Predicting The Likelihood of Patients Developing Sepsis Based on Compound Ensemble Learning
2022 IEEE International Conference on Bioinformatics and Biomedicine (BIBM)(2022)
摘要
Objective: Based on a dataset with few features predicts a patient’s likelihood of developing sepsis in the future. Method: We propose a compound ensemble learning framework. At the first stage, UMAP and XgBoost are explored for dimensionality reduction, meanwhile, weak classifiers such as KELM, 1D Convolutional Neural Networks, and XgBoost are employed as base learners to learn patient characteristics from four different emphases. The output of base learners is processed using the proposed Res-Stacking and modified Bagging-like methods in the first stage of compound ensemble learning framework to reduce the error rate. In the secondary ensemble learning stage, the D-S evidence theory is employed to fuse the results of the above two methods and BiLSTM, thereby diminishing the uncertainty of the final output and obtaining more prominent prediction result. Result: The performance of our proposed compound ensemble framework on the clinical dataset of the First Affiliated Hospital of Dalian Medical University in terms of sensitivity, specificity, diagnostic efficiency, PPV, NPV, F1 score and AUC are 0.875, 0.95, 0.928, 0.95, 0.875, 0.95, 0.9125, respectively, better than the single ensemble learning method.
更多查看译文
关键词
Sepsis,Ensemble Learning,D-S Evidence Theory,Artificial Neural Networks,BiLSTM
AI 理解论文
溯源树
样例
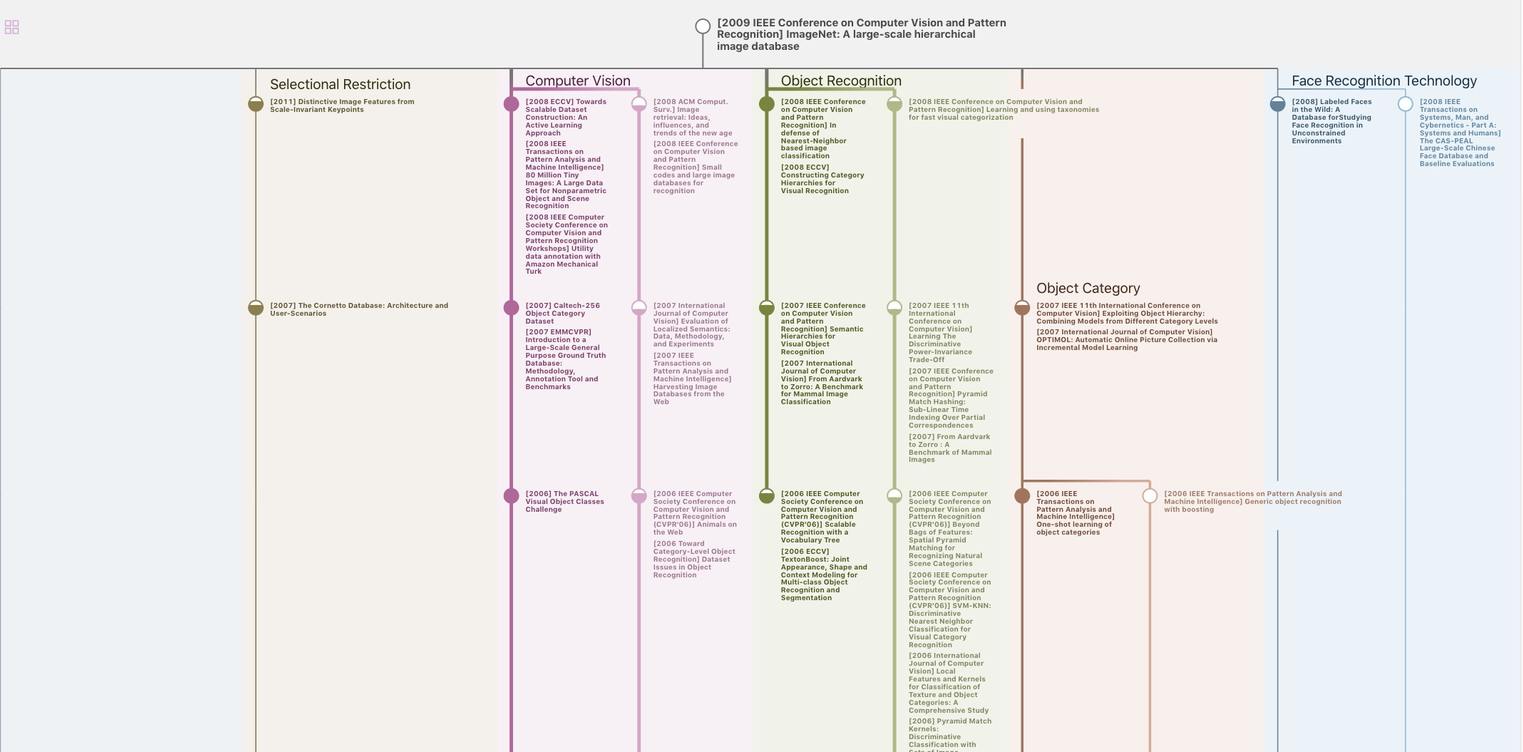
生成溯源树,研究论文发展脉络
Chat Paper
正在生成论文摘要