Prediction of a Road User's Most Likely Future Positions via Simple Kernel Density Estimation
2022 IEEE International Conference on Vehicular Electronics and Safety (ICVES)(2022)
摘要
A method is explored for determining from data a probability density function over future positions of a road user, given that the road user's current state is known. It is assumed that data consists of previously observed road users' state trajectories. The idea is to select from data those states that are most similar, in some sense, to a currently observed road user's state, and from the corresponding future states in the data, compute a probability density function using Kernel Density Estimation(KDE). The resulting method is simple and can quickly be implemented using off-the-shelf implementations of KDE. Qualitative results on real world traffic data show that the method correctly models road user behavior. A qualitative comparison is made with another method also using KDE but based on other assumptions than the those resulting from the aforementioned idea, showing that both methods yield similar results.
更多查看译文
AI 理解论文
溯源树
样例
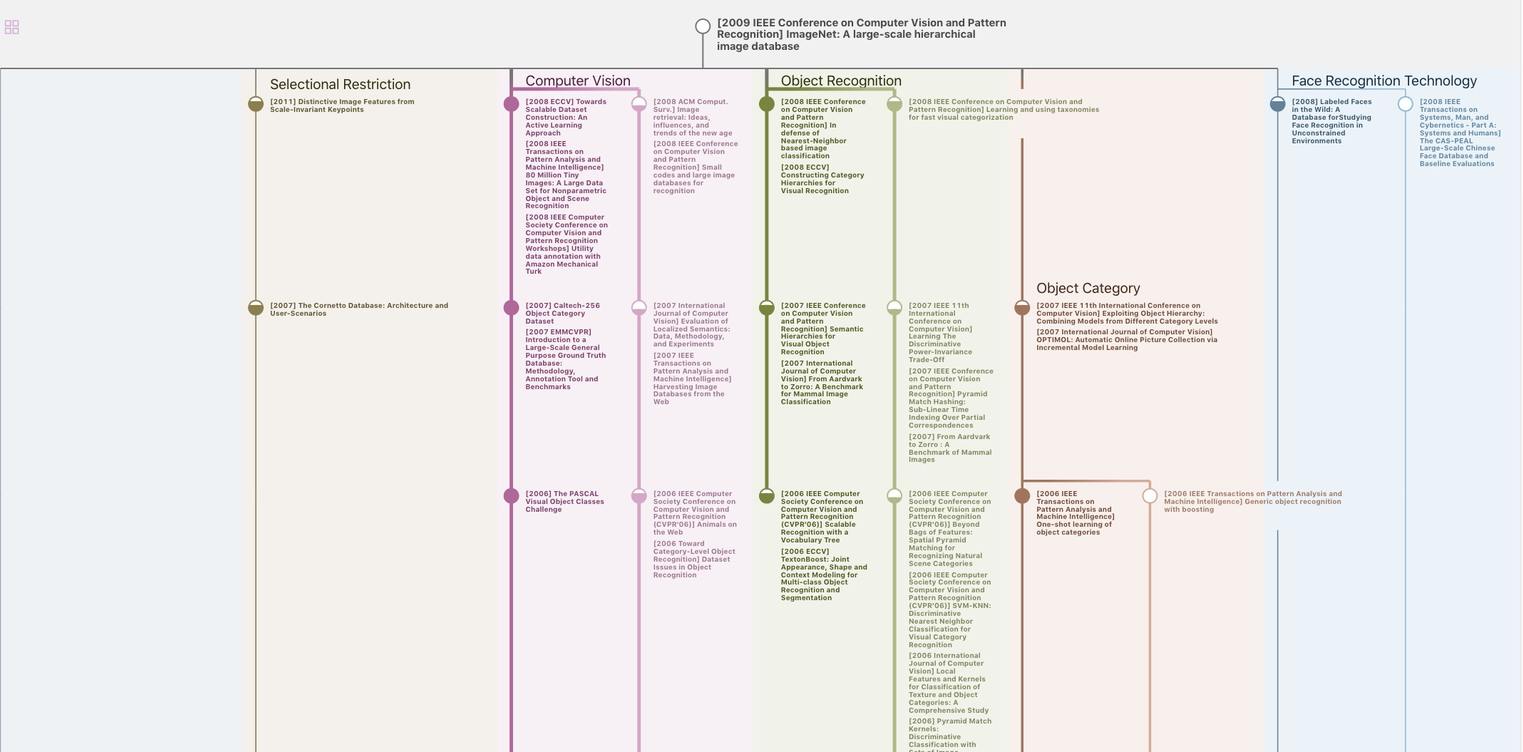
生成溯源树,研究论文发展脉络
Chat Paper
正在生成论文摘要