Bayesian prediction of wildfire event probability using normalized difference vegetation index data from an Australian forest
Ecological Informatics(2023)
摘要
Wildfires are impactful natural disasters, creating a significant impact across many rural communities. Predicting wildfire probability provides authorities with invaluable information to take preventive measures at the early stages. This study establishes Bayesian modelling for predicting the wildfire event probability based on a set of environmental predictors and forest vulnerability, represented by the normalized difference vegetation index. Prior information about the impact of these predictors on the likelihood of wildfire is available in the reports on the past major wildfire events. In that sense, the use of prior information in the Bayesian models has the potential to provide accurate predictions for the wildfire probability. Moreover, the relationship between the predictors creates mediating effects on the likelihood of a wildfire event. A multivariate prior distribution in the Bayesian modelling can capture the mediating effects. In this study, Bayesian models with informative and noninformative priors are considered with independent and multivariate prior distributions to utilize the available prior infor-mation and handle the mediating effects between the predictors using the normalized difference vegetation index data provided by Google Earth Engine. Nine years of data were gathered across 9841 sampled areas in a forested land of Australia. Modelling results concluded that forest vulnerability is found to be the dominant predictor of wildfire probability. This modelling can help create a Wildfire Warning Index based on climate data and forest vulnerability measurements, enabling preventative actions in high-risk and targeted areas.
更多查看译文
关键词
Bayesian model averaging,Bushfires,Forest fires,Multivariate prior,NDVI
AI 理解论文
溯源树
样例
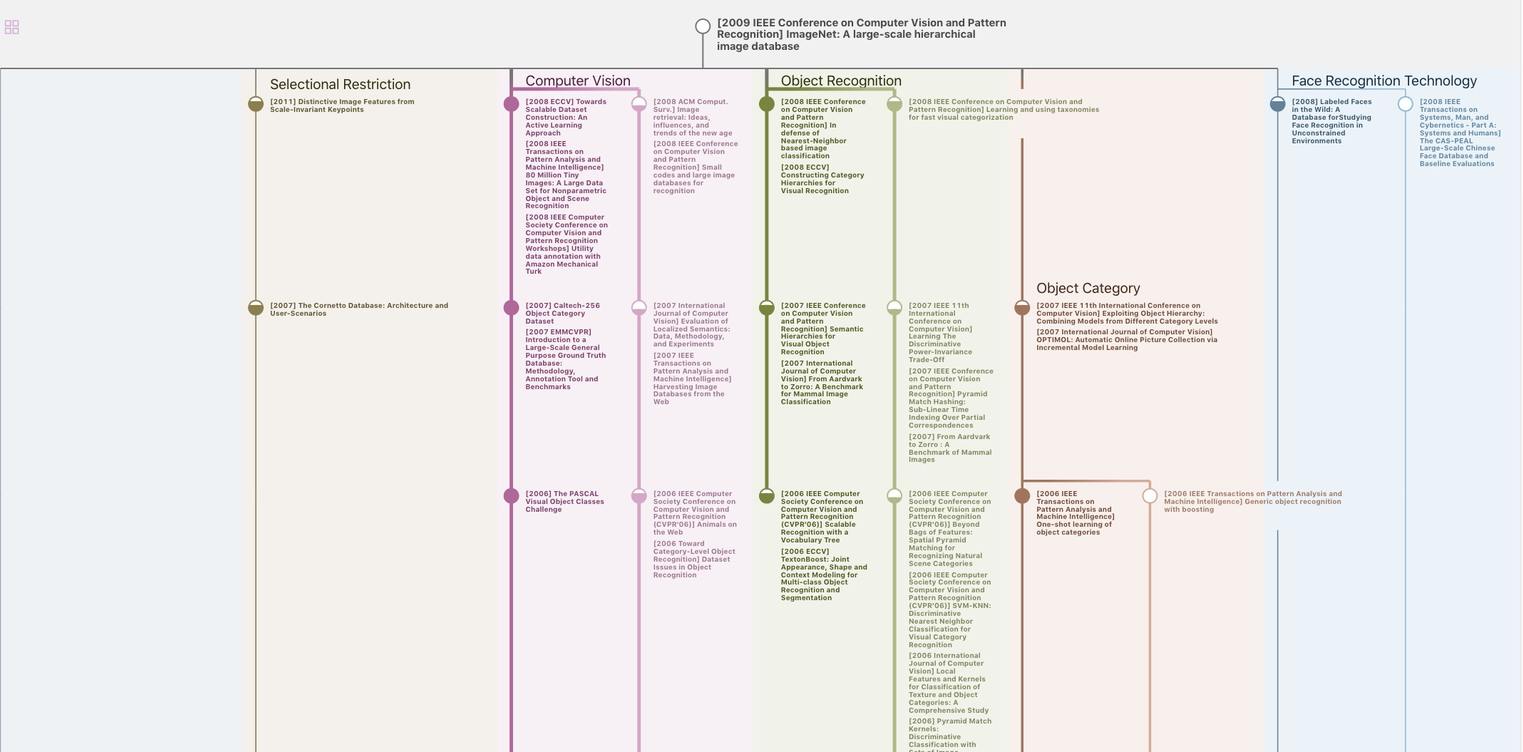
生成溯源树,研究论文发展脉络
Chat Paper
正在生成论文摘要