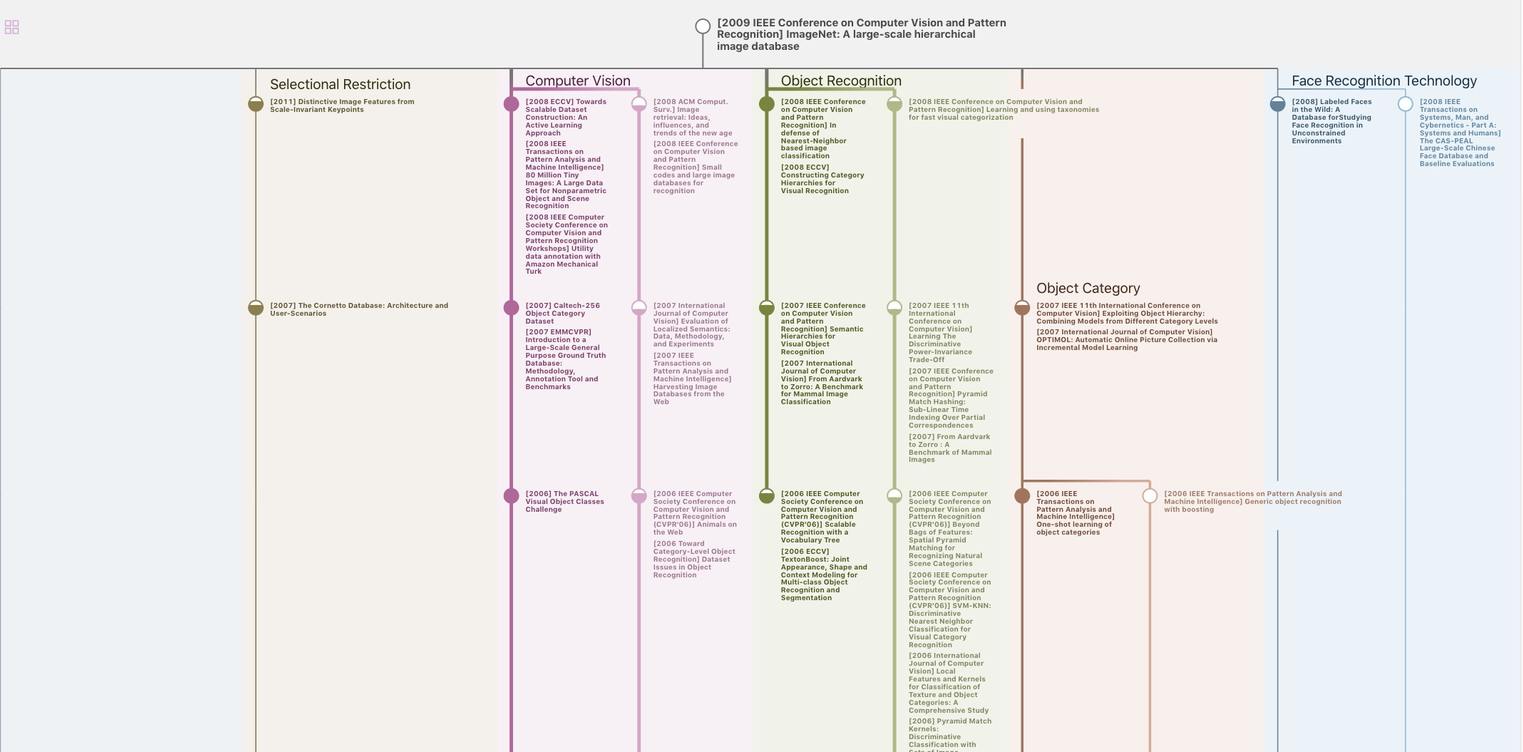
生成溯源树,研究论文发展脉络
Chat Paper
正在生成论文摘要