Effect of variation in the observations on the prediction uncertainty in crop model simulation: Use ORYZA (v3) as a case study
Ecological Modelling(2023)
摘要
The reliability of crop models for predicting ecophysiological processes determines applicability of models in modern agricultural research and production. However, many uncertainties in the modeling processes are strangling the extension of model application. Model parameterization is one of the major sources of prediction uncertainties, which depends on the Observations used for model Calibration (OC). In this study, we conducted a two-year field experiment to evaluate the effect of variation in OC on model prediction error and uncertainty. Model performance was analyzed after the calibration of each dataset. We found that model bias was the dominant factor for the overall prediction error with the low variation of OC. Model uncertainty would contribute more to overall prediction error when OC increased to a threshold of circa 14.5%. The low variation of OC resulted in a lower variation of prediction error (0.04%-26.8%) than that of the high variation of OC (0.4%- 71.8%). The variation of prediction error was different across environments that model simulated. Besides, the prediction error and uncertainty of in-season variables, such as biomass and leaf area index, etc., were also more significant than end-season variables, such as final grain yield. Our findings highlighted that the variability in observations was a major contributor to model prediction uncertainty during the calibration process. We recommend that efforts in uncertainty reduction should include quantitatively assessing in the suitability of datasets for the purpose of calibration, together with making a specific calibration strategy based on the simu-lation targets.
更多查看译文
关键词
Model calibration,Model uncertainty,Prediction error,Experimental data,Rice
AI 理解论文
溯源树
样例
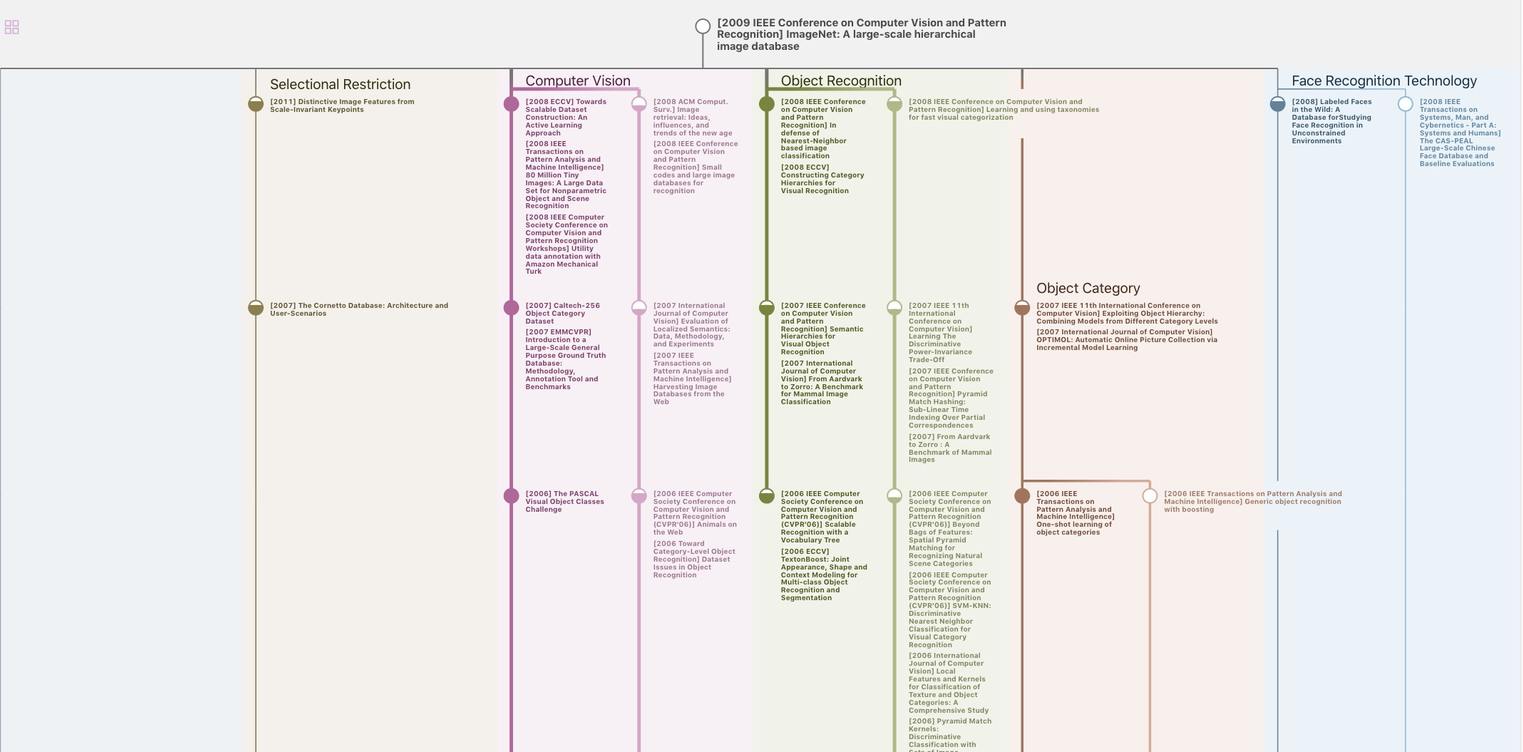
生成溯源树,研究论文发展脉络
Chat Paper
正在生成论文摘要